#115 Lessons from a data science content creator (with Egor Howell @ Deliveroo)
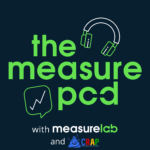
In this week’s episode of The Measure Pod, Dan and Bhav are joined by Egor Howell, a rising voice in data science and content creation. They dive into the differences between analytics, data science, and machine learning, offering valuable insights for anyone in the field. Egor also shares his take on content creation, data-driven decision-making, and the key skills needed to grow your career in analytics.
Show notes
- YouTube channel – specifically How I Became A Data Scientist (No CS Degree, No Bootcamp)
- Dishing The Data newsletter
- Episode 106 – What an ideal expert analyst looks like
- Forbes article on DS sexiest job
- Shaun McGirr
- Bhav’s LinkedIn post on World Economic Forum results
- Simpson’s paradox
Share your thoughts and ideas on our Feedback Form.
Follow Measurelab on LinkedIn and LeanConvert on LinkedIn.
Join the CRAP Talks Slack community and follow them on LinkedIn.
Music composed by Confidential – check out their lo-fi beats on Spotify.
Transcript
YouTube as a platform is more mature… you reach the audience more on a personal level because they hear you speak, they understand you, they see you on video.
Egor
I think what’s happened, certainly I’ve observed like three, four years ago, people were hiring data scientists and they realised what they really needed were data engineers.
Bhav
[00:00:00] Dan: Welcome to The Measure Pod episode 115, this time with Egor Howell. And I’ll start by introducing myself because I always forget to do this in these episodes. I’m Daniel Perry Reed. I’m an analytics consultant at Measurelab. And of course I’m joined by Bhav Patel, director of experimentation and analytics at LeanConvert.
[00:00:32] Dan: So Bhav, we’ve just ended our chat with Egor and. It was incredible. We haven’t really spoken to anyone like Eagle before in the world of data science, content creation. and I find it fascinating. And as you said, possibly one of our youngest guests, you know, living and breathing that kind of culture of content creation and YouTube.
[00:00:49] Dan: What was your, what was your initial reaction?
[00:00:51] Bhav: Oh, yeah, that was a rollercoaster of an episode. I feel like I had so many more questions that I could have kept going and going, and I feel like this is already turning out to be probably one of our slightly longer episodes, so I won’t, I won’t. Take up too much time on the intro side of things, but it was a, it’s really worth listening.
[00:01:07] Bhav: if you’re in the field of analytics and you’re kind of thinking about like, what is the difference between what I do versus what a data scientist does versus what a machine learning engineer does and what are some of the skills I need to harness to kind of supercharge my analyst job. You don’t need to like, want to move into it.
[00:01:22] Bhav: I don’t. I personally want to transition to data science, but I appreciate the advice that Egor has given. I agree. I, I, I followed a lot of it over, over the last few years, myself, in terms of being able to supercharge my skills and do more data science. You work, even if I don’t, even if it doesn’t come with a, the job title, the pay package.
[00:01:40] Bhav: So I would say it’s, you know, it’s worth it. And, Dan, Dan, you’re absolutely right. This is. One of the more unique episodes in the sense that we don’t often have an equal like person on our call, you know, he was, he’s, he’s approaching the world of working from a very different way that we would have done when we would have entered.
[00:01:58] Bhav: Right. So he’s come through the sort of like the content creation route and how that’s amplified his ability to land a job. Of course, he’s worked super hard, you know, it hasn’t come easy, but. He chips away chips away chips away. And so it’s a great example of and he’ll talk about it It’s it’s a process right anything like this.
[00:02:14] Bhav: It’s a process. content creation is a process learning Analytics and data science and machine learning. It’s a process. So
[00:02:20] Dan: yeah Well, and if you’re wondering what all these terms mean and what the hell data science is We try and cover that as well because I had to ask the question So stay tuned for that.
[00:02:28] Dan: all I say is enjoy the episode with Egor all the show notes are full of links of all the things we discussed today. So head over to the measure lab website, link in the description of whatever app you’re using to watch or listen to this. last thing back before we jump in with the episode anything to plug anything to say anything coming up in the world of Bab
[00:02:45] Bhav: one of these days, I will have something to plug at this stage in time I have nothing to plug except for last night.
[00:02:50] Bhav: We held crap talks 27 Don’t know when you’re listening to this. The next one is in march put into your diaries. It’s in london You would be great to see more people again. then probably march 12th or 13th
[00:03:02] Dan: amazing Well, let me follow that up with a crap talks brighton plug crap talks brighton number two Are we going to be scheduling that for the 4th of march?
[00:03:11] Dan: So another one in march, I haven’t yet to do this But by the time this episode comes out, there’ll be a meetup page just register. It’s free to attend. There’s drink. There’s food, great people and great energy. We’d love to see you there. All right on that note Let’s jump in.
[00:03:27] Bhav: Hi Egor, welcome to the measure pod with me and Dan. It’s really nice to have you here. I’ve been following your work on LinkedIn for quite some time now, and I’ve been seeing your numbers grow and grow to a phenomenal rate. So, why don’t you intro yourself? We love to give our guests a chance to tell us their origin story in their own words.
[00:03:42] Bhav: So we’d love to hear from you.
[00:03:44] Egor: Yeah. I mean, I’ll keep it fairly short because it could go for ages. so yeah, I’m Eagle. I’m currently a data scientist and machine learning engineer. I’ve been working in the field now for three and a half years. Originally, I had, was doing a master’s in physics, University of Surrey.
[00:04:00] Egor: So that was from 18 to 20, 22, kind of drew my, my, my year. I basically, my, my starting point was basically I wanted to do physics research full time, and then basically drew my studies. I realized physics is not for me. It’s a bit slow, not very fun. And then, kind of, I, I’m a very, what’s the word, philosophical person.
[00:04:20] Egor: I think things happen for a reason almost. or like things come into your life that you can take advantage of. So I think when I was midway through my research year, the AlphaGo documentary dropped. That was in my homepage feed. And like, most people wouldn’t click on a two hour documentary video about AI, particularly because it wasn’t that popular at that point in time.
[00:04:40] Egor: Like it was known, but it wasn’t like as it is now. And I watched it, I was like, oh, I was blown away. I found it so interesting and basically from then on got into machine learning and then just started teaching myself data science and then eventually landed my first graduate role after university. So nothing like particularly special wasn’t like I wouldn’t say like it was a massive struggle as in I know people struggle to find a job after university.
[00:05:02] Egor: Don’t get wrong I did I would, I would say like, I did try really hard, probably harder than a lot of other people probably would don’t know if that sounds arrogant, but I think that’s pretty, that probably is somewhat true because it wasn’t like just instant my first job. I had to apply for loads and loads of hundreds of jobs just to get it.
[00:05:17] Egor: and then when I got my first role, I basically just started blogging on medium because that’s just to upskill myself. I’m always trying to look for things that made me stand out with other people because I don’t, I don’t, it’s a bit boring just doing the same thing as everyone else. Right. so like medium was a place purely just for me to like upscale, learn more about, learn more about data science, teach myself more.
[00:05:36] Egor: And to be honest, just to make it look better for employers. Because, you know, there’s a famous saying from JP Morgan. It’s like a man has two reasons of doing something. The official reason, like the real reason is very much like, you know, it’s saying you do two things. You do one because of the politically correct thing.
[00:05:51] Egor: Or like you do one because it looks good. And there’s a second reason where there’s a real reason why you’re actually doing it behind the scenes. so that’s kind of my approach to blogging. And then from there transcended into YouTube and to other content essentially, which we can discuss in more detail.
[00:06:06] Dan: Well, yeah, I mean, let, let’s do it. I mean, because, I mean, just Just talking of YouTube. I want to talk about one of the videos that’s, that’s, that I watched before this call, but I mean, 15 and a half thousand subscribers on YouTube is not nothing to be sniffed at. Right. I mean, this is incredible.
[00:06:19] Dan: When you say I just do some YouTube videos, blog on medium, this is a big, big kind of audience. And it’s kind of well, from my perspective, gone, you know, these, this is really interesting to see what you’re doing there and where you’re taking that. So. Maybe talk to us a little bit about that. Like, how did you find yourself making YouTube content, talking about data science when, for one, you have a data science job, two, you’re writing on Medium, three, you’ve probably got a life outside of data science to get on with as well.
[00:06:42] Dan: So what, what kind of drove you to, to, to do this kind of content creation?
[00:06:47] Egor: So, like I said, originally Medium was a starting point just for me to document. My understanding and learn better and just learn more and a bit, obviously it stand out in the market then if again, the real reason why I was doing it medium, so you transition into more of a side hustle, like it was only decent income for me.
[00:07:05] Egor: So not like millions or thousands, like a few hundred pounds a month. realistically, and then at one point, I remember I think August 2023 medium had a problem where not a problem and I changed the way it distributes earnings. don’t get me wrong. I always say I don’t write purely for the money, but the money is a big benefit of why I do it, right?
[00:07:24] Egor: I wouldn’t just constantly upload, upload, or like post blogs if it wasn’t. Any financial benefit, to be honest, because that’s like a side, like it’s like a side tangent to it. And then at that point, I was like, well, okay, like my earnings were actually slashed and I’ve always wanted to do YouTube. I’ve actually had a few YouTube channels in the past, not data science related, just like other things.
[00:07:43] Egor: I was a younger, like FIFA or like gaming YouTube channels. I was like 14. So I have a lot of experience on YouTube and I knew how, not experience, but I knew how it worked. I’ve done, I’ve dabbled in the past. And I was like, well, okay, again, another side of like, there’s something telling me here that is, that I should be trying to do something else.
[00:08:00] Egor: And so YouTube as a platform is more mature. I think you reach an audience. You’re more kind of, you reach the audience more of a personal level because they hear you speak, they understand you, they see you on video. Blogging is less kind of connection to the audience. And I’ve always wanted to do it from like a young age.
[00:08:17] Egor: I was just always too embarrassed. But then, you know, as you get older, you overcome it. And I just started. and it wasn’t really a big step for me because I was kind of comfortable putting myself out there anyway. first video is absolutely terrible, still my channel, dreadful, but like everything in life, you just take time and eventually you build up.
[00:08:35] Egor: and like I said, I think YouTube to me is like the thing I’m most passionate about, like I really enjoy video creation, I enjoy talking. I enjoy filming process. I enjoy learning how to film. And the point was, like, most of my content on YouTube initially was just, like, lecture style videos on time series analysis and forecasting and then, like, probability distributions.
[00:08:55] Egor: I made, like, an hour video on Bayesian statistics, which no one watched, but I did it anyway. and then eventually like those, you find what hits the audience more and people want some more advice based content. So eventually I started just doing more like how to get a day assigned to things you should know.
[00:09:10] Egor: And at this point in time, I was still quite junior, like two years, three years in, but you speak to the beginners because the beginners are the biggest audience anyway. You know, how many mid level data scientists are there looking for content? Probably not many how many people looking to become data scientists a lot and even now if I try to appeal for mid level data scientists Don’t get much of an audience there.
[00:09:30] Bhav: It’s really funny We actually recorded a podcast really recently dan and I we had a question on one of our Slack community channels that we’re part of, both Dan and I are part of, and someone was looking for mid level data analyst material. And we respond, both Dan and I responded to this person and we were like, just saying how actually the reason why there’s so much more basic level material out there, it’s, first of all, it’s, it, you can take a cookie cutter approach to it, where it’s kind of like universal.
[00:09:56] Bhav: It’s a very applicable across many different roles, regardless of like, you know, if you’re a, Product analysts or a marketing analyst, like the underlying skill sets are very similar. and the size of the audience is huge, but actually as you progress, further and further up the ladder and you become more and more senior, the people available to do the, do those videos starts to shrink.
[00:10:20] Bhav: And actually there aren’t that many that want to do it. Plus hold down a full time role in a, you know, a mid to a very senior role and do video creation. So we actually, we, it’s, it’s funny. He says, and this resonates really well, both Dan and I literally recorded a video around what advice to give to sort of like someone who’s entering into that mid levels stage when there isn’t that kind of content available. So it’s, I hear you man..
[00:10:43] Egor: It’s quite interesting because I, I, I understand what you’re saying is quite particularly in the data science space. We’re very less developed than software engineering. If you go on YouTube, look at software engineers content, there’s a lot more. If you go to data science, very small machine learning, very small.
[00:10:58] Egor: I have a slight hypothesis in that because if you’re really, really, really good machine learning engineer, data scientists, or like you’re really good, you’re probably not posting content because you probably prioritize your career over anything else, if that makes sense. I kind of know that. Yeah, I’ll, I’ll give you, I’m not saying I’m great.
[00:11:16] Egor: I’ll say I’ll be, I’m a decent data scientist, but I’ll never be the best of the best realistically, because it’s just too hard, but I can be the best in my kind of intersection of content and data science. so there’s people out there posting content. I mean, I can’t give mid level advice cause I’m still mid level, so it’s a bit redundant for me.
[00:11:34] Egor: but hopefully as a progress of the ranks, I can give more tailored advice and hopefully the audience can follow me on my journey, because that’s what I’m trying to do. I’m not trying to like tell people the best way of doing things. It’s just. This will work for me.
[00:11:45] Bhav: Well, this is why I think your journey is so good because you are, I don’t say early stages because you, you know, by your own admission, you said you’ve been doing it for three years now.
[00:11:52] Bhav: So, you know, in data science space, you know, like it’s like dog years, right? the, that’s, that space develops very fast and very quickly and, and everyone’s learning and developing and understanding it. so I think in, you know, it’s in, I think that’s why I think your channel is so interesting is because actually this isn’t someone who’s talking down and At people, it’s someone who is very much at the grassroots stage, maybe not quite grassroots, but at grassroots stage, and you’re taking people with you on that journey of your own development and sharing your knowledge.
[00:12:24] Bhav: And, and actually it’s quite a, it’s a very unique angle because you don’t see that very often. And so, yeah, no, that’s, that’s, and that’s why I was so keen to have you on the, on the show. I was like, I saw your post recent, you have not only are your, YouTube numbers. Great. You’ve also just surpassed 5, 000 subscribers on your newsletter right.
[00:12:40] Egor: Yeah, the newsletter is a car crash for news that I say this every time if you read my post that everyone and everywhere and anywhere about anything I want to write about. I mean, there’s a slight focus on data science and, like machine learning and kind of like the business side. But if you read some of my recent posts, they’re all about like mental health.
[00:12:58] Egor: So it’s actually quite a wide range of things. But like, as I kind of what’s the word? developed, developed like stuff on a development audience. I’m very transparent, everything I discuss now, I don’t try to hide anything, pretend to be something I’m not. obviously YouTube is purely helping data science.
[00:13:15] Egor: The newsletter is more like get to know me and like a bit of personal wisdom and just see, like, you know, people have problems, you know, like everyone else does. so my newsletter is kind of, I don’t know if you’ve read a few posts, but if you scroll down there, the history, it’s, It’s not very data science focused, but I don’t try to make it purely data science focused necessarily.
[00:13:34] Bhav: It’s funny. My medium is very similar to your kind of like that newsletter approach where it’s not purely focused, focused on data analysis. I kind of bounce between subjects which are very relevant to me. So you’ll see, you know, you know, there was a post I wrote about what it’s like. Managing a team remotely and built, you know, like, and this was written at the peak pandemic.
[00:13:54] Bhav: I was just going through, I was like, what, you know, what works well, how do you succeed when you’re working in a remote environment? and, you know, and then there was another one on how do you hire a product analyst, which is, it’s, it’s still a very nascent role. So that was a problem I was struggling with.
[00:14:08] Bhav: And I kind of like, I don’t say I solved it, but like you, I was solving it. So I felt like it’d be fun to write about. So, no, I get that. I think it’s, I think it’s kind of cool that it’s a mishmash of things, but your YouTube channel is very much tailored and focused on, on sort of like this very specific discipline that you’re part of.
[00:14:24] Egor: Exactly. I want to kind of also get away. I think LinkedIn is very sterilized. I want to get away from that kind of view of people. I mean, depending on what industry you work in. But I always want to be just I have a tendency to overshare, I think, and be very vulnerable to people. Like, I always keep what’s on my mind, and like, if I have feelings about something, I’ll let you know.
[00:14:43] Egor: I won’t try to hide myself. Only because I feel like, what’s the point? I don’t know. I’d rather be open about everything I have. And if people don’t like it, they don’t like it. I’m not hurting anyone. I know some people will say like, you shouldn’t share if you’re online. Like, don’t get me wrong. I don’t share.
[00:14:55] Bhav: I disagree. I share everything. My wife says I share way too much online.
[00:14:58] Egor: Do you? Yeah. I mean, it depends. I’m not going to share my personal relationships with other people. For example, like I’m not going to say like, Oh, me and this me, my partner or me, my ex partner did that. You know what I mean? That’s like their private life, but it’s about my private life and my struggles, that’s fine because it’s me.
[00:15:12] Egor: I’m not sharing about anyone else. And I do that because well, why not? And also it’s cathartic. I always say that, you know, writing about your problems. And normally the response is quite good.
[00:15:21] Dan: I mean, like, it’s, it’s really interesting. You talk about that. So, and I mean, like, let’s, let’s go to one of the examples you, you gave him in that video around how you became a data scientist.
[00:15:29] Dan: So I’m thinking for those people that are listening, that are interested in this concept of data science, maybe they’re, it’s still a bit alien to them. Maybe they’re trying to get into this world themselves, or it’s an area that they think they might enjoy. I remember one part of the video when you explained your journey, I think you said you applied for over 300 different jobs before you got your first, data science role and, and I think, you know, so when we talk about sharing your experience and your story, I think it’s, it’s really relevant.
[00:15:53] Dan: It’s really valid because you’ve, you’ve been through it. This isn’t just, you know, Oh, I applied for a job and got it and I’m going to document it. It was an approach. So that, that video, by the way, we’ll link to it in the show notes. That was, that was a year ago. And you gave a bunch of advice on how to get into data science, right?
[00:16:08] Dan: Let’s take a step now, right? Let’s say someone’s asked you, he goes, Oh, I’m really interested in this data science, where do I begin? What do I do? Have you got any advice of how do I get in? I wonder if your opinion on that has changed since then.
[00:16:19] Egor: Yeah, I mean, it’s, it’s always a, it varies year on year. Obviously I’m not in the initial stage anymore, so it’s hard for me to, be precise and then field does move in three years. I would say now it is harder, but I always caveat that. Well, it’s always been hard when I was applying for jobs. It was post COVID. It was hard in a few years. It was redundancy. It’s hard.
[00:16:38] Egor: Like, I don’t think I’ve ever heard a cohort of people complain that it’s not hard to get a job. So like, in my opinion, that’s just like a given anyway, you’re going to face, in terms of how to get started. It’s always like, everyone has their advantages and disadvantages, and you’ve got to use them to your advantage.
[00:16:54] Egor: So, like, obviously, if you have a STEM background, like me, if I’m a Master of Physics, you’re obviously going to have an advantage. I don’t hide that. I mean, people always say, like, can I do it with a degree in, you know, history? I’m like, yes, you can. It’s harder, though. It’s just, like, not beating around the bush.
[00:17:07] Egor: It’s understanding that it’ll take time. the main thing is like, I’ve given so many roadmaps online. I think I always say to people, it’s, it’s just, it’s one of these things that getting into data science, I’ve written an ebook about it. It’s hard. You know, it’s simple, but it’s hard. The process you follow, you just got to learn, build projects, optimize your CV, apply for jobs.
[00:17:28] Egor: It’s, it’s just, it’s a very, very simple, like everything in life is very simple. It’s just hard work and consistency. And I know it sounds very cringe and people say like, Oh yeah, fine. You’re saying this. But that’s, that literally is the truth. I don’t, I don’t understand why it’s people try to find hacks.
[00:17:43] Egor: There is like kind of things you can do to be better, but I always say to everything, it’s just time, patience and playing the odds and eventually you get somewhere. That’s just how it works. You should go increase your, your life.
[00:17:53] Bhav: That’s classical human behavior. Right? I think instead of doing the doing this thing, they’re watching videos of you on how to do the doing right.
[00:17:59] Bhav: When they could just watch, just go and start it. They’ve watched the video, they go and do it, but no, they want to watch the next one, next one, next one. The next thing you know, that Procrastinating. so I’m going to bring it back a little bit to sort of like Dan and I’s, like primary audience of analysts.
[00:18:12] Bhav: Like if you are a data scientist and you want to get into data science, Eagle actually funny enough has a YouTube channel where you can do it. what we want to talk about today is people who are working in the data analytics space and, Not maybe they haven’t quite harnessed the skill sets and the tools and, you know, the parts of the job that they need to do, in a traditional analyst role, just because they haven’t exposed to it.
[00:18:36] Bhav: What are the kind of like the core skills you think an analyst should be able to do, that they can kind of like tap in from, like, from the data science space and the data science world?
[00:18:45] Dan: Can I, can I, can I jump in before we, we, we do anything else and just, just be like, Back even one step, let’s define data science and analyst, right?
[00:18:55] Dan: Let’s let’s define those, but yeah. Yeah. So where’s the overlap? What’s the differences. And then, and then I think we could do that because I mean, I, for one, I’m really curious and I think we all have a slightly sort of different, unique perspective on this anyway, Egor over to you, what, what is a data scientist? And what is an analyst?
[00:19:10] Egor: Right. So I’ve done a video on this in the past and it does vary between companies, but if you want, if you want what I think it should be and what I think it is in most companies, data analyst is purely analytical and like prescriptive statistics. or I think the difference between a data scientist and a data analyst mainly is the predictive models you’ll build.
[00:19:27] Egor: So data scientists normally do more predictive modeling, predict, predict statistics, building statistical models, building machine learning models. That is kind of the main difference you will find. So they kind of have, I say this like, you know, not to offend anyone, they typically have better mathematical skills, like understanding of calculus, probably a bit better statistics.
[00:19:46] Egor: In general, you kind of need a bit more knowledge to be a data scientist than a data analyst. There is an overlap, of course, but I think the main difference is that a model building and more of the predictive statistics. That’s the main difference between the two roles.
[00:19:59] Bhav: So now going back to my question then, well, actually no, in that case, what is the difference between a machine learning engineer and the data scientist?
[00:20:06] Bhav: Because that’s where you kind of have two titles, based on like what you said at the start of this call. So how do you, what’s the, what is the distinguish? distinguishing factor between those two job titles nowadays, because I think like four years ago, Forbes claimed that data science was the sexiest job in the world, but they hadn’t really, at that time, the concept of machine learning engineering wasn’t as mainstream as it is now.
[00:20:26] Bhav: And everything was like dates. People were just changing that. They were analysts, but they were just changing the job to data scientists and asking for 20 grand more in their pay package.
[00:20:35] Egor: Yeah it’s quite interesting. So it’s a transition as, as, as an answer in two ways. It’s kind of a nuanced question.
[00:20:41] Egor: So data scientists, you do. Kind of more of the modeling building side. Most data scientists don’t know how to deploy production code. If you ask a lot of data scientists I’ve met, they don’t really understand how to, you know, write production code on unit testing, CI, CD, the point AWS. A lot of it is.
[00:21:00] Egor: It’s changing now, but a lot of it was like notebook based and a kind of like offline modeling machine learning engineer is kind of a mixture of software engineer and a data scientist where what will happen is like, say you build a model, you will then deploy it yourself on say AWS, GCP, whatever kind of, cloud server, but then you’re basically delivering value directly because your model will be actually in production, making live decisions.
[00:21:23] Egor: So machine learning engineer understands how to bring a machine learning model. Into live, you know, live data, so live predictions now where it’s kind of fades in that in my previous world, previous Augusto, I was kind of a machine and engineering in my titles data science and that I’ll build the models and I also deploy them, which will machine engine engineer does in my head at the moment what’s happening is that data science is going into two different pillars.
[00:21:49] Egor: You’re either more of an analytical experimentation data scientist, like product analytics, running A B tests, doing more of a statistical analysis, or you’re more of a machine learning engineer data scientist, where you’re building models and also deploying into production with the help of software engineers, things like that.
[00:22:04] Egor: So I think data scientists is now pivoting into those positions. Obviously it varies, but that’s kind of where I see it going, particularly at Gusto, that’s what happens, or at least in my role at Gusto, that’s what was going on. And like, where I’m now at Deliveroo, machine learning engineer is very much more deploying and coding models, and data scientist, from what I know, I haven’t started yet.
[00:22:25] Egor: It’s more like experimentation, stuff like that. So there’s kind of a split between those roles now.
[00:22:31] Bhav: So one of my good friends, Sean McGill, Dan, you remember Sean from one of the crap talks events where he, where he gave a really fun talk called, data science, versus data religion. And in that talk, I know it’s, it was not about the talk really, but he’s always says to me like, cause I, I refuse to call myself a data science based on the latter definition of what that.
[00:22:51] Bhav: Machine. And I always considered, I was like, no, I’m not a scientist. I don’t build predictive models and put them into production. I do more of the statistical analysis of experiments. I can build forecasts and I can do like build, I can do regression. And I was like, I can do all of that. Kind of like the stats kind of side of things, but I refuse to call myself a data scientist.
[00:23:09] Bhav: But this was like three years ago or four years ago. Now I’m kind of kicking myself because I wish I had just called myself a data scientist. I would have been earning way more money.
[00:23:18] Dan: So I was going to say, but it’s now, you can argue that at any point in time. I mean, a lot of the machine learning engineers, they’re now AI engineers.
[00:23:26] Dan: You know what I mean? Like you can retitle stuff to make it sexy for the moment in time to capitalize on this kind of budgets that are available. But I mean, it still doesn’t change the brass tacks of what we’re doing and the approaches we’re using. I mean, a lot of the tools might change over time, but a lot of them are consistent and the methodologies are pretty, pretty similar, right?
[00:23:42] Dan: I mean, again, things change all the time, but you know, I, an AI engineer today and a machine learning architect from four years ago are probably doing a similar role.
[00:23:51] Bhav: Well, actually, it’s funny you say that. I think what’s happened, certainly I’ve observed like three, four years ago, people were hiring data scientists and they realized what they really need or what data engineers.
[00:24:00] Bhav: So, because what you had with you, you had these people, the data science, job titles, but they are having to build data warehouses and data pipelines and maintain the date, the code. And before they could even do the data science side of things, so we’ve, we’ve, we’ve established some things we’ve established what machine learning engineers we’ve established that data science.
[00:24:20] Bhav: Is a gray area at the moment in time because it could mean either closer to the analyst side or could mean closer to the engineering side. and then we obviously, we know what analysts is because that’s what me and Dan do day to day. And we have done so for longer than we can remember. So for any analysts, That are listening and potentially having that kind of like, you know, you, equal.
[00:24:41] Bhav: I loved about what you said was actually, you tried to talk about the human aspects of your role. there are a lot of analysts who are out there probably thinking they’ve got imposter, imposter syndrome, because there’s these data scientists who are coming through and they’ve got all these fancy skills and fancy qualifications.
[00:24:56] Bhav: And I don’t know what else they have that’s fancy, but. They’re probably feeling like there is a skills gap that’s missing, but you and I and Dan, we’ve just discussed that maybe there isn’t a skills gap, certainly in what was previously perceived to be a data scientist. So, so now what would you say an analyst needs to do to bridge that gap and become more data science in that kind of like experimentation and testing world?
[00:25:23] Egor: Yeah, so I would definitely learn some sort of, not necessarily machine learning, but more like predictive statistics, so any regression, gamma regression, all these regression models, polynomial regression, logistic regression, understanding how you do Bayesian regression analysis is very key. I think a lot of machine learning, I hate the word machine learning because it’s not really machine learning, and I hate the word AI because AI is just machine learning and machine learning is just statistics.
[00:25:45] Egor: You know, you could digress it back. Well, the point is, I think you learn more of like the heavy statistics and particularly the regression analysis part. Like understand how to build basic models, understand more about how you build predictive things. Once you can do that, then you can do like basic machine learning.
[00:26:00] Egor: Basic machine learning is really simple. I mean, I’ve had analysts at Gusto I worked with who basically came up to scratch of like, trees really quickly because it’s just, you know, once you understand the theory behind it, the package just don’t do a lot of it. It’s more the how you build the models. So I give you a really simple example.
[00:26:17] Egor: When I was working in insurance, Most people, you know, say I should build a model to predict claim, severity, which means like the cost of a claim. Now, most people would just build, you know, linear regression model. Now there’s a trick there because a claim can never be negative. It can be zero.
[00:26:33] Egor: Whereas linear regression assumes that it’s normally distributed and all distribution can be negative. So that’s when you need that knowledge of like, okay. I need a gamma model or gamma target because that could be non negative. It’s things like this which are more like you need to understand the intuition behind building predictive models and you only really get that from studying statistics and not necessarily machine learning to start with.
[00:26:52] Egor: So if you’re a data analyst, focus on just purely statistical predictive models. And that would be the bedrock for everything else you do. That is actually the main thing I think most people in the data field should also study as well.
[00:27:04] Bhav: So, I really like this, and it’s nice to kind of go, like, because sometimes, you know, we talk to our guests and the topics go a bit abstract.
[00:27:11] Bhav: So, it’s nice to have a, be talking about something that’s a bit real and understand sort of like the, like the use case. So, we’re going to talk about this. Again, for someone and someone who’s listening, say, okay, cool, Egor, I understand what you’re saying. I need to do some, I need to learn something about linear regression models and gamma regressions and things like that.
[00:27:27] Bhav: But what, what am I doing then? Like when might I use that? So what would be the advice that you would give in terms of a practical sense? Because yes, I could read where I find the way that a lot of people teach is there’s theory and there’s application and. Never shall the two meet in the professional sense for some reason.
[00:27:46] Bhav: So how do you bridge that gap between the theory of statistics? Cause I understand the use case of linear regression model, right? And try, you know, someone who has never come across it. I did, and I did my undergraduate degree in math and I dropped stats, not realizing that would have, that’s going to be the biggest problem.
[00:28:00] Bhav: I hated stats when I was doing my undergraduate math degree. but now I’m kind of like, shit, I wish I hadn’t dropped and I wish I’d taken it into year three. So. The application, how do you, where do people go to, to understand when they should use a regression model, when they should use a gamma ray, like that application aspect I feel is missing.
[00:28:21] Egor: It’s a hard one because. If you want my, this is my experience, right? So theory, I like theory a lot because it’s perfect. Theory works, theory works because it’s based on mathematical axioms. It doesn’t break, it’s purely perfect. You know, statistics, state spaces, wherever you want, all that works perfectly because it’s theoretical.
[00:28:40] Egor: And it’s based on, you know, fundamental axioms of maths. In practice, nothing’s that perfect. So, how do you go about applying it in practice? It’s basically just experience and trial and error. Like, not trial and error in the same thing, but like that example I gave you about, let’s say we have a claim.
[00:28:57] Egor: The claim can model as a normal, normal linear regression, gamma regression, but you can also model as a plus one, plus one, so plus one, plus one regression. the point is neither is better because the problem is not so perfect. You can do it. So how do you go applying to real life? That’s like, that’s that, that’s that any job, right?
[00:29:17] Egor: That’s a, that’s a, you find, you’ve got to find the source, right? That’s, that’s where you get your money from and understanding these principles and what the best ones are to apply them. you only get it for experience. So. The best way I would suggest it is one, build loads of things, like build loads of things for every single problem you have, just, just try out so many models, build a model using, you know, gradient bootstrap tree, regression, random forest, neural network if you have to, and through experience and practice, you’ll get intuition.
[00:29:44] Egor: I think, You know, people think it’s very formulaic, but there is an art to modeling, like modeling everything. There really is an art to it, and I’ve seen it happen before. I’ve worked with people who are like, they can see things that I don’t see. And it’s not necessarily that they’ve kind of, this is what, this is exactly the process you follow.
[00:30:02] Egor: They just have that intuition. And like I said, you only get that for applying. Those are things. And that’s when you, that’s when that statistics knowledge would naturally just come out and you visualize it. I know it sounds really philosophical at a high level, but this is just how, what I’ve seen and you can’t rush that process. It’s just intuition. You develop through time.
[00:30:21] Bhav: So, because I think what you talked about there, you’ve introduced a third potentially risk Okay. factor that people aren’t going to be fully aware of, which is when, when it stops being the perfect theoretical model and you start entering into more real life situations and you are trying to find the perfect model, you run the risk of overfitting, right?
[00:30:42] Bhav: And you, and you might not know when to stop. So, you know, you’ve got your, let’s say you’re targeting some R squared value and, you know, you’ve hit like 60, 70%, 0. You probably should stop, but you keep going, you keep going, you keep going, and you might try something else. And then you start realizing that you’ve got some type of like multicollinearity happening and then you’re not really aware of it.
[00:31:03] Bhav: So again, these kinds of things are like, people aren’t there to teach them. Right. So what’s your advice in those situations? Because I 100 percent agree with you. You have to trust the process. Right. You have to trust the process. You have to build, you have to build, you have to build. But there comes a point when you don’t even recognize the mistakes you might potentially be making because of the imperfect world we’re living in.
[00:31:25] Egor: Yeah. And that’s, it’s a hard question because like, what point do you stop? I think there’s a saying from, it’s a George box. It was like, all models are terrible, but some are better than others. So you’ll never get a perfect model. So don’t chase a perfect model. It’s impossible. as much as possible, chase the 80 20.
[00:31:43] Egor: that’s what I’d say. At the end of the day, if you’re working for a business like I do, All you care about is your model, is your model generating profit, or is it being financially suitable for the business? That is kind of your holy grail. And that’s when, that’s when that kind of theory, non profit models kind of transcend because it doesn’t really matter how you build your model necessarily, as long as it’s profitable for the business. And that’s all that matters realistically. And that’s what I try and do.
[00:32:11] Dan: This, this is another favorite topic of mine and Babs, which is talking about the measurability or the kind of return on investment in these kinds of things. So, so let’s say you’ve built a model, you’ve, you’ve fitted it as best as you can, or where you’re happy to stop.
[00:32:24] Dan: And you’re saying about making revenue or making money for the company or being valuable in this way. How do you. How do you ensure that next step happens? Because once you’ve built your bit, you’re relying on other people to make that into some money or some value, right? And so what is your approach to making sure that that has succeeded, that you have made the business money and it has been valuable?
[00:32:45] Egor: You mean in terms of how it’s like deployed or how do you ensure your model is financially viable?
[00:32:51] Bhav: I think that latter one, because I was going to literally jump in and say, how do you know if you’re like, I know there’s an element of testing that you’d have to do, but how would you test that actually the model you’ve built is working?
[00:33:00] Bhav: Cause let’s, let’s say you have amazing stakeholders who just trust you. They’re like, yes, you go, we’ll do whatever you say. Like we are not worthy. Right. But they don’t know. They’re not comparing it against some, you know, some control group. So how do you know in that case that it’s work? How do they know it’s working? How can you be reassured? It’s a good question.
[00:33:21] Egor: It really depends on the model. The ones works for me well in the past is backtesting. So i.e. you have an old model, new model. Then you backtest this. In other words, you run the old model with all the pre all the information that a new model would have run.
[00:33:34] Egor: So in other words, you timestamp it. It’s like, if it was like, what are we now? 25th of January. If my model ran on 22nd the results be compared to the old model? So basically you have your new model and you’re backtesting all the previous results. So you see if it’s actually better than a new model on the same input data historically.
[00:33:51] Egor: That’s how I do it. That’s an offline test. Then the other way of doing it is that when you deploy it into production, you run it in a shadow mode. So in other words, it’s live, it’s making predictions, but it’s not actually making decisions. But you can compare the predictions and you can see it’s better.
[00:34:05] Egor: These are like really good machine learning engineering principles of testing models, both in production and offline, and eventually you launch it live.
[00:34:13] Bhav: So this is, I feel like I’m learning some new stuff.
[00:34:16] Dan: Yeah, I love this, but I want to go deeper on this because if I’ve got, if I’ve got a predictive model that can, and I’m running it in shallow mode and I can accurately predict the lottery results every single time, it’s still not valuable unless I go out and buy a ticket and I’m not the one doing that.
[00:34:32] Dan: Right. So, so what about that next step within the business of saying, okay, I’ve got this amazing. predictive model, who’s going to go out and buy the lottery ticket, who’s going to activate that and use it to make change to be valuable to the business. Otherwise we have a clever machine, but it’s not sort of the next part hasn’t been plumbed in yet.
[00:34:49] Egor: I see. Well, it is really depends on your infrastructure. Like let’s say for my assumption, you already had a model in place to decisions. So let’s say, So let’s say you had a, you had a algorithm that decides the recipes and you find another algorithm that decides the recipes and that algorithm is better, you can directly compare it.
[00:35:07] Egor: what you’re describing there is very different because it’s kind of, if I go to the, well, I can go to the details. There’s two things here. One is like customer driven behavior algorithms are very hard to measure because let’s say I have a recommendation system for two things. It’s really hard to know if my new recommendation system is better than the old one, unless you do a 50 50 A B test, you can’t run it purely in shadow.
[00:35:30] Egor: That’s one way of doing it. So if you want to know if it’s better, then that’s where you run an A B test. For example, when I worked in forecasting, like forecasting stocks, That’s quite easy because the forecast, it doesn’t affect anything. And you can immediately see the forecast is better because it’s not influenced by customer behavior.
[00:35:46] Egor: So like I said, there’s an art to everything. There’s an art to this and really testing it. So in your scenario, I would do an 80, 20 split test, anything that’s like, You want to do a test, and again, this is when it comes to really business specific industries, or very specific to the company. You want to do a test that really minimally affects the customers, but gives you enough signal that you know your algorithm is indeed bringing value if the customers are involved.
[00:36:10] Dan: I suppose that’s when it goes over to someone like you, Bhav, right? Into the experimentation and the analytics side, and saying, you know, Or, or, you know, pumping that into the marketing campaigns with bid adjustments or whatever, but that’s the, that’s the, that’s the testing and that’s the hard thing to measure, like you’re saying, Egor, but like, you know, you can have an objectively sexier or better model, but actually putting it into practice and then run the test and see if it actually works.
[00:36:34] Dan: Right. I mean, that’s the thing. Does it change hearts and minds or does it not? If you’re doing a recommendation engine, for example.
[00:36:40] Bhav: Well, I think in this instance, actually, yeah, so you’re right. I mean, this is where we would step in. So as. listeners will know I used to work at Gusto and I looked after a lot of the AB testing that we had, there and I, and I don’t know who looks after now, but the processes are very much, you know, we would work with the product team to run AB tests.
[00:36:58] Bhav: And we, you know, we, we, and we actually AB tested the, the, the recommendation algorithm. We tested, we tested the routing algorithm in the factory. And so, you know, it’s kind of fun to have this. Cross discipline way of working where you’ve got the, the data science team who are building these amazing models and then, then sort of like my team would come in and work with the product teams to kind of test those models, understand the outcomes, do the analysis on them and make, and so, you know, actually has it worked, hasn’t it worked, et cetera, et cetera, et cetera.
[00:37:25] Bhav: So I guess going back to kind of the theme of what we’ve been talking about, which is that, An analyst who is potentially looking to kind of like supercharge their skills, because I think, I don’t know if anyone saw the most recent report from the world economic forum, the top skill for the next five years is going to be analytical thinking.
[00:37:42] Bhav: So I think there are going to be more and more people in the world who are probably looking towards more data science type skills, analytic skills, critical thinking skills, and it’s these type of. It’s this type of decision making this, this, this thinking, this kind of like basic statistical analysis is needed to be able to even do the bare minimum at the moment, I would say in, in most of these organizations.
[00:38:04] Bhav: So you go, I have a question for you that, it’s, it’s somewhat controversial, not for you, but for people who might be listening is. We have you, you’re familiar with the AB testing world, right? How much of that is, do you think should be science? And how much of that do you think should be odd? Because I know a lot of people who work in this space who actually don’t have a background in statistics, but they are running AB tests all the time, day in and day out.
[00:38:31] Bhav: Like, and I’ve had this debate for a long time for me, actually, I won’t influence just go. I’d love to hear your thoughts.
[00:38:39] Egor: It’s a good question. I mean, like, if we go back to an AB test really is doing, it’s like statistical hypothesis tests, right? Ultimately, And that’s like, yes, it’s, it’s, you know, I don’t, who was it?
[00:38:50] Egor: I forgot who did it. Was it a guy who did the plant? No, that’s someone else. Anyway, it doesn’t matter. it was purely mathematical. and it was, it’s, but at the end of the day, it’s pretty mathematical, but it’s still statistics, right? You pick an arbitrary significance level, right? 10%, 5%, 1%. Why, why do you pick that?
[00:39:08] Egor: That’s again, that’s an art to that. That’s intuition. You don’t pick it because it’s right. You pick a certain distribution, you pick a certain sample size, you know, there’s numbers to go to back that up. I’m sure there’s like, this is getting out of depth of my knowledge, I’m sure there’s initial derivations explaining exactly why certain sample sizes, certain percentages are better for certain cases.
[00:39:27] Egor: Like there may be some sort of mathematical reason for it, at least I hope. the question of how much is that art? Yeah, it’s, it’s tricky, right? Because I feel like all the examples you get from hypothesis tests are very concrete. Like, you know, the average height of the U S is this. I’ll take an average sample of California’s height is this, how much of this is an outlier?
[00:39:49] Egor: You know how, you know, can I, can I confirm certainty that California is an average higher than the rest of America? You know, that is a very like one dimensional problem. Yes. You could do two tail tests. You need two dimensions. When it comes to testing things live, like we do, there’s so many factors in that.
[00:40:03] Egor: That’s so difficult to understand, right? Because let’s say Augusta, you launch a menu and you give half a minute to some customers, half a minute to other customers. It is a fair test, but just think of all the different, you know, things that can be happening there, you know, really control the customers, you know, you know, really in control when they log in.
[00:40:19] Egor: There’s so many other variables that are very hard to measure. So it’s very, it depends. That’s everything. but I do think there’s an art of it. Yeah. And I think there is an art to everything. And like I said, I think also statistics is one way of viewing things as well. It’s on the, it’s not necessarily the only way.
[00:40:38] Egor: Sometimes common sense just plays a role in it. So, you know, let’s say you find a conversion rate of 60 percent for a certain group and 40 percent for another group, but let’s say that result is not statistically significant. Well, there’s quite a big gap. So I know we’re speaking in arbitrary terms here, but just because the numbers tell you something does not mean that’s actually what’s happening.
[00:40:56] Egor: You know, so if you’ve heard of, you know, is a Simpsons paradox or Simpson, you know, is that I kind of equate to that or something similar because it’s a bit more like, yes, there’s a correlation there. It’s a causation. There’s something else going on here. It’s so multifaceted. So I would say, yes, you can say it, but pure numbers.
[00:41:15] Egor: But taking everything by pure numbers doesn’t always work. So I would say you need some common sense as well. Don’t rely on purely the numbers on any decision you’re making in like a business setting.
[00:41:24] Bhav: You need that foundational knowledge, right? To be able to then make the trade offs, right? So if you’re, if you’re, if you’re going to introduce, common sense, you’re going to need some type of baseline, because I think a lot of people view A B testing as, you know, the ideation side of things, the hypothesis side of things, right?
[00:41:40] Bhav: And there is, and I, whilst I agree, there is an art in doing user research, finding problems. Doing things like assumption maps and problem statements and all of these types of things that go with, like, you know, like, like anything, like what, there’s a million things we should do. What should we do? Right.
[00:41:54] Bhav: There is that aspect of it, but then there is that kind of like other side of the coin, which is very much how reliable is the thing we’ve just done. And that is the thing. Like, and it’s, you know, I, we talked about the differences in the term data scientists. And I know I deliver, even at train line, you have product data scientists who focus more on the experimentation.
[00:42:13] Bhav: Experimentation side of things. so, okay. You mentioned something that I have, I’m going to dig in to a little bit more just because we have a bit of time. You mentioned that just because the numbers say something doesn’t mean it’s true. Right. Imagine I’m a business stakeholder and you say that to me, I’m going to turn around and she’d say, what the fuck you go, right?
[00:42:29] Bhav: I came to you for an answer and you’re now telling me that, Hey, look, just because I said it, Doesn’t mean it’s true, right? What do you do in those situations? Because you’re right in that in that recommendations Example where you’ve you know, you’ve done 50 one recipe one menu 50 the other there are a whole bunch of factors I could have Played a role in it. but you need to make a decision at the end of the day, which one do we roll out? And if there are these additional factors, what are the criteria? Like how much deeper should you go outside of just the P value for when you’re doing this, these analysis?
[00:43:04] Egor: Yeah, this is the thing. And this is, this is where people get paid the big box, right? This is where you make the money because this is where you need that intuition and both sides of the camp. You need a business, you need industry knowledge and you need the pure figures. and this is why businesses ought to do well, not because. These decisions are quite crucial. how you go about it.
[00:43:24] Egor: I don’t know that to be honest, it’s, it’s a, it’s again, every situation is so different. You have to really understand your business very well and understand what your customers are doing and how they behave. I think it transcends purely data science at that point. And you have to really take into account every single thing that your business is doing to make a certain decision.
[00:43:43] Egor: I know the answer is very vague. It’s just so like crucial to everything. I can’t, I don’t want to say follow this criteria. because I don’t think there is a criteria. I think every business is so different and every situation is so different.
[00:43:57] Dan: I get that. I did a, I was asked to come and talk to a bunch of recent graduates around like, you know, careers into data and giving some advice.
[00:44:06] Dan: And it was really interesting, like speaking to people that are like, Oh, what, what language should I use? What, what tech should I learn? And I was like, well, Well, maybe you should start by thinking about the industry or the, the vertical you want to go into, like, do you have a passion for travel or shopping or, insurance because, you know, being a, in the world of data, whether you’re, you know, anything from tracking to analysts to data science, if I, if I’m doing that in finance, that’s a different kettle of fish to econ, to, marketing, you know, so I, I, my advice to them was always pick that.
[00:44:37] Dan: And I think this is kind of echoes what you’re saying here, which is like. Okay. You know, the, the data says this, but you have to have industry or even specific business experience to understand the application of that and what the gears and levers you have to pull. I am, I very much found out the hard way when I was a very new into the world of analytics.
[00:44:55] Dan: and I was doing some attribution modeling and I, and I looked at the kind of the cross channel mix and I was just like, Oh yeah, you should, you should do more email. You should spend more on email. And I was just like, Oh, and I was just like, I can’t spend more on email. And I was just like, Oh, and I didn’t realize at the time I had to learn.
[00:45:09] Dan: I was just like, what am I even saying? You have to be aware of the recommendations you’re giving if it’s even possible so that you know, not to make a fool of yourself. Like I did.
[00:45:17] Egor: Now, as I say, it’s quite interesting what you’re saying there, because I’ve said it before in a few videos where I’ve pivoted from technology to solution to, you know, solution technology, as in like, how do I solve this problem first?
[00:45:28] Egor: And then do it at the end of the day, it doesn’t really matter what you do. As long as you bring money to the business, I know that’s very reductive. As long as you bring money to the business, you bring money to the business, no one cares how you’ve done it. As long as you do it, obviously there’s techniques in place and it’s processes, but your job is to find a solution, no matter what the solution is.
[00:45:45] Egor: No one really, to an extent, no one cares that much building neural network or you build a linear regression model. It doesn’t matter. As long as the money’s on the table, you’ve done it. That’s all I really meant to say at the end of the day.
[00:45:55] Bhav: Yeah. And I think that debate of, should I learn Python? Should I learn R is just for people putting off actually doing any type of learning altogether, just pick a lane.
[00:46:03] Egor: I mean, I still have an opinion on that. I think you should learn Python, I don’t think you should learn R.
[00:46:06] Dan: Okay. Well, let’s, okay. I want to, I want to jump straight into the, the rapid fire questions and then we can, we can pivot and we can wrap things up slightly separately because, my, my first rapid fire question that I have for you, Egor is, if you could only, if you could only. Do one programming language or coding language. What would you pick?
[00:46:26] Egor: It depends on the question. If I was going to be a machine learning engineer, I don’t know, if I had to only code in one language for the rest of my life, it would be Python. If I didn’t want to be a data scientist, I could be anything else, right? I could be a back end engineer as well. Could be front end. I could do loads of stuff with Python.
[00:46:42] Dan: Second question, Egor. if someone asks you, where should I go to learn about data science? What’s the, what’s the one sort of location or place you would send them to?
[00:46:50] Egor: Can I say the internet.
[00:46:53] Dan: The internet. I feel like that’s a bit of a cop out.
[00:46:55] Egor: Okay. Let’s assume it’s all right. I guess it’s so specific. I would say I really liked blogs, like there’s paid, I have used paid resources in the past, but everything paid can be found out free online, but pros and cons to everything, right? I always say go for blogs because it’s free.
[00:47:14] Bhav: You don’t know any good data science YouTube channels do you?
[00:47:17] Egor: Yeah, but mine aren’t technical stuff. Some of them are. So yeah.
[00:47:22] Dan: well, I would love to see how the YouTube channel evolves, like you said, is as you progress in your career, I think that the tech, the subject matter will naturally become more technical and deep. Right. That’s the, the. last question. We ask every single guest we’ve ever had on, what do you do outside the world of work to wind down?
[00:47:36] Dan: What do you do when you’re not data sciencing?
[00:47:40] Egor: Quite boring actually. I do a lot of sport. I play hockey three times a week. I run, I’m not that no, but people will say like, I kind of, I don’t, I don’t like, I don’t really watch, I don’t really watch Netflix. I don’t really watch TV shows.
[00:47:55] Egor: I watch YouTube because I’m on the platform. I read, I need to try to read a lot. It’s mainly just sport reading and that’s it. I don’t really have anything else that I do that frequently. I would say.
[00:48:10] Bhav: I think it’s a generational thing. Like Egor, I think you might be the youngest guest we’ve had on the show. just the fact that you kind of like always on YouTube, like it’s, this is such a, like a generation thing. there is one question and, and actually this. This kind of like brings me onto a point. I know Dan, sorry, we normally stop after rapid fire, but there is one question I’ve been dying to ask that I’ve been holding off.
[00:48:30] Bhav: You mentioned, obviously you want to try and move away from LinkedIn, right? Because it’s, for whatever reason, I can’t remember what you said, but you want to move away from LinkedIn. I read about this, this lady recently who was a data scientist and she switched from, she switched off from doing PhD into moving to Onlyfans. I don’t know if you heard about this one.
[00:48:48] Egor: No, no, no.
[00:48:49] Bhav: She, okay. She was doing a PhD and she was doing all these like YouTube videos and data science videos. And she stopped her PhD. Now she only does data science videos on only fans and p hub. Like, are we going to see you on those platforms anytime?
[00:49:05] Egor: I don’t, I don’t think, I don’t think I’m the type of content grades that they want on those platforms. no.
[00:49:10] Dan: I think the monetization game is strong. I mean, this article, well, this is, it. Read this, I read the same thing. And this person had, I think those platforms versus YouTube, and it was like, the revenue difference was incredible.
[00:49:20] Dan: I’ve seen this. Yeah. and it was the same content, the same video. There was no difference.
[00:49:24] Egor: Oh, really? It’s like I, I’ll upload my actual YouTube videos. I think it’s worth a go.
[00:49:29] Dan: It’s worth a go.
[00:49:31] Egor: I would say one thing, and I say this to, I don’t know if anyone watching this. I would, I say it’s, I say it’s every single video. I always recommend for anyone to start making content on anything they do in data science or tech, anyone who watches this, they probably won’t do it, but I always say it’s such a game changer for your life and career. It’s unbelievable. Like people say, Oh no, just do it. I know people always say like, Oh, I, you know, I don’t think there’s a bit of bigger, like multiplier in my career, professional life.
[00:50:01] Egor: and probably personal life then. content. and also like there is money in it. It just takes time. You know, if you choose like a business, it’ll build slowly, but you know, people expect business, you know, most businesses don’t do well in the first few years. It’s pretty normal. So it’s also the best way of learning.
[00:50:19] Dan: Like you learn by teaching, right? Yeah.
[00:50:22] Bhav: By teaching we learn is a, I think there’s a, there’s a Latin version of that, but I think the officially translates to by teaching we learn. And I I’ve been preaching this for years, Egor. So Dan and I, obviously we have our own different ways of doing, content.
[00:50:35] Bhav: Obviously the, the, the podcast is where we cross over, but I have been preaching for years to people. To create content to write more writing, obviously helps you articulate your thoughts and structure your thoughts and like really understand what you’re doing. And then things like video content. And I don’t, I don’t do video content.
[00:50:52] Bhav: I won’t lie. This is something I’ve been putting off just from pure fear of having it out there forever.
[00:50:58] Dan: Well, I also, I also, have not done video content. However, as of the time of recording about a couple of days ago, I did a, I’ve got a Google analytics for training course and I recorded 80 eight to zero videos, all between one and eight minutes long.
[00:51:17] Dan: and they, Are now all on YouTube. So if you head over to measure labs, YouTube channel, it has just been flooded with pictures of my face and talking about Google analytics for, and loads of other things. So I would say I don’t really create, you know, video content, but now there’s almost a hundred videos of me talking about stuff on YouTube, so there is that.
[00:51:34] Bhav: Right you both have inspired me. One of my objectives over the next couple of weeks to produce was to produce a handful of short bite sized videos for my team internally around proper data analytical, analytics problems that they can think about and solve. Like, you know, as you said, you learn by doing it.
[00:51:49] Bhav: so this was kind of like that, so I’m going to do it. I’ve been putting off and I won’t lie. I was, this was an objective I was going to fail on.
[00:51:55] Dan: Egor, we’ve got, I would ask the normal question of where can people find you if they want to connect with you, but I think we’re going to be linking out everything, your YouTube channel, your newsletter, anything. But if you could ask them to do one thing, what would it be? Where would they go? Would it be YouTube? newsletter?
[00:52:11] Egor: Go to YouTube. YouTube’s probably the best place to go.
[00:52:14] Dan: Awesome. And that you can come in and chat to you, you go on those videos. and of course, every link that we’ve discussed will be in the show notes.
[00:52:22] Dan: I always try to make a good note of all the things we discussed. and I will put your LinkedIn profile in there. Thank you so much, Egor. But, is there any last pearls of wisdom or advice that you want to give our listeners before we wrap up for today?
[00:52:35] Egor: Yeah, I kind of said it earlier in the, in the podcast, but I’ll say it again in that. I always say this to everyone, I mentor people, I always tell them this, is that just be patient, take your time, and eventually you’ll get there. And it’s just putting in the effort, and everyone always says it, put in the effort consistently over time, and eventually you’ll get there. Don’t be disheartened if you don’t get your first job in three months, six months, even a year, just take patience, follow.
[00:52:59] Egor: It’s like, I don’t know if they trust the process, but it is generally true. Just as long as you put in the effort and you play the odds, you will get your first job and you’ll do well in your career over the long term. That’s all there is in pretty much everything in life, right? Just time, patience and effort.
[00:53:14] Dan: I love it. Well, thank you so much. That’s it for this week. Thank you for listening. We’ll be back soon with another episode of The Measure Pod. You can subscribe on whatever platform you’re listening to this on to make sure you never miss an episode. You can also leave us a review if you can on any of these platforms.
[00:53:28] Dan: We’re also over on YouTube if you want to see our lovely faces and our lovely guest faces while we do this as well. Make sure to subscribe to the Measurelab channel to make sure you never miss an episode as they come out. If you’ll leave us a review that would hugely appreciated. You can do that on most of the platforms the podcast applications, or there is a form in the show notes. You can leave feedback directly to me and Bhav. Thank you for listening and we’ll see you on the next one.
Subscribe to our newsletter:
Further reading
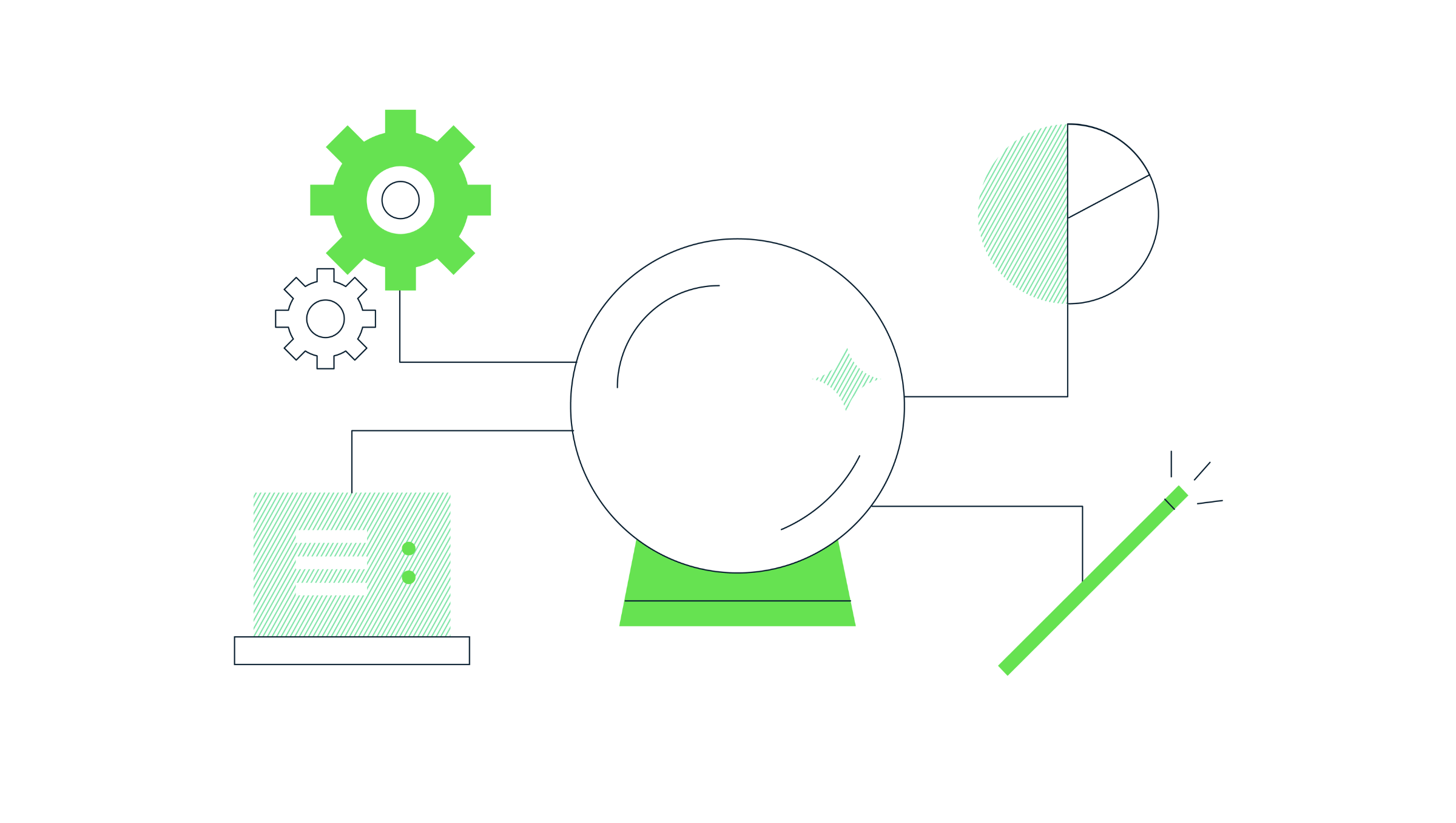
How to start forecasting in BigQuery with zero training
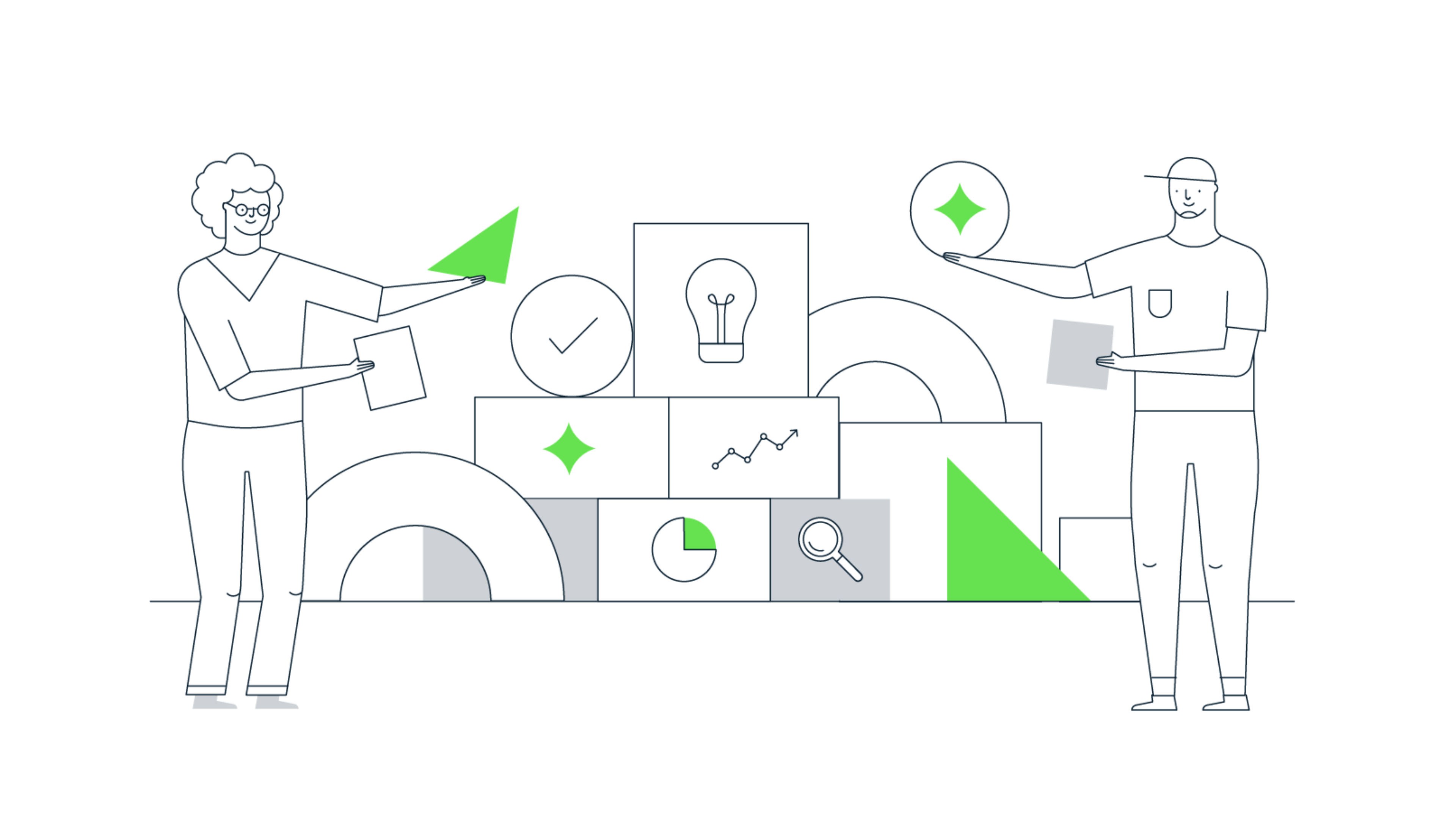
Easy ways to prepare your BigQuery warehouse for AI
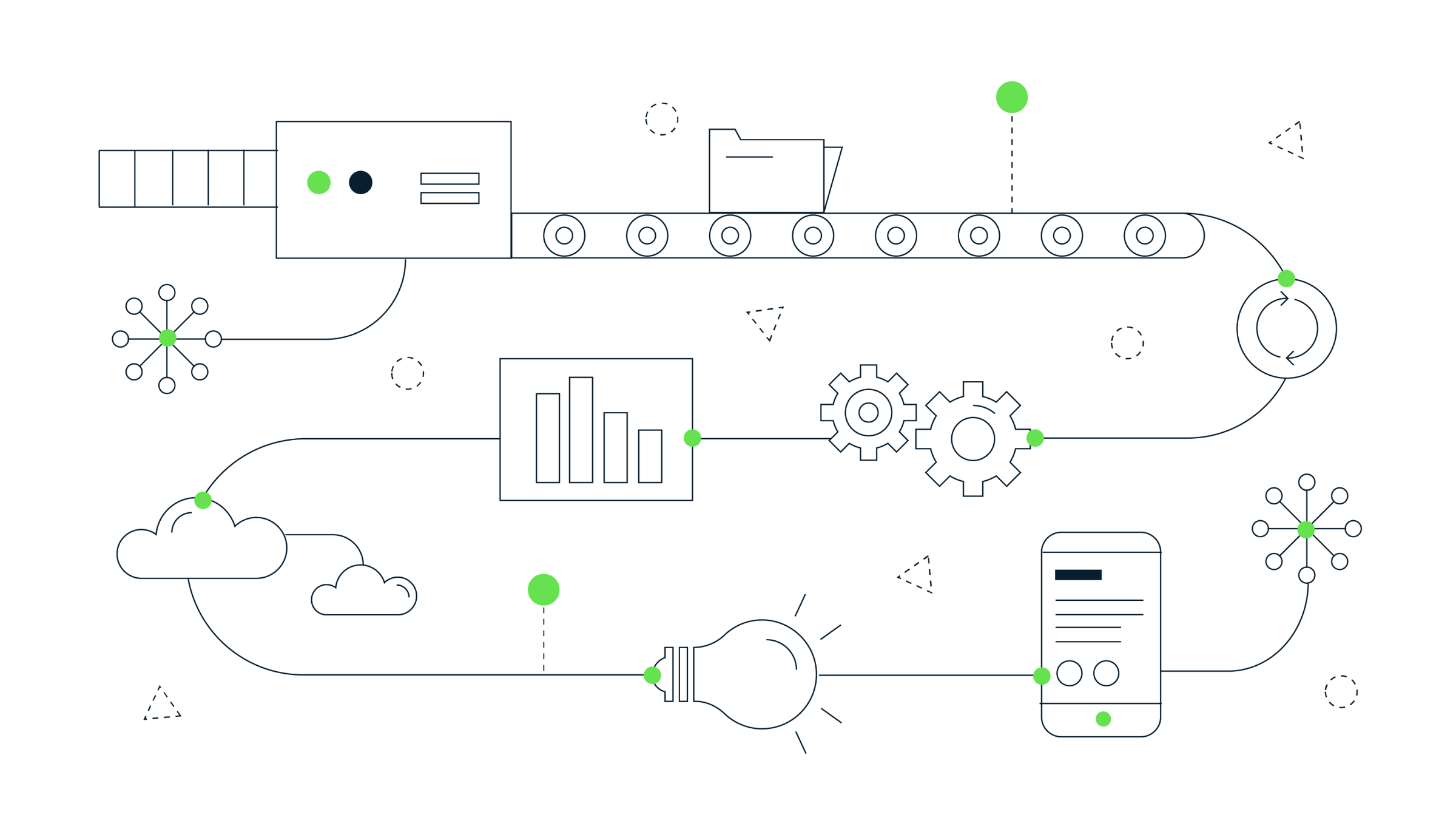