#11 Is this the end of real data in analytics?
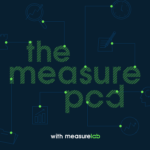
This week Dan and Dara discuss how the access to ‘real’ data in Google Analytics (UA and GA4) is becoming more limited due to technology and legislation changes. They think about what this means to people using analytics platforms like GA that are used to having access to all their data, and how this may change we use data in these platforms in the future.
The article from Google that inspired this is titled “How modeling technology enhances your analytics and prioritizes privacy” – https://bit.ly/3AkDJST.
The article from Google Ads announcing the move to DDA – https://bit.ly/3BkO0jh.
The GA4 help centre page on conversion modelling – https://bit.ly/3Afg4mx.
Dara refers to HyperLogLog++ as the methodology for UA to calculate Users, this is a page on the function in Standard SQL – https://bit.ly/3iBg8qQ.
Dan talks about GTM celebrating their 9th birthday, this is the original article releasing it to the world from Google back from 1st October 2012 – https://bit.ly/2Yoal0A.
In other news, Dan watches more TV and Dara visits the emerald isle!
Please leave a rating and review in the places one leaves ratings and reviews. If you want to join Dan and Dara on the podcast and talk about something in the analytics industry you have an opinion about (or just want to suggest a topic for them to chit-chat about), email them at hello@measurelab.co.uk or find them on LinkedIn and drop them a message.
Transcript
[00:00:17] Dara: Hello and welcome back to The Measure Pod. We’ve had a week off after hitting the big one zero on our episode count, but now we’re back with some more chit-chat, ramblings, bit of industry news, gossip, and maybe even the occasional guest if you’re lucky. So I’m Dara, MD at Measurelab. I’m joined as always by Measurelab’s longest serving Analytics Consultant, Dan. Hey Dan, what’s new in the analytics world.
[00:00:44] Dan: Hey Dara. Well, a couple of birthdays really. First of all, GTM had its ninth birthday on the 1st of October. So back on the first October 2012 is when it was introduced to the world. And nine years later here we are, still using it day-to-day which is crazy to think it’s been nine years. I remember it coming out. I don’t know about you Dara?
[00:01:01] Dara: I remember it like it was yesterday Dan. And so I’m going to steal your thunder. Eight years ago Measurelab was founded. So we had our eighth anniversary slash birthday at the end of September, so eight years of Measurelab.
[00:01:14] Dan: Wow, so eight years of Measurelab, nine years of GTM.
[00:01:17] Dara: How time flies.
[00:01:19] Dan: Well, in other news, we had two announcements from the world of Google. So server-side GTM is now officially out of beta. Which means that probably not a lot has changed, to be honest, maybe the beta flag has gone from the UI. But it means that it’s in a stable enough position or people are using it enough for it to be classed as a non-beta product from Google’s perspective. So, it’s exciting, it’s still early days with the product. I’m looking forward to getting more and more used to it, having more situations where we can suggest the use of server-side GTM. But it’s great to see that it’s moving forward full steam ahead. Even if it isn’t an industry standard just yet. The second one is that Google Ads have just announced that they are moving everything over to data-driven attribution as their attribution model of choice. So we’ll put some links to all these things in the show notes, for anyone to have a read through the official announcements, but a couple of things to bear in mind here is that. At the moment, there are thresholds, you have to be hit in a certain number of conversions per month for the data-driven attribution model to be available to use. And then they get rid of that. So there’s going to be a load of customers in Google Ads they’ll now be able to utilize data-driven attribution that have low volume conversions. Although a big question mark of how they’re going to be doing the modeling or the model is accuracy if they have low volumes of conversion. So there’s question marks floating around, but interesting to see how they’ve managed to do that once they roll it out.
[00:02:39] Dara: Is there going to be a choice? You said it’s going to be the new default. Is it going to be the only option or will you be able to switch it off, change to a different attribution model?
[00:02:48] Dan: You’ll always be able to go back. So you’ll always be able to select one of the other rules based models. You know, the last click, first click, linear, those kinds of things. But as of the. Well, as of now, really, as of the data for recording. So as of October 2021, they’re going to start moving it over for all new conversion types added to Google Ads, and next year they plan to have it for everything. However, again, there is always the option it starts with data-driven attribution, you can always undo that if you need to. Rather than starting with last ad click or whatever it uses at the moment.
[00:03:18] Dara: And this Is actually a nice lead in to this week’s topic, which is…
[00:03:24] Dan: Is this the end of real data in analytics? This is inspired by a post from Google they released a week or so ago, where they are basically moving all of their products over to machine learning models in some way or another. Whether that’s data-driven attribution from a conversion attribution perspective. Whether that’s filling in gaps with unconsented data, using things like consent mode in GTM or Gtag. It just seems to be a big push right now, so much so that they’re putting all their eggs in one basket as it were. And the thing that I have to question really is whether this is a good thing, a bad thing or something that just doesn’t matter and is going to happen whether we like it or not. But I think that’s the conversation today really is that, the more fuzzy data gets to people that have been around for a little while in this industry, Dara like you and me, where we’re quite used to having access to a hundred percent of the trackable data at very least. I know it’s always not a hundred percent. But for people like you and me Dara, we are so used to having all the data export it to BigQuery from Google Analytics, for example. Or creating audiences and segments on your customers based on the smallest segments of users when they go through a website in a very specific way. It just sounds like in the future, that’s not going to be a reality and we’re going to have to start adapting now. And Google are kind of forcing our hand a little bit, trying to help do that using machine learning.
[00:04:44] Dara: I think, yeah, the key point is that this is, this is happening like it or not. And it’s partly, or maybe more than partly in response to the fact that observed data is becoming less and less reliable, I suppose it’s probably the right word to use. So this is in a way Google’s response to the fact that there are an increasing amount of gaps in the data collection due to improvements really in privacy legislation and technology within browsers to control the data that’s being collected about you as a user.
[00:05:21] Dan: Yeah, exactly. There is less than less data collected in tools like Google Analytics now compared to where we were 3, 4, 5, 10 years ago. And you’re absolutely right, I think the big one there is around the technology catching up. So, well two fold, you’ve got technology catching up and legislation catching up, right. In terms of this wild west of the digital marketing and analytics world that we’ve been living in or playing in for the last 10, 15 years. So the first one is the tech, right? So every browser has quite specific rules around how they manage cookies and trackers in general. Most browsers, except for Chrome, now already block third party cookies. Chrome is removing access to third party cookies as of the middle of 2023. That will be officially the end of third party cookies. Something that irks me a little bit is people keep talking about the death of cookies. There’s loads of conversations right now around the death of cookies. Cookies aren’t dying, cookies aren’t going anywhere. What we mean by that is third party cookies, cookie set in a third party context. They will be a thing of the past probably when Google says so, in 2023. But with the technology around the browsers, even setting cookies in a first party context can be quite limiting. So in Safari is limited to either 24 hours or seven days depending on certain factors. In other browsers, you don’t even set any analytics cookies at all, there’s no tracking whatsoever. So there are issues around that that didn’t exist back in the day as it were. And the other side is the legislation. So we’ve got things like GDPR and the CCPA, but a hundred other things that are coming a hundred miles an hour at us and changing all the time. Which requires active opt-in consent, to be acknowledged by the user that you’re taking their data and doing something with it. So these two things in combination just means that the actual data you end up collecting in a platform like Google Analytics, regardless of what version you’re looking at, becomes less. There’ll be less users, not everyone will opt in to be tracked first of all. And when they do opt in to be tracked the data won’t be as necessarily accurate as it has been in the past. Where if a user comes back once a month, then they’ll probably be seen as a new user, regardless of what browser they’re using. Because the cookie just won’t be there to tie it all back together. So yeah, a bit of ramble there, but you know, legislation, technology, those two things catching up to where we’re at.
[00:07:29] Dara: So even though the observed data is becoming less and less, the fact is analytics data, web analytics data, it’s never been entirely accurate anyway. There’s always been reasons why data will be missed. And something else that hasn’t changed and it has always been an issue is even when cookies were more prevalent and less likely to be eradicated, deleted, destroyed. Cross-device, cross-browser, that was always an issue anyway. And some of the modeling techniques are actually improving the accuracy compared to how things were in the past. So it’s like a bit of a blend isn’t it. In some cases is trying to plug a gap that now exists that maybe didn’t previously, but in other cases, it’s advancing the technology. So maybe we’re going to be left somewhere in between the two. And, really this data is better used for trend analysis anyway, and it has never been entirely accurate.
[00:08:28] Dan: Yeah, for sure. I mean, no doubt this has never been completely accurate. You know, we’ve always validated things to even 10% differences compared to a source of truth. And we’re like, yeah, that’s probably about right. And that’s the closest we’re going to get. And then we move on with our lives and we use it exactly as you said Dara, for trends for comparative change. Rather than thinking of it as the total revenue you’ve earned, it’s a change compared to a previous period, in which case that’s the interesting part. But the thing that I don’t know if I necessarily 100% agree with, is the fact that the modeling makes it more accurate. Again, take the cross-device users for example. Yeah cookies have always been an issue in terms of measuring a person right. We can never be able to measure people in something like Google Analytics it’s always been cookies that we relabel as users or visitors, whatever you want to call them. But we’ve got nothing to validate it against. We’re taking a black box algorithm, we’re taking Google Analytics’ word that they’re modeling users and stitching users together and understanding behavior from unconsented users and applying it to the real data, and we have to take their word for it. There’s no way for us to say, oh yeah, that is right, go for it. You know, I, I trust you. I trust this model. It just feels like it’s a decision been made by some. Well, a faceless corporation, they’ve decided to apply this to our data. We assume they have our best interests at heart, and that it’s going to be a hundred percent accurate, but the reality is we don’t know, and we will never know.
[00:09:48] Dara: I’m with you on that, it’s not transparent. The black box modeling doesn’t allow you to dig into and understand how it’s working. I guess, maybe I’m in a more generous mood today, I just think that this is something that has to happen. These gaps exist. Modeling is, well, maybe put it this way, it’s going to be the best that we were going to have. We’ll be left with the best situation available based on the constraints, both technical and in terms of the legislation.
[00:10:20] Dan: That’s the big thing, it’s not our choice, it’s not our decision to make is it? Well, there’s always a decision right, there’s always a choice. And I think something like Google Analytics is quite clear. If you want to use our tool, you sign up to our terms and conditions. Otherwise, there are a hundred other ways to collect data. You don’t have to use Google Analytics. So the deal has been sweetened with this easy to install free product, or free version of a product that anyone can use. That people are familiar with, there’s those of online training and resources for it. But you don’t need to, go back to the log files, set your own analytics tool up sending data directly to a data warehouse somewhere. I mean, all this is completely possible, but people don’t, and that’s the thing is that people are kind of pushed into a corner. Especially companies that don’t have a analytics team resource or data engineers or scientists, you know, they’re kind of put into a corner and then someone’s come in and said, by the way, this is happening. So maybe I’m in the cynical mood right now. And that’s where I’m coming at it from. But, I’m going to happily go along with this and use it and, uh, make the most of it of course. Like I said, I’m the cynic here and I’m coming at this from a, can we really trust it perspective. Even though realistically this will roll out and we’ll not think twice about it going forward.
[00:11:25] Dara: So let’s talk about some of the cases, some of the uses of this modeling. So Google Ads has been modeling data for some time, specifically around conversion modeling where there’s no cookie data available like restrictions around safari or if cookies are deleted, also the cross device conversions as well. And modeling is used with the store visit data within Ads as well. And even GA Universal Analytics has been using some modelled data for a number of years. The user’s metric was changed, I’m not sure when it was, but several years ago where it’s not counted anymore, it’s modeled using Hyper Log plus plus, which I’m not going to explain because I don’t know how, um, but GA4 is going to be introducing even more modeled data, isn’t that right Dan?
[00:12:16] Dan: Yeah, it is. And on top of what it already has, right? So GA4 is already, well, the sales pitch of GA4 is that it’s built on machine learning. And the way that it kind of justified that since day one has been around using predictive audiences and predictive modeling that’s built in. So predictive conversions, predicted churn, using the churn and conversion probabilities in creating custom audiences. Those kinds of things have been there for a little while now. But what they’ve introduced as of the end of July 2021, they introduced conversion modeling into GA4. And it’s very similar to how Google Ads does it. These tools are overlapping all the time, they’re both basically the same team that work on this stuff. But the GA4 conversion modeling, what it does is it identifies viewable users that convert via everything that’s not direct traffic. And then it takes all of your users that converted directly, so no campaign data was available. And then it tries to model the actual campaign that drove that conversion. So, so it’s a bit of an odd one actually, because it’s not creating conversions where there were none. This isn’t a conversion modeling in the sense of modeling out unconsented users or filling in the gaps to fit your backend systems. It’s just about saying, when a user looks like a new user, they come to your website or your app and they purchase or convert in some way. And there’s no marketing data attributed it to that on a last click basis, they try to model, based on viewable data, what that channel was that drove them there. So in a way, trying to do things like cross-device and all that other stuff, but without actually doing fingerprinting. They’re just modeling the viewable onto the non-viewable data. And I just wanted to, I’ve got it open here. I just want to read through some of the use cases or some of the scenarios where this conversion modelling comes in and it kind of covers every base possible. So let’s see what you think here. So examples of conversion modeling in GA4:
Browsers that don’t allow conversions to be measured with third-party cookies, we’ll have conversions modeled based on your website’s traffic.
Browsers that limit the time window for first party cookies will have conversions beyond the window modeled.
Some countries require consent to use cookies for advertising activities. When advertisers use consent mode, conversions are modeled for unconsented users
Apple’s app tracking transparencies, that’s the ATT thing that iOS 14.5 introduced recently, requires developers to obtain permission to use certain information from other apps and websites. Google won’t use the IDFA. So conversions will be modeled in that instance.
When the ad interaction and the conversion happened on different devices, conversions may be modeled.
Conversion modeling covers both click-based events and engage views for YouTube to help with attribution for engaged view conversions, that’s the EVCs.
And lastly, any conversions important to Google Ads from link to Google Analytics 4 properties will include modeling.
And there’s a big yellow tip box at the bottom there, I would say more of a warning. When looking at Google Analytics reports, keep in mind that attributed conversion data for each channel can still be updated for up to seven days after conversions is recorded. What that means, what it’s basically saying is, you can’t use Google Analytics for the last seven days of data because it’s likely to change the attribution. The channels for that conversion is likely to change, which is kind of crazy. We’re basically saying, Google will continue to be modeling your data days after it happened. And thus the kind of approaches that we might be more familiar with with Google Analytics of like going into the office, when there was an office to walk into, check your data for yesterday, that was where data for yesterday, that’s moved on with our lives. Whereas now it’s saying that up to seven days later, that modeling is still going to take effect or still be applied to, to your last seven days of data.
[00:15:48] Dara: Practically, I just found myself thinking, I can imagine it being almost a bigger issue the fact that it’s going to continue to update the data for seven days. If it’s going to be modeled and if it’s gradually moving towards a point where more and more of the data is going to be modeled, I can imagine most people are going to accept that because they’ll just take what’s there and their understanding of it as being the numbers they’re using. It’s almost a bigger issue if those numbers are gonna continue to change. So you go back in seven days later and the numbers of all changed. That practically could be a bigger issue for people than the simple fact it’s based on modelled data.
[00:16:23] Dan: So we’re going to get more of those questions of, oh, my reports don’t match. Those are the things that can be a hundred different reasons right now, and now we’ve got another one to add in there. It feels, it feels weird, right? It feels weird to say in Google Analytics, you can’t report on your data for yesterday. It just feels crazy that we’re saying that the data isn’t accurate to a point where on Monday morning, you can’t run last week’s data ready for your sort of stand up in the morning or whatever you do within your organization. It just feels a bit, I dunno what I’m trying to say actually, it just feels a bit odd. It feels different. And I don’t know if different is good. And again, it’s one of those things I think we’re just going to accept and move on. But I don’t know if I, I like it right now. You know, again, I, will have to accept it, but I don’t know if I like it.
[00:17:08] Dara: It’s going to cause some headaches for sure. it was always the case in Ads though isn’t it, that the data could continue to update because it was attributed back to the click. So if you looked at conversions for last week today versus looking at the same week tomorrow, the numbers could change. So it’s not like this is a completely new concept, but it is new within Google Analytics.
[00:17:31] Dan: Yeah, it’s new within the Google Analytics sphere. The attribution modeling methodology might be the same now, both maybe synchronizing to data-driven attribution or whatever. But you’re absolutely right that Google Ads has a interaction time attribution approach. Whereas Google Analytics had a conversion time attribution approach. So the date the conversion happened stayed on the day the conversion happened, even if you reattribute to different campaigns. Whereas in Google Ads, it attributes it back to the date of the interaction, so the click if it was a search ad for example. Which is better for doing any kind of marketing ROI or cost per acquisition, anything like that, because if you have your one pound of spend 10 weeks ago and your conversion today, your ROI is going to be all over the place. It’s really hard to do, so I completely get why it exists. But Google Analytics has always been traditionally conversion date. Google Ads has been interaction date. There’s still gonna be that difference, but the concept is the same. In Google Ads you kind of half expect data to always be in flux and changing. In Google Analytics we don’t expect that, and now we have to start expecting that.
[00:18:32] Dara: There’s, this might be slightly oversimplified, but there’s two types of modeling we’re talking about here isn’t there. You mentioned about in GA4, where it was always the propensity modeling and the churn is kind of predictive. Whereas these changes that they’re introducing are more about plugging the gaps of what’s already happened.
[00:18:52] Dan: Yeah, absolutely. Those two types of modeling is really interesting because when they’re laid on top of each other, it adds almost like an exponential fuzziness to the underlying data. So you take data that is partial and then Google models the difference. So all of a sudden you are collecting, let’s say 60% of the traffic data on your website, Google goes in and it models the difference, or at least what it expects the difference to be. It then does the conversion modeling, where it tries to reattribute the channel for the conversion. And then it’s applying a machine learning, well they’re all machine learning models, but it’s applying another machine learning model to do data-driven attribution across that. So when you get to the reporting, the other side of it, and you say, what is my revenue per channel or revenue per ad, all of that compounds. So where do you sit when you pump out a report and you put a pie chart of revenue by marketing channel at the end of it. At what point do we say I have to just accept that that is the best we’re going to get a move on. Maybe it’s, sorry, I’m being slightly negative with all this. I, you know, I think this is amazing work of machine learning models and the use of Google to a confidence they must have in this to roll it out to almost absolutely everything within their Google Marketing Platform in Google Ads and Analytics and whatever. But I think, where I’m at with this, we knew that the data that we are outputting out the other end, we could validate that. We could go in and we could validate the accuracy of that data to some extent to a five, 10% difference for example. Whereas now we look at that data and there could be underneath all that a flaw in our tracking technology, there might be a bug in our GTM implementation and we just wouldn’t know. There’s going to be no indicators to say this isn’t accurate, we’re just going to keep running with things. And I think that’s where, maybe for the old school analytics people like us, I think that’s the biggest fear I have is, well how are we going to know if things are right or wrong. But maybe again, maybe that doesn’t matter.
[00:20:47] Dara: That’s what I’m wondering, and we can still, maybe I’m misunderstanding this, but we’ll still be able to validate total conversions.
[00:20:55] Dan: But what if we can’t? What if we’re only tracking 60% consented data and that is the data that’s exported to BigQuery. And then the machine learning models get applied on top within the walled garden of Google. They’re not going to do that in BigQuery, by the way, they’re never going to give us access to that. So we’ll have 60% of our data export it to, or tracked, consented, full data we’re used to seeing in BigQuery. But then the difference is modeled and then we’ve got data-driven attribution, all the other things that are applied on top, before we see a report through the UI or the API. So that’s where I don’t get, how do we validate that? How do we know that there’s not an issue with the tracking rather than, for example, consent? Hard right. I don’t know, this is, this is my conundrum. This is why we’re chatting about it now really is just to kind of see what we think.
[00:21:37] Dara: Yeah, it is hard to know and I know it keeps saying the same thing, but we don’t have a choice. So this is the direction it’s moving in. And maybe you’re right, maybe we won’t be able to validate it and maybe we won’t know. I suppose the bigger problem is not knowing how far off it is if we don’t know how wrong it is, or if we don’t know that’s the problem with not having consent, isn’t it. We don’t know how many people are not consenting. We don’t know how many people were missing. And it’s one thing with conversions, it’s a bigger thing with traffic and page views because we simply won’t know who’s not getting tracked
[00:22:10] Dan: And this is actually not a unique case to Google Analytics. This is something that we might be more familiar, me and you at least Dara with the Google Analytics side, but there’s the whole concept of GAFA right, the GAFA. So this is the Google, Amazon, Facebook and Apple ecosystems. So these four big players, they have their own walled gardens. Everything existing within that is kind of within their terms and conditions. They can share data within that, but we can’t as a representative of an organization or as an individual, we can’t access that data. So we’ve always had this right. We’ve always had this, if we take the Google Analytics side, we’ve always had something called Google Signals, well now called Google Signals. But this is the demographics and interest data that Google Analytics has always had. Age, gender, interest reports like the in-market segments, all those things are available to use in Google Analytics. It’s not everybody. So it’s only where Google can join those dots and that information has been provided. And those kinds of things are not exported to BigQuery using Google Analytics 360. So when we get into GA4 is no different, right? We’ve got Google Signals enabled, we can get that same data into the data set. We’re not going to get that to BigQuery. But now imagine that times ten right. So not only are we going to get age, gender, interest reporting, but we’re going to get conversion and behavioral data like sessions and user data modeled on top of that. So now what’s happening is at least the way I think about it is that divergence between the data available in the UI and the data will have access to in BigQuery. The data we get in BigQuery is the consented data that we can collect based on cookies, user ID, whatever we can get that’s completely legit. But the data in the UI is going to take that and then apply this walled garden modeling. So if you’re on the Google world, it’s going to take the Google Signals, it’s going to take the behavior modeling, it’s going to take the conversion modeling, it’s going to do whatever it needs to do and make that better. Or at least from the outside perspective better. But we’re not gonna have access to that, or at least not directly. but the same with Amazon, Facebook and Apple, they do the same. There’s walled garden states within these four companies and loads of other companies too, but these are the big players. Our first party data is always going to be a subset of this, and that’s the data that we need to be looking at. That’s the data we can do our own attribution modeling on. Everything else is at the mercy of those walled gardens. Wherever happens in the walled garden, stays in the walled garden. I think that’s the key thing.
[00:24:26] Dara: This isn’t a new problem and this is, think you mentioned earlier, this is a case of the technology in a way catching up, and the increased need. And this is let’s be honest as well, this is a good thing. The increase in legislation around user privacy is in principle a good thing. And these problems aren’t going to go away. And the use of modeling is gonna only become more and more prevalent, both in Google Analytics and any other marketing analytics and ad technology. So we are stuck with it, we have to adapt and maybe we just need to accept that we’re going to have less and less visibility. We’re going to move away from counting things and have to start trusting what we’re told. Right Dan, when you’re not reading up on privacy legislation and GDPR and cookie-less tracking what else have you been doing lately?
[00:25:22] Dan: Well, there’s always time to catch up on my legislation reading at night, but when I’m not reading up on that, I think that the thing I’ve been up to most recently has to be a new TV show on Netflix I’ve been watching. I think everyone else in the world has been watching, it’s called Squid Game. And apparently it’s been going pretty well for Netflix. It’s on track to be the most watched thing ever on Netflix, which is crazy. It’s a South Korean TV show that’s kind of like Battle Royale meets Hunger Games, where a bunch of contestants go in to a fight to the death to win a bunch of money. And it’s awesome. The aesthetics, the music is awesome. The stories wicked as well. I highly recommend anyone that’s maybe umming and ahing whether they want to watch it. I reckon you should just do it.
[00:26:05] Dara: Well I can include myself in that category, I have not seen it. I’ve heard about it, but I’m always out of touch. So yeah, I’ll be, I’ll be late to the party.
[00:26:14] Dan: So Dara, tell me, is the cabin finished?
[00:26:18] Dara: No, but I have an excuse. I was in Ireland for the first time in almost two years. So I was seeing my family, which was great. I had a nice time, it was a short trip, but I got to catch up with family and, managed to get out for a few pints of Guinness as well. So had I had a nice time. That’s us for this week. As always, you can find out more about us over at measurelab.co.uk. You can get in touch with us by email, at hello@measurelab.co.uk, or you can find us on LinkedIn. If you have any questions, or even if you have an opinion about something in the analytics industry then why not get in touch and join us as a guest on The Measure Pod. Otherwise, that’s it for this time around, join us next time for more analytics chit-chat. I’ve been Dara joined by Dan. So it’s bye from me.
[00:27:08] Dan: And bye for me.
[00:27:09] Dara: See you next time
Daniel Perry-Reed
Subscribe to our newsletter:
Further reading
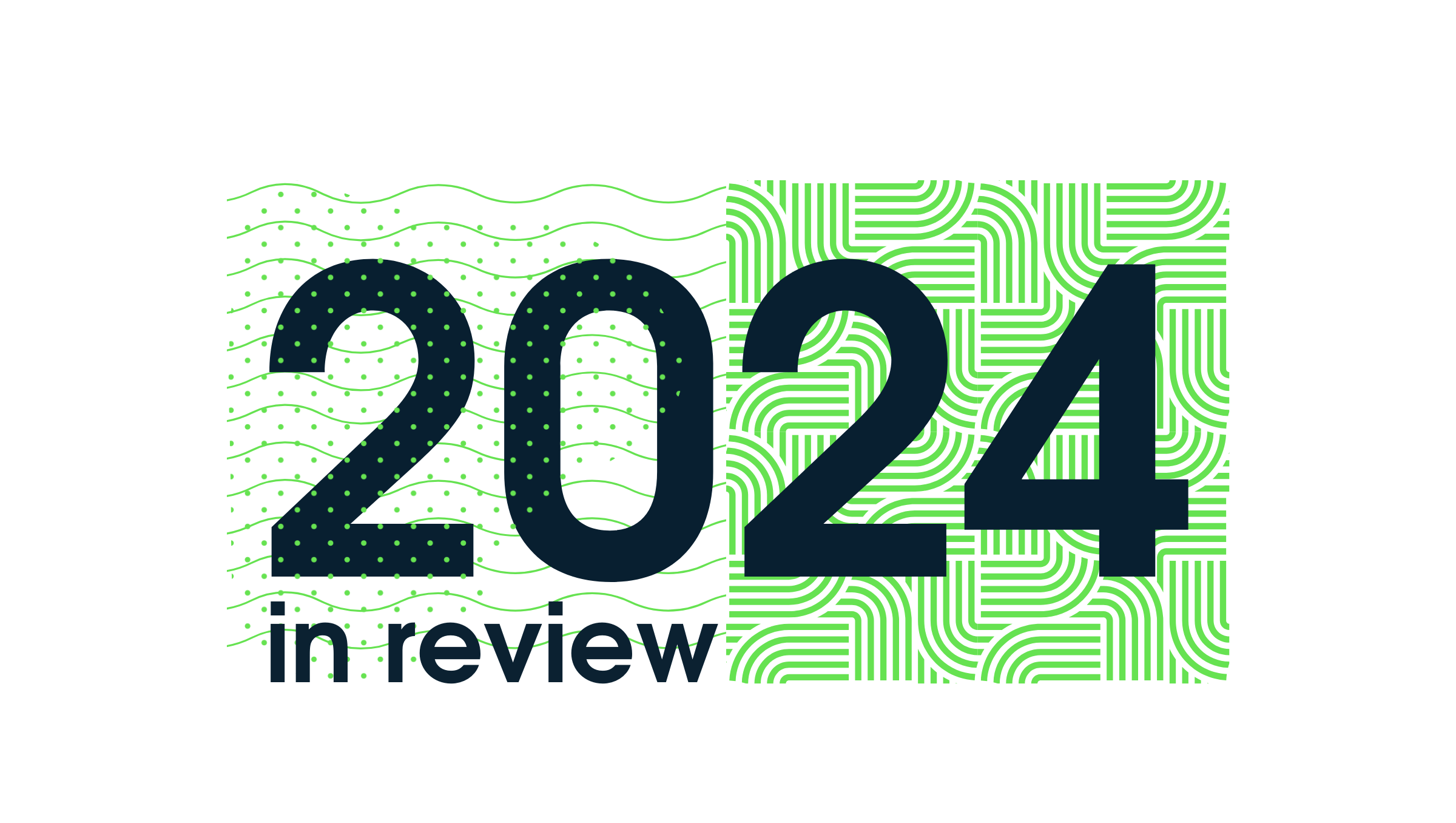
2024: Measurelab’s year in review
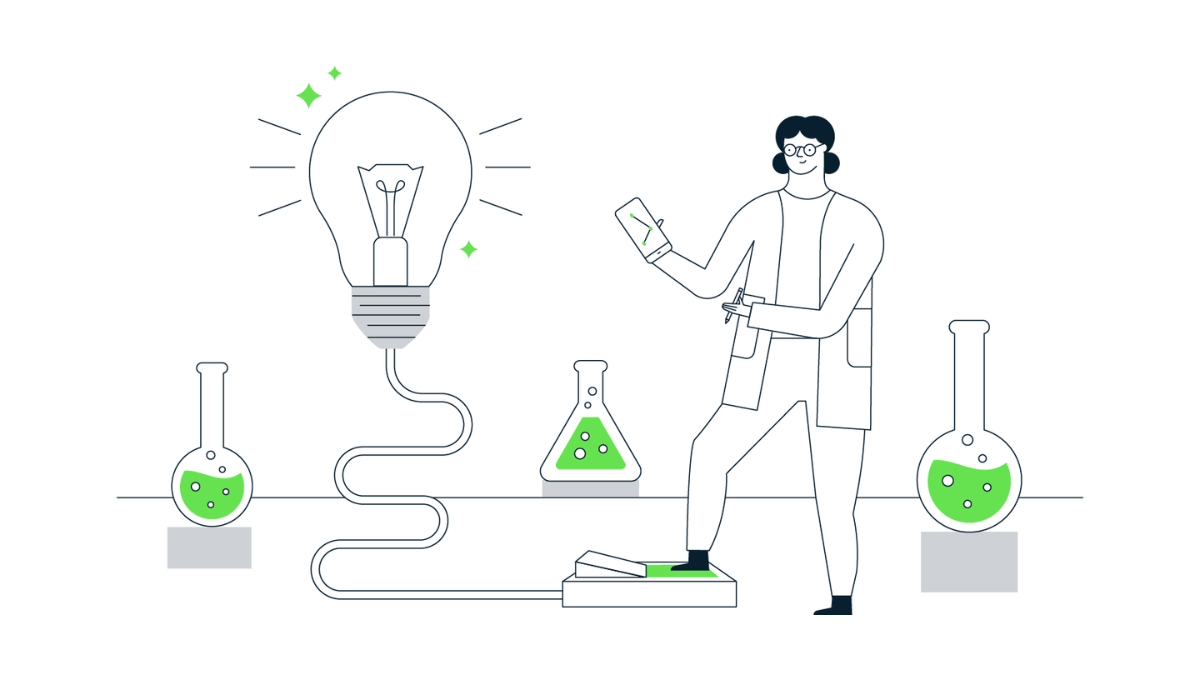
A step-by-step guide to migrating scheduled queries to Dataform
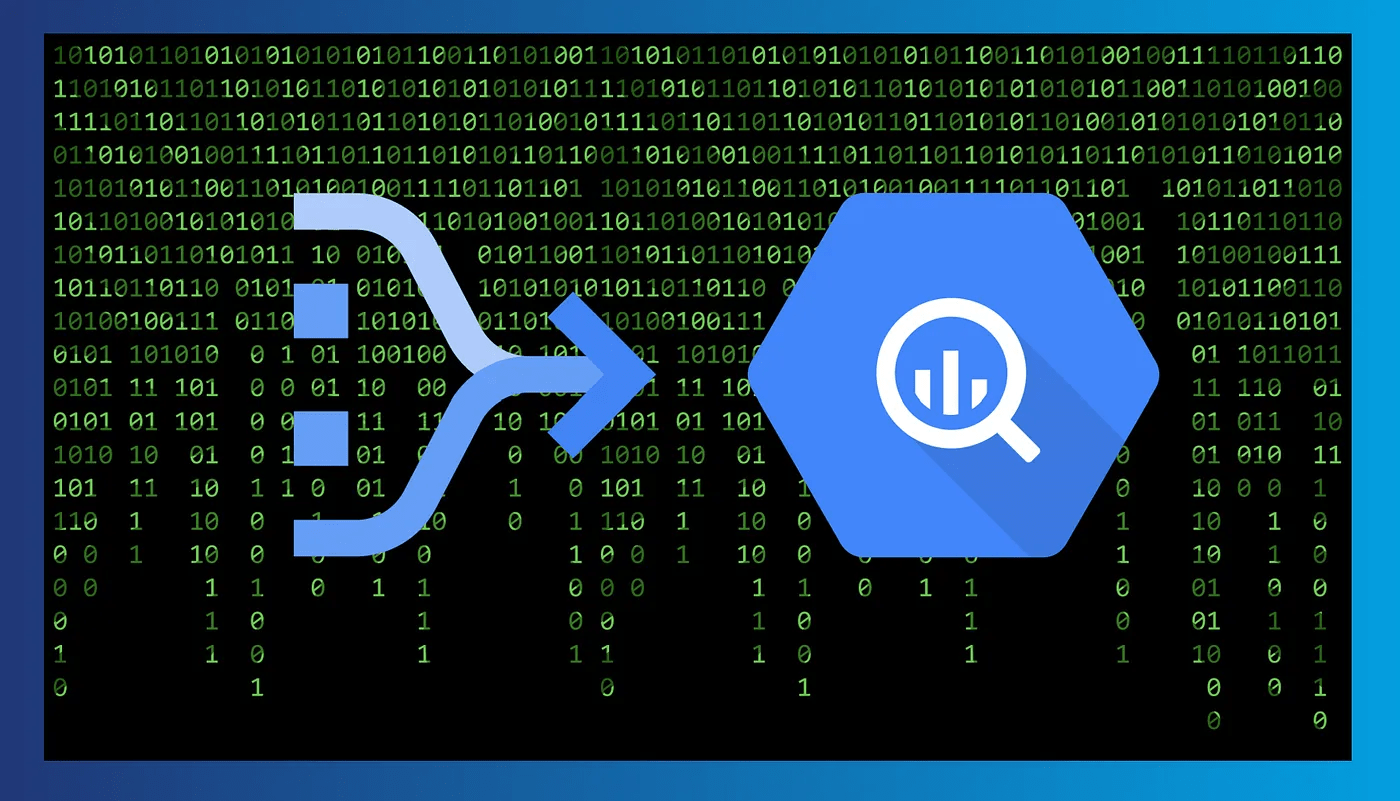