#87 A/B testing controversies and generative AI (with Shaun McGirr @ Dataiku)
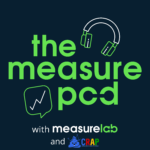
In this week’s episode of The Measure Pod, we dive into a fascinating conversation with Shaun McGirr, a data expert at Dataiku and former Crap Talks presenter. We explore the world of A/B testing, experimentation, and the future of analysts’ roles as generative AI becomes more prevalent. Shaun shares his thoughts on the controversy surrounding A/B testing and the importance of considering other methods of learning about the world. We discuss the challenges and potential of using generative AI in the data analytics space, and how it can revolutionize the way we work with data. Grab your headphones and stap in for an insightful and thought-provoking discussion on the evolving landscape of data science and experimentation!
Show note links:
- Connect with Shaun on LinkedIn.
- Check out what Dataiku are all about.
- Watch Shaun’s popular CRAP Talk on data science vs data religion.
Follow Measurelab on LinkedIn and on Twitter/X, and join the CRAP Talks Slack community.
Find out when the next CRAP Talks event is happening on LinkedIn.
Music composed by Confidential – check out their lo-fi beats on Spotify.
Master Google Analytics 4 with Daniel Perry-Reed on the next GA4 Immersion 6-week cohort training course. Early bird, charity and group discounts availible!
Quotes of the Episode:
- “We very commonly hear, oh, I can’t do anything interesting with data or AI or predicting anything until I’ve got all my data perfect. It’s a myth subscribed to by people who have never actually worked with data. Because if you have, you know it’s never perfect and you still have to make a decision happen.” – Shaun
- “What if you provided an interface that helped people ask the right questions or at least better questions? And if they had no questions, they could be suggested questions and they could pick the questions that they liked the most, and then the job of data people to make LLMs useful is to do prompt engineering, right? To take the small human questions right? Put more context around them.” – Shaun
- “It still requires human curiosity.” – Shaun
Let us know what you think and fill out the Feedback Form, or email podcast@measurelab.co.uk to drop Dan, Dara and Bhav a message directly.
Transcript
The full transcript is below, or you can view it in a Google Doc.
Intro
[00:00:15] Daniel: Welcome back to The Measure Pod. In this episode we’ve got Shaun on as a guest, and this is another ex CRAP Talks presenter who had an amazing conversation at one of the CRAP Talks and managed to antagonise or be slightly controversial in terms of the presentation, which we’ll go into in a bit of detail later on. But I found this conversation absolutely fantastic. A rollercoaster of A/B testing, experimentation, generative AI, the kind of future, certainty of analysts roles as generative AI picks up and everything in between. Be sure to stick to the end to hear Shaun’s rapid fire answers in terms of like what’s going to be the next couple of challenges because I’m sure, as I did, found it very fascinating. But thanks again Bhav for recommending Shaun and bringing him on. I think this is a fascinating conversation and very much out of my depth in terms of subject matter expertise, he knows an awful lot.
[00:01:03] Bhav: I think it was, it was a great discussion. Speaking to Shaun in these type of situations, I always find the conversations are, they never go down the route they’re supposed to go down. It always ends up down some philosophical path, going down some technical elements and I think one of the good things about Shaun is that he’s great at distilling technical information into very easy to understand concepts and he brings his own flavour and opinions into it as well, which keep it really exciting and interesting. So yeah you know, Shaun and I, we met about five years ago. I actually went to someone else’s event and he was one of the speakers. I used to do this a lot back then, I’d go to other people’s events just to recruit speakers and Shaun was one of them. And I think you know, just, we stayed in touch ever since. So it was, yeah, it was great to have him back.
[00:01:42] Dara: And we’re going to share that talk in the show notes, and I’m really looking forward to watching that actually because I missed that CRAP talks. But this chat with Shaun, my head is spinning in a really good way from that, it’s such a good conversation. As you say, Bhav it, you know, we start out in one direction, but it very quickly takes its own, its own form and it’s a really, really good conversation. Enjoy the episode.
[00:02:04] Bhav: Hi everyone, today we’re welcoming Shaun to this episode. Shaun and I have a bit of a history. Shaun is a another ex CRAP talk speaker. He came to an event we hosted at hotels.com. He was one of the last speakers and a fun fact about this event that we ran is that Shaun never actually sent me his slides ahead of time because he told me he’s editing in real time as the other speakers were still speaking and I won’t lie that made me a bit nervous. I had nothing to be nervous about as it turns out, what Shaun was actually doing was taking the content of the previous speakers’ topics and putting him into his own talk so that he could rip them apart and tear the previous speakers an absolute new one. So welcome to the show, Shaun. It’s really great to have you and it’s nice to be speaking again, it’s been a while.
[00:02:48] Shaun: Yeah, that was more than five years ago last time I checked, I do sometimes look at that video, or at least the start of it, and it’s an interesting presentation that you made of what I was doing. Probably I was trying to fill in the last few ideas, I don’t really have anything as a public speaker, like a hundred percent ready when I go on stage. It’s important for me at least to have a little bit of wiggle room, but I do remember, yeah, saying some things about experimentation programs where the sponsors and your stakeholders were in the front row in front of me and I made them a little bit squeamish, but so people came up to me afterwards. And said, thanks for coming in and saying those things, not enough people say those things. Whatever those things were, you can watch the video later.
[00:03:30] Bhav: Before we kick off, do you want to do a quick intro and introduce the world to who you are, what you do.
Topic
[00:03:34] Shaun: Sure so I usually say I’m Shaun, I am a data person and I’ve been doing something with data for about 20 years. So right after high school, before I went to university, I had a kind of a, a summer holiday job for a couple of weeks where I had my first data janitor tasks is what I called them, kind of given two lists of numbers, what numbers are in list A and not on list B. This was printed out, people printed things out 20 years ago and that was long before I knew that this could be done with a join or a merge or a match or anything like that. And I just dutifully went through following instructions and things sort of snowballed from there. So that was with a government agency in New Zealand that runs the census. Obviously they create data, very expensive and high quality data. And then like for maybe the first five, six years, what I studied at university was not related to that ongoing holiday job.
[00:04:29] Shaun: They were just different things I did. I was interested in politics and history, and so I studied those things at university and then, during university holidays, I worked with data and started to dabble in SQL and SaaS and Excel and VBA and all those, you know, a pretty classic kind of slippery slope into automating things story. And then they, those two things came together when I went and did a PhD, or started a PhD at least in Political Science at The University of Michigan in 2008. I did finish that eventually, not without some trials and tribulations as most PhD students go through. And through that process, I worked with some, yeah, really messy, found data and taught myself R and yeah, emerged from that quagmire with enough skills, right as that data science term was becoming fashionable.
[00:05:16] Shaun: So I remember the first time we met Bhav which is what led to you inviting me to CRAP Talks you tried to, we were walking back to the tube and you were trying to convince me that you weren’t a data scientist and you never could be. And I was like, but we basically have like identical backgrounds. You just went into this thing called conversion rate optimization and I went into this other thing and I think actually, in the data world, most conflict is kind of people just using different words to say the same thing or same words to say different things. A huge amount of it’s path dependent on like where you learned a thing and, oh no, I put that line first in my script and you put it second so you’re crazy. And that’s why one of the reasons why the title of that talk at CRAP Talks was data religion versus data science.
[00:05:57] Daniel: I’m really keen to revisit that. So I was at that event, I believe but it was a long time ago, as you said, and for everyone listening that that wasn’t at that talk I’ll link to it in the show notes for anyone that wants to give it a go, but what was the controversy? What was the big kind of moment that you talked about just now in terms of realising you had a lot of these sponsors and testing people in the front row? What made it so controversial?
[00:06:17] Shaun: Just because I was a sort of outsider to that particular flavour of data people that I just said some things that I believed were true and probably still believed that are true, that aren’t controversial to me. Like think of all the things you could want to learn about the world and know about the world right? Call that a hundred percent of all the things. What is the proportion of things in any business organisation that we think we can learn from A/B testing, from running experiments, and that’s driven completely by my background in social science, where experiments are expensive, right? In a way that they might not be in an online platform, but what that resource scarcity around that gold standard evidence drives when, at least when science is done well. And yes, a lot of science is done poorly. A lot of social science is done, is done poorly. What it means is you use experiments to adjudicate between debates.
[00:07:05] Shaun: So when there are two explanations, two or more explanations, but you need to whittle it down to two, ideally through other methods. Two explanations for some phenomenon. So in my world, corruption or voter turnout, or how people choose to vote, or how governments spend their money or that kind of stuff, experiments are kind of the cherry on top that help resolve things at the end, and so that was for me, coming into a community of people who seemed, at least to me, to be talking about this stuff as the starting point for an investigation. It was a bit of a mindbender, so I just wanted to offer that perspective of like, guys, there’s dozens of other ways you can learn things about the world, and there are just trade-offs in how you do that and what you learn.
[00:07:40] Bhav: For what it’s worth I’ve always agreed with you on that one, Shaun. I actually made a note of what it was because your talk was one of my favourite all time talks at CRAP. It’s actually the second most watched video of all my CRAP Talks anyway. And I think it came down to three things that were controversial. The term hypothesis had been thrown around in the previous talks quite a lot. The second thing was they were annualising revenue that was generated as part of the uplifts. And the final part was ignoring multicausal effects and narrowing it down to just one effect. So I think those were the three bits that really got you riled up.
[00:08:17] Bhav: And there was a lot, I mean, you did, for what it’s worth you did give a lot of love and actual acknowledgement of the fact that, you know, the community is made up of people who want to learn and they’re doing it by asking these questions. It’s just, I think some of the approaches were what really, they weren’t what you were used to given your background. I think your view was that the people who work in experimentation CRO, land a hypothesis just on a whim without ever actually considering human behaviour.
[00:08:42] Shaun: Yeah which I know is totally unfair, but like my hypothesis going into the prep for the talk was like, maybe I should say that, maybe I delete it. But then a few previous speakers were talking about, well here we have a hypothesis that people will click a red button more or less or something. And then that combined with analysing the effects of these one-off treatments, right? So like, you know, an experiment is a valid measure of something. And then you, you absolutely can’t multiply that by 12 or 365 to add up some aggregate effect because yeah, an experiment, the reason it’s valuable for inference, for causal inference is that it has this extremely restricted time window right. And I think it’s an impulse, which is a word one of my professors used to make this point.
[00:09:26] Shaun: Like it’s an impulse shock to a system. And the way that shock is measured in the moment tells you nothing about how it plays out over time. And to understand how that plays out over time, it would be really good to have a theory of user behaviour and a theory of the economics of your organisation. So that you could value what you’re doing in a less naive way. And of course people have theories of user behaviour but in the discussions after my talk it emerged to me, perhaps unsurprisingly, so no one in the room was responsible for the model of user behaviour, that was a different team that aren’t in this particular meetup group and then they do something and then generate hypotheses and we test those things and someone else then does the accounting right. So another way to think about it was just a kind of a call to arms to just be a bit more holistic.
[00:10:10] Dara: Is that silo effect, is that unique do you think to the kind of digital world, do you see that in more of a either in government or in, in the kind of academic space? Do you still think that silo effect is there where people are not seeing the whole picture and they’re looking to kind of say, oh, that bit’s not our responsibility?
[00:10:28] Shaun: So this is probably where this recording gets a bit controversial. So if you work in a crumbly old enterprise or public sector bureaucratic organisation, there are lots of good reasons why you are siloed. If you’re in a digital organisation, what is your excuse? Your whole business is data. So I think, you know, I tend to divide businesses into those born before the internet and after the internet is kind of the, a good dividing line, right?
[00:10:50] Shaun: So like, you know, Spotify is a well-respected kind of brand in terms of how it did a lot of its early work amongst product people, CRO people, data scientists, data engineers, software engineers, and their whole product and everything that they came up with in terms of ways of working, you know, was made possible by not having any physical products or assets.
[00:11:12] Shaun: And so, it’s 10 to a 100 times worse in enterprise land because people are physically in different places or all of that. And so it was maybe a little interesting to step into a more digital world right, which is probably the biggest difference about the data community that you guys are more a part of is that it is very digital and I guess some of that’s digital parts of enterprise companies, but all that tells me is that humans are humans and organisations are organisations regardless of the raw material that they’re working with. It technologically should be easier in digital land but being in physical analog enterprise land, it means you always have the excuse of, well, we didn’t measure that. So the theory of behaviour is easier because you’ve lost 99% of the relevant data. In digital land you can instrument everything and then you have a real hard time working out what to experiment on I would say.
[00:12:05] Bhav: So Shaun, it’s been five years since our talk. The question I was going to ask you is why do you hate A/B testing? But that’s, I feel like a bit of a knob asking that but what’s changed now? Has your view changed on A/B testing? Do you feel like you still hold the same views? Obviously your role has changed, you play more of an evangelist role going from companies and organisations and conferences talking about data science. What have you seen in the world? Are people doing it better? Are people doing it worse or is it about the same?
[00:12:28] Shaun: One thing I’ve kind of observed from the side on that platform that used to be called Twitter, which you know, I don’t know if anyone’s still on there anymore. Now it’s mostly on LinkedIn. I would say that when I was doing research to prepare for that talk. I know you think I prepared it all in the moment, but when I was looking into, hey, who are these CRO people that I’ve never met? Because they use all the same technology and talk about 90% of the same things, but they seem very different. There seemed to be a bunch of debates about these themes we’ve been talking about that were in early stages, I would say five years ago.
[00:12:58] Shaun: And I know those things never really get resolved, but I think probably fewer people in five years on are kind of building their own very elaborate experimentation platform that would be good just for their one company and more people are probably using more scalable third party products, that’s what I’ve observed as a sort of side observer of this. And then I think that that phrase I used causal inference, right, has started to get in. Part of that is actually, yeah, machine learning and AI stuff, like those noises bouncing out in these companies and then refracting back in and maybe sharpening people’s thinking about the purpose of all of this. So that’s what I’ve observed, the debate on your side of this made up fence seems to be much more about like, how do we implement some pretty solid ideas and not screw it up completely? And what I felt, even in that moment, on that stage five years ago, I was like, wow, there’s people are about to spend a lot of money, right? If it was free and easy to do whatever you want it doesn’t matter.
[00:13:57] Bhav: But it’s almost free, right? You mentioned that running any type of experiment when you are in the government side is very expensive. I guess one of the reasons why companies can run 500 experiments in one go or over the course of the year is because it’s so cheap to run, you’ve already taken the upfront costs of the platform. You’ve paid for ’em upfront, you may as well utilise them and get the most out of them. My problem with this has always been for me, experimentation, A/B testing. I’ve never used the term CRO, I know that’s the general term for the industry, but I always use the term experimentation. For me, it’s a science, but I think a lot of the people who do it don’t come from a science background, and therefore you’ve kind of got this sort of pseudoscience happening on experimentation.
[00:14:36] Bhav: And that, for me, has been the biggest problem. And actually there are a handful of people I talk to in the industry who really think of experimentation as a science and less as an art. Maybe there is an element of it you know, in the grand scheme of things, everything can be mixed, but there is this complete lack of science happening from the majority of the people when you look at their backgrounds, they don’t have the background. And maybe I’m being a bit pompous here and I’m, you know, I’m being a bit stuck up about it, but when you’re doing things that are measuring, you know, marginal differences in two groups, and you’re trying to determine the effect of that in the grand scheme of things, that’s a science.
[00:15:08] Shaun: But I think generalising, so I agree that it’s a science, but I think generalising is still not, well generalising is science. But generalising is not the white lab coat version of science. It’s the engine of human progress thing right? Which is when you need information from outside your experiment to contextualise it and to understand why did we run these 500? Why not some other 500? That was the thing that probably bugged me the most, like stepping a little bit into your world was here’s a bunch of people saying that they’re super duper white lab coat scientists, and no one can tell me like how they came up with those 500 things and did those things and that that seems to be highest paid person’s opinion (HiPPO) or what we felt like doing on the first day or a ticket came down the system and so we did it.
[00:15:52] Daniel: That’s always been the thing with CRO, A/B testing, experimentation is that, that’s been the thing I’ve noticed the most, which is that people are quick to the tech people are quick to maybe even the analysis of the results and being aware of the kind of nuance around or the things to be mindful of, but actually about running the experiments, it’s like, well actually there’s some politics internally that meant we had to test the checkout flow when actually there was nothing wrong with the checkout flow or something like that. You know, actually there’s some other bits elsewhere to do and I’ve always found that the biggest hurdle, the biggest reason, maybe think of it this way, a biggest reason why a kind of testing culture or A/B testing platform doesn’t find success within an organisation is because they don’t run the right tests.
[00:16:32] Shaun: You know, someone with much more expertise in that aspect has come to the same conclusion. So it is cheaper, Bhav, right? But it’s still a choice, right? You can’t have an infinitely sized team.
[00:16:43] Bhav: you don’t have infinite size of traffic either unless you’re an Amazon or Netflix or something like that. You don’t have infinite level of traffic. So you do have to be very selective about what you do. And that bit, obviously for me, is still part science. If you can do 10 things and, but sorry, if you have the option to do 10 things, but you can only do 3 of them, the decisions required to pick which 3 require a certain element of science and methodical thinking and prioritisation as anything else, you know? Otherwise it does just become a case of throwing shit at the wall and seeing what sticks right.
[00:17:15] Shaun: In the discussions afterwards, the kind of schism that I detected in the, even in the audience was that there were a lot of user experience, user research people who probably do have quite rich, well-founded theories about how people might respond, that would make bigger predictions than has an effect or not right, because like effect size is important, right? Yeah.
[00:17:33] Daniel: There’s a time and place for this kind of red button, blue button testing, or maybe just testing the HiPPO requirements or maybe just the politics internally. I think one thing, like especially when we go into the kind of science side of things, or if we go into the kind of doing things quote unquote properly, like it requires headcount, it requires money to be spent on this thing to make the most out of it, but also you have to kind of invest upfront. Whereas actually, I find a lot of this and I found this with things like the kind of cheap or free tools that Bhav mentioned earlier is like, it’s a kind of getting a taste of it and it’s kind of like proven a justification for investing properly into this world of experimentation, of testing, of CRO. And actually, it’s like a maturity thing. You’ve got the very, maybe experimentation immature companies that know that they’re immature on that scale, which is already a step ahead of most other companies, but they’re actually dipping their toe in the water by testing button colours and using these off the shelf solutions and not doing anything outside of the platform in terms of identifying winners.
[00:18:27] Daniel: But actually that’s kind of getting a kind of buzz and an excitement and the culture around testing so that they can hire someone, they can devote some endeavour resource towards it or, or something like that. But I always find there’s a time and a place for that, and it’s not about staying there and perpetually testing button positions and colours just for the sake of it. You know, polishing something that could be, you know, a hundred times better if it was just different. But actually it’s a means to an end. It’s that kind of journey that companies have to go on. I’ve always found that to be a really useful use case of running a stupid red blue button colour test just to kind of get people excited.
[00:19:01] Shaun: And the thing you’re running up against there is like, and actually amongst all of the data people, the thing that, you know, the particular set of techniques you are trying to increase the maturity of right, and get more value from are some of the most damaging to existing organisational decision making structures. If at the board table they’re having a really robust informed debate about should we do X or Y or A or B, you present a methodology to adjudicate that right? And that is super scary, right? And now a lot of other data people, data analysts who don’t work in experimentation, data scientists who are doing whatever it is that we do, sometimes calling those experiments weirdly, even though they’re not but that’s a whole other topic for another day. Those people always have this out of, oh, well we were just analysing stuff anyway and you can take my recommendations or not, and organisations are complex, but because your particular flavour of data people have made this stand that here’s a methodology that needs to be taken seriously and done the right way and it is extremely challenging to put a lot of the ways that product or budget decisions get made, which probably that pressure probably keeps a lot of organisations lower than they should on that maturity curve, which is a shame.
[00:20:13] Bhav: I think product folks don’t like A/B testing. And I because it goes directly, it flies in the face of what I call the Steve Jobs complex and the Steve Jobs complex you know, Steve Jobs always knew his customers and that was okay. He knew what the market was heading towards and he never had to A/B test, arguably right. I’m sure he maybe did.
[00:20:31] Shaun: There’s a difference between not A/B testing and like having really good operational secrecy on all of the failed prototypes. Yeah, driven by ego on Steve Jobs’s side right. And yes, he was a visionary of some sort, I don’t mean to defend that view of the world and most product people are not going to be as good as he was at that, but, In his defence, he did have a very well grounded and coherent theory of why people use the kinds of products that he was wanting to build.
[00:20:59] Bhav: But I think also he was able to think at a more macro level, right? I think when you are a product manager for a payment platform, it’s hard to have intuition about, you know, your next payment test or feature you’re going to build. I do want to say one thing and you know I’m going to puke in my mouth when I say this in defence of the CRO industry, which are words I rarely ever say. I think these concepts are sort of like colour testing. I think the people have moved on a lot from this. And the other thing I was just going to add onto this is one of the challenges around A/B testing experimentation and the reason why it’s done wrong. And I guess it’s kind of like data science, I’d love to hear your views about how this relates to data science, is that it’s become so democratised and accessible for everyone that there isn’t some guardian of truth available in every organisation to ensure the quality of experimentation is at its best. So people are doing you know, half-assed tests based on, on a whim, on on very loose hypotheses, whatever you want to call them, very little research because they can, they’ve been given the platform, but none of the thinking that’s required that goes into A/B testing. And I guess in some ways this is kind of like data science because of what you do and where you work as well.
[00:22:04] Shaun: No, completely. I can share very openly. So I work for Dataiku, which is a data and AI platform company. So it’s software that lowers the entry barrier to machine learning, and now we’re much less afraid in 2023 to talk early about AI than we used to be. You know, a year ago, if you were talking to a less data science-y community and a customer, potential customer, you would sort of sneak AI in it, don’t say AI too early they’ll freak out. Now, everyone wants to know how they can take advantage of this stuff upfront, but your concern is valid because it can lead to unexpected and undesired results. What makes you a bit of a stinking elitist by peddling that, you know, peddling that opinion on this widely circulating platform of this podcast is who’s to say that the guardian of truth self-appointed was the guardian of truth.
[00:22:56] Shaun: And the reason that I can, you know, we go back away and we have that relationship of trust. But the real reason I feel comfortable saying that is that, that’s something that I would’ve believed quite deeply as recently as five years ago, right? I am the kind of data person given my background and how I came to it, and you know too much education and time to think about stuff. Oh, do we really want to give people more data and more tools? They’re just going to get the wrong answers, right? They’re just going to screw it up. And I think data land in general has maybe gone through that. So even when I started Dataiku two and a half years ago, that was a very frequent thing when we would try to, even to people who were relative experts using the Dataiku platform, when we would try to sell them more Dataiku. But what about your finance team? What about these other adjacent teams or teams that you serve or work with? Would you like to give them more powerful tools? Oh no, we don’t want to do that. And then slowly, organically, a couple years later, that’s a very infrequent criticism or complaint.
[00:23:57] Shaun: And it, you know, teaching everyone to read and write was a good thing in the long run, right? Early on, probably some people were annoyed that people who couldn’t read and then people who couldn’t write now had that power. It’s a very butchered kind of metaphor, but I think to like have any hope for the future, you kind need to believe in change and this idea that more people from more basically diverse backgrounds, right? Not just self-appointed experts, are going to slowly work towards the right answers, but, It’s like a reversion to the main thing, like there’ll be lots of screw ups along the way. So it’s in the end, it’s a generic scaling question, right? If you want to apply a given set of tools a lot more, you’re going to have to accept, right, some mistakes are going to be made. What we usually say at Dataiku is, If it’s that domain team, finance, marketing, whoever, like making their own mistakes with the ability and some guardrails to help correct them, it’s probably a better way for the organisation to learn overall than to have some, have their problem put in a ticket and shipped to a remote expert who doesn’t know the context then you are in silos and crossing silos and different people caring about different things.
[00:25:03] Shaun: So maybe your part of data land is ahead or behind of that. But I think it’s the maturity thing Dan was saying. Early on, it’s just going to be the people who are really into it, then it’s going to get trendy a lot of people are going to do it. Don’t worry, they will lose interest and do some other things. And if that can help build a business case for having a community of practice or a centre of excellence or whatever you want to call it, to put those guardrails in place, but putting those in place before you’ve had the screw ups it’s going to be a really hard business case because you are like, how do you value not making mistakes in the future?
[00:25:32] Bhav: No so I think when I meant like having like a guardian or a custodian of knowledge for this, it’s not so they act as a barrier for people to develop. It’s so that as people develop.
[00:25:42] Shaun: But they inevitably do.
[00:25:43] Bhav: Well it depends on, well this then goes down into the discussion about organisational structure. And I think there was an element you guys were talking about around, you know, research teams and things like that, and I think there is an element of team structures and company structures that plays a big role. I think I’ve just been fortunate that my role has always been evangelization of data analytics, experimentation, and to be able to disseminate that information across the company in a way that is done by embedding analysts into teams as opposed to having people come to us, we kind of bring it to them. So there isn’t this ticketing shit funnel that you’re referring to.
[00:26:15] Shaun: And it’s the way to do it you know, the data world overall is going through a mess of re-decentralisation push after a number of years of back and forth one of the trendier ideas out there recently has been something called data mesh, which is pushing the capability back out to the business. But it’s very hard to do that if you have no capability to start with right? So I think it is just that maturity thing, like before you push some experts out into the business to train others, there need to be some experts and the likelihood of them developing in a really useful way within those silos and never meeting each other right, would be, it would be very low. You know, software went through the same thing 10, 20 years ago as well.
[00:26:51] Bhav: Is generative AI going through this now?
[00:26:53] Shaun: Definitely too early to tell, but like for me, a year ago I was like, the world doesn’t need more art of that particular flavour of a Midjourney, you know, fantasy art, like nothing against fantasy art if that’s what you like, great. I’m not going to hang it on my wall and so, yeah, that was a year ago, and then six months ago I was like, okay, no, this is I think for everyone there’s a, there’s a use case or an application of it that really gets your attention. And for me, some of it was the coding stuff, but more in a terrifying way. And then, once I just started to see ways that it could be applied just to like how people work with data, like just in very simple workflows. For example, you know, schemas of data and joining stuff together is still somehow really complicated. The data on what could be joined to what is literally sitting there in the database, data lake, data platform, whatever you want to call it. And most of people who get far enough in this have a script they wrote one time that pulled something together and tried to match some, match some stuff. And so I think, yeah, it’s way too early to tell what will happen with it.
[00:27:54] Shaun: But I think what’s different about it, what’s different about it is it has gone straight for consumers, right? And so what that changes for everyone working with data in any way in an organisation, is I think it has and it should do more hopefully raise the expectation of what those consumers of data, be it data generated by experiments or anything else. Like, hopefully they start to expect a lot more because they’re starting to see data and AI actually be relevant in their personal lives rather than this weird thing that they just do at work and then ignore elsewhere. So I think it’s too early to tell, but the level of enthusiasm, the change in that has been pretty remarkable.
[00:28:34] Daniel: We’re seeing it all with the coding side in Chat-GPT, I’ve had to play with that and even just throwing files at it and it can do some basic coding to kind of visualise, to blend, to calculate, to do anything off the back of it without even knowing you’re doing it. And I think this, for me, and having not worked in this space on like yourself for the last couple of years, I mean, and just being a consumer of this, you know, as alongside everyone else, what I’m finding really fascinating is this almost like a slow moving thing that’s kind of moving into the data world. So it very quickly became a kind of like, tell me about this subject matter here and summarise it for me beautifully. Or take this video and give me the highlights or maybe generate me some fantasy image, but where I’m excited about seeing this and where it’s starting to dabble now is in the world of data.
[00:29:17] Daniel: I’m really interested to hear from you, Shaun, in terms of like, what is that next step in terms of the world of data analytics, because the hardest thing that is never truly kind of broken through before is this understanding of data. You can visualise data if you tell it, plot this on this graph and do this to this data, it’ll do it. But if someone says, how is my data performing? You know, how is my website performing this magical P word that doesn’t exist as a metric or something like that. Or they’re saying, why has sales gone up? And these are questions that still require human input. I’m just wondering if you see the kind of the movement towards like AI or large language models or generative AI coming into the world of that space.
[00:29:53] Shaun: I really hope it does because it will deliver on a promise that people have been making for decades, right. Ever since business intelligence became a thing in the late eighties and nineties, ever since you could store more data than you knew what to do with in the moment right. That’s why the data warehouse was really invented. Okay storage is cheap enough and maybe we’ll save this for later because it could be useful, but if you look back at the marketing materials and the narrative for maybe you meet people who kind of got their stripes and scars back then, what were they trying to do? Push more and more relevant data out to the business to take some kind of decision. Then there was, you know, after BI you could maybe say there was this self-service thing, which was somehow different, but exactly the same. And then now there’s citizen data science again. When you squint at it, is that not exactly the same?
[00:30:41] Shaun: The difficulty at least till now with that way of interacting with data that you’ve been talking about is if that was true, if business demand to interact conversationally. How are my sales going? I feel that there would’ve been solved or it could’ve been solved some other way earlier. And so there have been vendors and one of them that comes to mind is ThoughtSpot, the ‘dashboards are dead’ people. And so they took a very different approach right to that. But if I also think about Tableau, it’s had conversational stuff in there, even for data prep for like five or more years, people just didn’t seem to use it, right? And so whenever I tried this little conversational thing with salespeople in the automotive industry who were some of my stakeholders, you know, only three years ago. I think it sometimes presumes a desire to self-serve that the consumer does not actually want.
[00:31:32] Shaun: So that would be the main warning sign for me would be if data people, if people producing data, use this as a way to shift work and responsibility and all the difficult stuff to their customers, to their consumers. It’s not going to work very well because it’s already not worked three, four times over. Each time it’s made more progress. Each time more people have gained skills and knowledge. And so the place I’m most excited for it to happen is actually in the work of data people, right? Like if there’s a group of people most at risk, like if you’re a artisan woodworker, or you drive an Uber or clean a street, you have nothing to fear from AI or generative AI or even robots like robots are just way too, like how are you going to get a robot to clean a street in London, like the size of their problem right? It’s just absolutely amazing.
[00:32:26] Shaun: A lot of people used to say, oh, Uber’s going to take over all transportation. Why would Uber want to have the balance sheet problem of owning a hundred trillion dollars worth of vehicles, right? Right now they just outsource it to an independent contractor who’s responsible for everything and say, hey, we’ll tell you when people want to ride from A to B and then they do other stuff, but their business models kind of very low capital intensity.
[00:32:47] Shaun: But data people like data janitors of whom there are many right people kind of yeah, getting that spreadsheet emailed to them from Jane and then combining it with this thing from Larry and then sending it onto Ahmad in sales. Something’s going to radically change their entire job. Like, and depending on the company, like their job could not exist within 5 years, or it might take 15, but like compared to a year ago where I would’ve said, 15 to 50 years now that horizon is really shortening. People will still want to look at graphs where the numbers go up into the right or be told that by something that’s making up words based on the number going up into the right. So I’ve yet to see strong evidence that conversational questions of data really deliver any value to the person asking. So I think what data people should be doing is like, how can I use this stuff to just make everything I do way more efficient and reusable which turns out like LLMs are quite good at that.
[00:33:42] Bhav: We have a future episode we’re recording, which goes into my biggest failure as an analytics lead or, you know, whatever, and that’s self-serve. You’ve inadvertently just answered a question that’s plagued me for nearly five years, which is why have I failed at getting self-serve to work? And I think it’s because people don’t care and we’re forcing it on them when they don’t want it, I don’t know.
[00:34:03] Shaun: I mean, that’s a, that’s a very good short way to say that.
[00:34:06] Daniel: The big problem with, let’s take large language models like chatbots, specifically with generative AI, and especially when it comes to data, because getting an answer is probably something that it’s going to very quickly be able to do. So it will understand things like data schemas. Even if there’s a nuance, if you ask for visits, it will know, you mean sessions in a platform X, Y, or Z? It doesn’t matter.
[00:34:26] Shaun: It’s amazing what it knows about some quite narrow subject matter areas of at really high quality. So I was running a gen AI workshop at some event recently and some guy was like trying to like fill in the gaps on his data governance strategy or something. Turns out that the only people who’ve blogged about that, people who kind of know what they’re doing. And so what ChatGTP has learned about the finer points and nuances of data governance is like gold.
[00:34:53] Daniel: But the thing it’s going to miss is that it won’t be able to ask the questions of the data effectively. So this is the thing where it can answer if you give it a great question, it will give you a great answer or at least it’ll understand and kind of join the dots. Do those lovely joins you were talking about earlier to kind of get the data out and kind of provide some visualisation, some graphs, some, some answer, whatever, but like the thing that it’s not helping, and I think this is the biggest blind spot when it comes to the huge vast mass adoption of generative AI and large language more specifically is the fact that it’s not helping you ask better questions of it itself. And I know you can ask it to ask better questions and you can kind of go down and, but the thing is, it’s like, but then you have to kind of educate people and people have to be aware and it’s so early to know to ask it, to ask better questions.
[00:35:37] Shaun: It still requires human curiosity.
[00:35:39] Daniel: That’s it, it’s this curiosity and it’s like inception. You go one layer back and you have to know, to know, to ask it, to ask it to.
[00:35:44] Shaun: It turns out you got the same damn problem at every single layer.
[00:35:48] Daniel: Yeah, exactly. Where do you start?
[00:35:50] Shaun: But then how would they know that they need to know well, some humans just, you know, are always asking that next question, right. Maybe the people on this, you know, episode are more tilted that way. Or as Bhav said, they don’t care because they don’t have a reason to care, that’s precisely why the ability to ask some questions, how are my sales doing? I just don’t see that taking off. And then the people who really care about that are already our self-service customers who are asking us different kinds of questions.
[00:36:18] Bhav: For what it’s worth, I think analysts have been trying to get stakeholders to ask better questions for a long time. So this is nothing new as far as I’m concerned. The only problem now is that you’re now dealing with an inanimate platform that is not going to say to you, hey, ask a better question because the thing you really care about is this.
[00:36:34] Shaun: Just a small addition to that, a friendly amendment, right. So because we’re an AI vendor, we’re talking to lots of people about generative AI and one of the things that we are trying to help people understand. If you want to be a big Rambo and like buy those GPUs and like train your own models. You better have much more money just lying around than you pay Dataiku for software licences because McKinsey have done the maths and like, you better be like the Pentagon. You know, if you really must do this yourself, why don’t you instead use this thing called prompt engineering, which if you’ve played around with any of these things, I like to explain that as you’re kind of putting the LLM in the right state of mind through a series of questions to then ask the question that you want.
[00:37:12] Shaun: So any of you can go out and do it, right. We’ve been complaining about people not asking the right questions or not knowing to ask the right questions. What if you provided an interface that helped people ask the right questions or at least better questions? And if they had no questions, they could be suggested questions and they could pick the questions that they liked the most, and then the job of data people to make LLMs useful is to do prompt engineering, right? To take the small human questions right? Put more context around them. Like what is your business? What kind of systems do you have? What questions have people asked of this kind of data on the internet before right?
[00:37:46] Shaun: I think LLMs probably do know a lot about what are relevant questions that people have asked, and so I think. Again, using this to escape hard work is the road to ruin, using it to make hard work easier. And if they can help us wrap naive business questions in something that starts to draw those people into demanding a human conversation to dive into this and just give me the data so I can use it myself, then we will have won. So it’s another tool that we can use to draw a different kind of user who might have been a bit remote from us in just using this conversational element. But it’s going to take a lot of, a little bit of magic in the backend to sort of make sure that the true magic of the LLM is actually delivering more than, you know, those naive dead end question answer things that we all get into.
[00:38:35] Dara: And that typical user is going to get more proficient and familiar with using things like ChatGPT because I think at the moment it’s still you know, you go a level beyond us and maybe a level beyond that again, and people are somewhat familiar and maybe have played around with it and looked up who won the World Cup in such a year or something silly like that. But as time goes on, people will become more and more familiar with it. So they will eventually, even if it’s still out of laziness if they think I can learn what not to ask and I can learn how I need to interact with this thing to get some useful information back then it’s only going to grow, isn’t it?
[00:39:10] Bhav: I’m a consumer of ChatGTP, I have no idea of how it works underneath. And actually, I think ChatGTP has trained me to ask better questions, right? And now I’m in a position where, actually my first question is almost like bang on to what I really want to ask with all the, all the nuances around what I’m trying to ask and all the you know, all of the discrepancies and things that I wouldn’t have asked in my early days of ChatGTP. So, enter fun fact about ChatGPT, it’s the fastest I’ve ever gone from free to paid platform of all platforms. Spotify took me about a year but ChatGPT took me about a week to go from free to paid.
[00:39:42] Daniel: On that Bhav do you think that’s because what, like what Shaun was saying earlier in terms of like maybe we’re naturally more inquisitive or questioning and we have that kind of tendency to want to know how it works and to learn it and get better at it, to your point, Bhav, around it being like data democracy or like sharing, it’s like some people will never want to do it themselves. They don’t want to learn how to get better at doing it themselves either, they want like an immediate result and there’s always going to be those people. As a vendor diagram, there’s going to be an overlap and the kind of the LLMs are going to get those people in the middle, but it’s never going to touch those people on the other side. What I’m wondering is that, is there like a layer?
[00:40:16] Shaun: It’ll touch them as a large boulder rolling over them.
[00:40:19] Bhav: Yeah, I was going to say, what’s that diffusion of adoption model? You know that diffusion of adoption where you have your early adopters, then your keen ones, then you get your laggers. They’re the ones I think Shaun, you’re referring to again, you know, who are going to get steamrolled over.
[00:40:32] Shaun: And I think with just within data land, it won’t be good to be a late adopter of this stuff if your job title was something like data analyst.
[00:40:39] Daniel: I don’t think if you’re in data land, if you’re within the kind of the boundaries of data land and you’re not jumping on this kind of stuff or getting or being aware of how important it’ll be, then I think you are going to be left behind for sure. But I’m just thinking of the other people and I’m wondering then you mentioned something earlier around the kind of like prompt engineering, but maybe kind of like abstracting it slightly a bit, but saying like, could the analysts, the analytics people, the data team, they could pre-prompt a machine and then the kind of consumer could be digesting it through or consuming it through things like Slack or some kind of chat bot. And actually it’s already programmed to understand the business. And if the sales team ask for sales from Facebook versus the marketing team. They might mean slightly different things, but you can pre-prompt, pre-program these things based on the answer. And I think maybe there’s an element there that’s like, you don’t require the end user to know how to ask the right questions of the data, but actually you’re doing 90, 80% of that for them right? And that can be a role.
[00:41:31] Shaun: Yep, and that is in some companies a few months away, other companies a couple of years away. So a lot of people are kind of focused on how do we give a safe version of just ChatGPT to everyone to like ask questions about our HR policies or something. It’s like, is that a good idea? Just even if you do the paid version, and so the prompts aren’t stored for any more than 30 days and they’re not used to train the model. Like that’s the initial bandwagon thing is I want that thing, I want an Uber, but for me, like I want that thing but for us, right, a lot of people are stuck there. In a year or less there’s going to be hopefully a lot of like special purpose models that are, A much smaller to run and therefore much cheaper, whether you are running it yourself or consuming that as a service that really specialise in some different things, right? So it’d be really cool if someone did something that can pull the levers on Tableau based on that.
[00:42:26] Shaun: And whether Tableau do that or someone else does it doesn’t matter. But I think the, it’s the same, you know, waves of adoption we’ve been talking about. There’s people who just get into it. There’s a whole, you know, majority who might use it for like laughs or to cheat on pub trivia or whatever. But the real value is going to come when it’s kind of in lots of different places just helping us get to objectives and be noticing it a much, much less. And that will be the job of someone. And so I’m strongly suggesting that data people, especially data people who know a lot of the pitfalls we’ve been talking about, you know, until we got onto this topic. For me it’s like personally important that those guardians, returning to your theme Bhav, that people who have like messed around and messed up with data, like are at least part of the conversation about how this adoption happens. Wouldn’t it be even better if they were the ones leading that and shipping these internal products to their customers because they know all the ways it goes wrong.
[00:43:22] Shaun: You don’t need to like go and study a lot about responsible AI or anything. If you’re just a data person who’s calculated something wrong and then maybe someone took a wrong decision. Like you know the consequences of wrong data right? Which is what that unhelpful LLM response is at the end of the day, it is just generated data by a different kind of process. So I’m telling everyone I can inside and outside of work like, this moment needs people who are aware of the risks from any kind of prior data background, anywhere in data land. I think everyone’s got something to add to make this all go faster and safer.
Rapid fire
[00:44:01] Dara: Right Shaun, five rapid fire questions before we let you go, things are going to get real now. So what is the biggest challenge today that you think will be gone in five years time?
[00:44:10] Shaun: In five years, no one will need to struggle on how are they going to join data together in some new kind of system they haven’t seen? They may choose to still struggle, but they won’t need to struggle.
[00:44:22] Dara: Okay so what will be the biggest problem in five years?
[00:44:25] Shaun: People will still need better questions all the time. That’s never going to end, yeah. It’s not going to change. And so, yeah, but there’s a positive way to think about that. Yeah, I’m laughing that it’s a problem, but we’ll still have the same problem, but if we get to the real problem faster, hopefully that’s better, I hope.
[00:44:40] Dara: What’s one myth that you’d really like to bust?
[00:44:43] Shaun: The biggest myth that I see is, again something that I’ve pedalled in the past. This idea that there is one linear maturity model for every organisation. So we very commonly hear, oh, I can’t do anything interesting with data or AI or predicting anything until I’ve got all my data perfect. It’s a myth subscribed to by people who have never actually worked with data. Because if you have, you know it’s never perfect and you still have to make a decision happen.
[00:45:11] Dara: Yeah, for the benefit of our listeners, there was lots of nodding heads during that one, so that’s a good answer. So kind of slightly similar question next one, if you could wave a magic wand and make everybody know one thing, what would that be?
[00:45:23] Shaun: This is too much power, too much responsibility. I think if everyone knew that correlation does not imply causation and they knew that it doesn’t necessarily imply lack of causation, that’d be great.
[00:45:37] Dara: Last one, easy one or hard one, depending on how you feel about it. What’s your favourite way to wind down outside of work?
[00:45:44] Shaun: Usually it’s something, yeah, physical exercise, but it’s kind of like winding up in order to wind down. But probably the, I don’t do it as often as I should. But I learned to sail as an adult, so I grew up in New Zealand where lots of people learned to sail as kids, but I didn’t. And then as an adult I learned to sail and sailed quite a bit. And then moving to London, it became a little bit harder to sail? Well, it’s not that it’s impossible, but just doing anything in London is, you know, takes more effort, so if you’re lazy, it falls off. But I was recently on a boat with my wife, my daughter, my dad, one of my brothers and my brother’s father-in-law on a just a beautiful 10 knot day, which is not what I’m used to. And like yeah, my four year old daughter is steering the ship right, and in complete quiet right, I do a lot of stuff with technology. I mean, to have a particular kind of technology that doesn’t make any sound while you are gliding through the water brings a lot of things together, that’s pretty cool.
[00:46:44] Dara: Amazing, great answer. And nobody’s said sailing yet, so you’re the, you’re the first one to claim.
[00:46:49] Daniel: Amazing, well thank you so much, Shaun, for coming on the show. It’s been amazing chatting to you and yeah, getting to ask all these fun questions around A/B testing and generative AI.
Daniel Perry-Reed
Subscribe to our newsletter:
Further reading
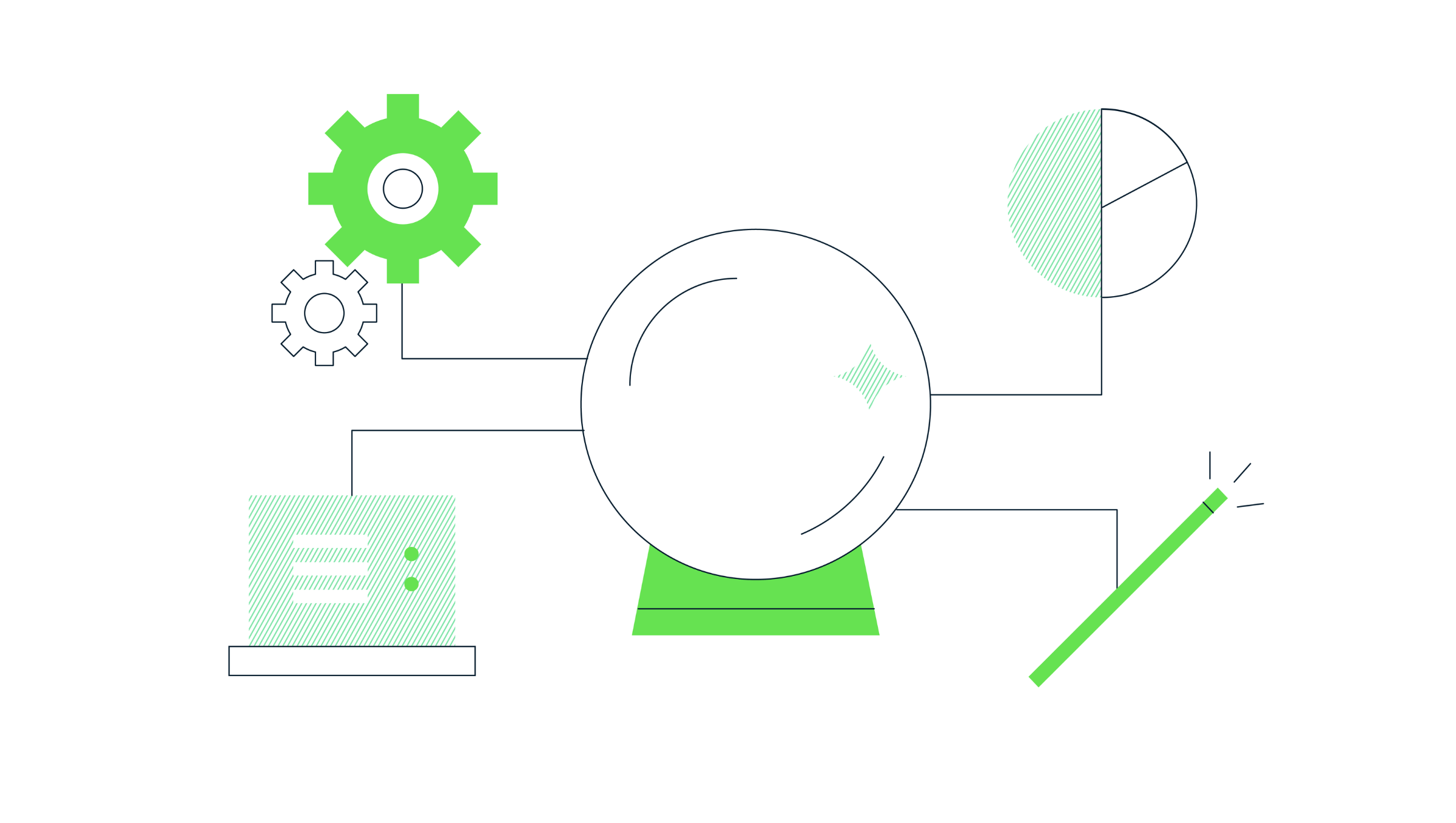
Automating BigQuery workflows with conditional logic and data readiness checks
Mastering data loading in BigQuery using Dataform
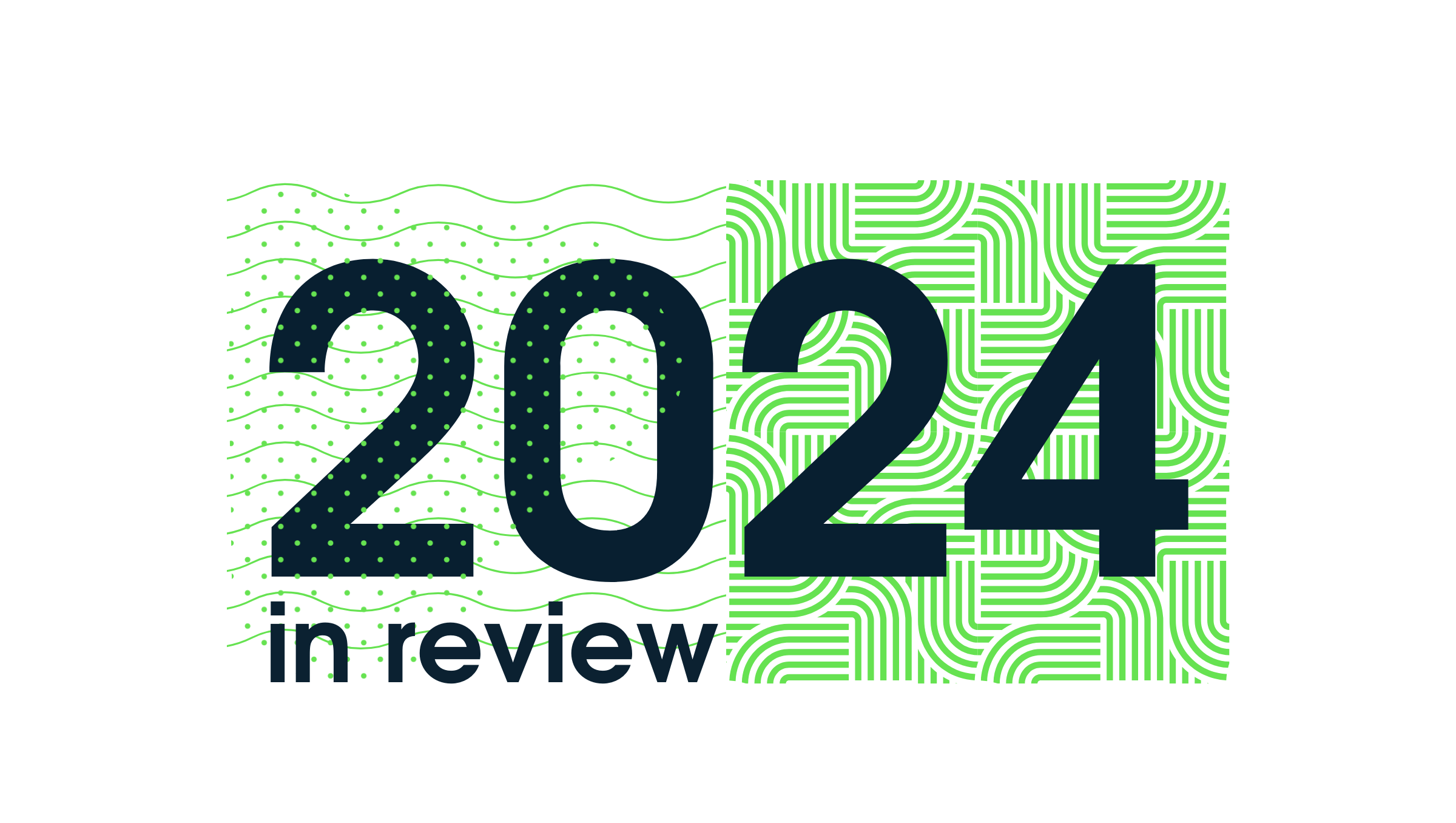