#113 MMM, MTA and the future of marketing measurement (with János Moldvay @ Funnel)
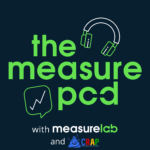
In this week’s episode of The Measure Pod, Dan and Bhav are joined by János Moldvay from Funnel to dive into the intricacies of Marketing Mix Modeling (MMM), Multi-Touch Attribution (MTA), and the future of marketing measurement. János shares his expertise on analytical methodologies and highlights the critical role of measurement in shaping effective marketing strategies.
Show notes
- János on LinkedIn
- Funnel Measurement
- Avinash’s article (Bhav’s reference)
- Google’s “The modern measurement” playbook
- Ken Williams episode on modern marketing
- Marketing Measurement Matters podcast
- MMM Hub (podcast and Slack)
Share your thoughts and ideas on our Feedback Form.
Follow Measurelab on LinkedIn and LeanConvert on LinkedIn.
Join the CRAP Talks Slack community and follow them on LinkedIn.
Music composed by Confidential – check out their lo-fi beats on Spotify.
Transcript
Last click is like McDonald’s. You know it’s crap. You know you shouldn’t go there, but you know it’s there.
János
I always consider these different measurement techniques as like lenses you apply on the data, right? They just tell you different stories and collectively you can make up the narrative and join the dots between them.
Dan
[00:00:00] Dan: Welcome back to The Measure Pod. This is episode 113, and we have a special guest today. Janos, who is from funnel. That’s going to be talking to us all about MMM MTAs and measurement or marketing measurement as a whole. I’m Daniel Perry-Reed. I’m a principal analytics consultant at Measurelab.
[00:00:31] Dan: And as of course, always, I’m joined by Bhav Patel, who’s director of analytics and experimentation at lean convert. Well, that’s over with, Bhav. We’ve just come off this and we both lost complete track of time and I think we ran pretty long on this one, so apologies. Let’s see what the actual final time is when we edit it down. what’s your thoughts?
[00:00:50] Bhav: I’ll try and keep this brief just because it was a slightly longer episode and for, you know, for the first time in a long time I actually lost, lost track of time. I think it was a, it was a really interesting episode because We don’t typically go into the amount of depth we go in, we went into in this episode with, with many of our talks that we’ve had.
[00:01:08] Bhav: So it was actually kind of refreshing to be really going in depth. And then if anyone’s listening, who doesn’t maybe come with the same sort of like background in, in MMMs and MTAs and all these types of things, I would say just bear with it. We do try our best. to explain certain concepts, in a way that hopefully is not patronizing, but allows you to follow the conversation from start to finish, with some really good, I think Janos provided some really good insights and takeaways that you could apply or at least bring to the table to, in your own organization.
[00:01:36] Bhav: So I think it was a very practical episode, which I know, we don’t always do the most practical ones. Sometimes it’s just us being contentious.
[00:01:45] Dan: Yeah. Or, or, or me trying to find something out for my own personal game. So there’s that. Okay, perfect. Let’s, let’s jump in. Is there anything you want to plug or mention that before we jump in?
[00:01:55] Bhav: No, it’s Christmas, or coming up to Christmas. I’m not sure when we’re gonna release this episode, but I hope everyone has a nice break. Take some time off, do some reading, spend time with your families, and just, you know, just, just be present. I guess that’s, that’s, that’s, that’s my Christmas message.
[00:02:11] Dan: Oh, that’s beautiful. Well, that’s the completely, makes my plug look really rubbish. but I was going to plug another podcast and another resource that’s all about work and analytics. So, of course I, I echo the sentiment. I think everyone have a great, holiday, festive period, X mas, whatever you celebrate. but I do want to plug something and of course links will be in the show notes.
[00:02:31] Dan: but, if anyone is interested in MMM and wants to go deeper and enjoys podcasts, as you’re here listening to us now, there’s a great podcast called the measure up podcast, by Jim and co, and they have guests on talking about MMM in more detail. And there’s also a Slack community called MMM hub, which they also run.
[00:02:46] Dan: So I’ve got links in the show notes. check them out. They’re good episodes. I love listening to them talk about this kind of stuff. All right, enough of us yabbering on, on with the show. Enjoy your holiday period.
[00:03:01] Dan: Today, we have Janos joining us from Funnel. And, I, I’ve actually been looking forward to this episode for a good couple of weeks now. So Janos, we had the opportunity to meet up a couple of weeks ago, an event that you held in London. But before I start bombarding you with questions about media mix modeling, attribution, and everything else in between, why don’t we do a quick little origin story?
[00:03:22] Dan: Why don’t you introduce yourself? Tell us how you. came to be here, how you got into this industry and what it is you do now?
[00:03:28] János: Yes, i’d love to and thanks so much for the invitation really looking forward to the session. So, yeah, my name is Janus moldwey. I Was previously the ceo and co-founder of atriba a measurement company that we built for the last 10 years.
[00:03:43] János: So we founded it in 2015 and that eventually got merged with funnel and 2000, well, this year, basically in June, it seems like, almost a more than a year ago or more than half a year ago. And so my background story is I studied computer science, was always really interested in analytics and numbers, decided not to go into typical software development and software engineering was more interested into using business analytics, PI and data science.
[00:04:07] János: back then it was more called data mining, to really sort of create valuable nuggets or insights, that really can drive business value. So for example, to figure out which customer segments one should target, things like that. So I started my first job at a, rather large e commerce group here in Germany, the auto group, where we basically used machine learning models.
[00:04:31] János: to predict which client would buy if we sell them a catalog. That was, that was it. But, that was in, when was that? 20, 2005. So we use machine learning AI, so to say already back then. And, yeah, from, I found that area really interesting. we used, also, the, the, the recommendation, algorithms of Amazon, to kind of, you know, predict which clients we should, show which products.
[00:04:57] János: And then essentially I went to, Social business, social network called Xing, which was the biggest competitor to LinkedIn in Germany. And, that we had a lot of really interesting data, as you can imagine, about all the, members of that network. We did a lot of interesting analytics. So for example, running Google’s page rank algorithm on the network to figure out which, is the most influential member, of that particular business network.
[00:05:25] János: I did an MBA in 2010 in Cape town, South Africa, a lot of kiting, just by accident was also the football world cup. And I remember, Germany beating Argentina four zero. So that was quite nice to watch the life. But, in essence, at some stage I had to get back into real life, so to say, and I went back to Germany, started at an agency and that’s in 2011, where I got in touch with the topic of attribution modeling, customer journey analytics.
[00:05:51] János: And it really, I found it really fascinating, how in the online marketing world, how much data was available. So all the ad server lock files and, you know, GPR and, cookie tracking restrictions when the thing back then. So we had a lot of amazing, valuable data and a lot of questions that needed to be solved and answered, for example, how much value, which campaign, which, marketing touchpoint generated.
[00:06:14] János: And, yeah, that’s basically where I got in touch with the topic of attribution modeling and, the, the. so typically like an agency back then, at least at that particular agency, we already sold the attribution modeling customer journey, the next project before I even started at that company. And so we had to figure out a way on how to actually deliver on that.
[00:06:35] János: So what we did is, We thought through different ways and I remember exactly, laying awake at night and thinking, how can we do soft for this attribution? Ah, we could actually maybe use the coefficients or something like that from a regression analysis to figure out, you know, if we had the converting and non converting journey.
[00:06:53] János: So then really that’s kind of, this idea, started off. And I mean, obviously there were a lot of other, Vendors and companies at that same time that, already had, you know, solutions for that, or we’re thinking about the same things. Yeah. And then, long story short in 2015, I decided with my co founder to, build a company in that space.
[00:07:13] János: we were very much focusing on multi touch attribution. MMM back then was still a thing that nobody really took too serious. It was. The thing that the big FNCG did in the 1980s, but that always lacked the, you know, rich, data foundation that MTA had, right? Because we have to keep in mind multi touch attribution and tracking, consists of millions of data points.
[00:07:33] János: Whereas MMM is, oftentimes very much reduced to a very small data set. Yeah. That that’s basically the story, the origin story. And then there was, as you pointed out. Maybe can imagine quite a few interesting years with GDPR coming out, coming up in 2018 and all the tracking restrictions. I remember Google, we had quite good contact with Google sitting in our office and telling us, guys, actually we launching a, a free for all tool that’s basically doing what you guys are doing.
[00:08:01] János: So we thought numerous times that it’s a game over for us, but, yeah, we, we survived and fought our way through and. essentially at some stage ended up at a, at a stage where we think we, we have a good, good answer for the, for the, questions that marketing managers nowadays have.
[00:08:18] Dan: Wow. I like that. I like how the last 10 years were completely sped through in the whole kind of MMM, process. And then the acquisitions of funnel and stuff. We’ll, we’ll dig into that. I’m sure later on, but I just want to kind of say that I completely, understand. I, I, I started my, analytics journey working for a dedicated attribution platform with our own tag manager software.
[00:08:38] Dan: And, Google started kind of giving out their versions for free and completely destroying our business. We had, and then GDPR was the final nail in the coffin. So, it’s a very similar kind of journey, like thinking about that kind of process from data, from attribution, modeling, when you had everything and then move into, we, we still called it econometric modeling back then, but the rebrands to MMM, I suppose that was something, you That that is coming back, but, but yeah, and also Google releasing kind of like basic attribution modeling and stuff like that for free, kind of like underpinned a lot of the, the move and the shift around.
[00:09:08] Dan: So, I just wanted to say, yeah, I get it. It’s an interesting world and it’s always changing, right. whether the old things come back into favor or whether things like GDPR kind of like turn upside down the things that we’re able to do.
[00:09:19] János: And maybe, maybe to add to that, because back then when GDPR came up and we kind You know, starting to understand the implications of that is we really thought this is a huge, huge disadvantage for European tech businesses.
[00:09:32] János: And, we, in, in essence, and, you know, I think it was actually quite, if you look at it from a, you know, what kind of, perspective this brought in, it helped a lot of marketing managers realize maybe they shouldn’t just only look at clicks and trackable marketing interactions as a way to understand how marketing effectiveness, you know, evolves, but rather understand that there are.
[00:09:53] János: other marketing activities, and, you know, prospecting branding that cannot be sensibly evaluated and tracked through tracking systems. so to say, so in, in hindsight, I think it was, good for us because now we also realize when we look at us based competitors that they might’ve started thinking about this a bit later, maybe that’s also an advantage to some degree, but because they have the green field, but we, we still, you know, when we looked into that and around 2018, and then decided to also, look into econometric modeling.
[00:10:23] János: And time series analysis, our machine learning engineers where they were frustrated. They were like, this is nonsense. Like looking at, you know, only this few data points you can make out of it. Whatever you want to, if you have a CMO telling you, you need to build an MMM that tells you that TV needs to look great, no problem.
[00:10:40] János: Every, every one of us would have been capable of doing so. So that’s kind of the issue that, you know, that we saw back then.
[00:10:48] Bhav: Yeah. I have to ask like What are the other factors that people should be considering in that case? Cause you mentioned obviously clicks is just one of them. I think for most people working in the marketing space, they probably don’t know like what other signals they could be looking at.
[00:11:02] Bhav: And I think this is, as much an education piece as it is, you know, like moving the industry forward. I think first people need to have a baseline understanding of what their options are before they start to think about what they do with them. So I’d love to hear like, and I’m sure our listeners would love to hear like, what other things they.
[00:11:21] Bhav: the average Joe marketing person could be using to help them understand.
[00:11:26] János: Yeah, great, great question. because I think that’s also one of the challenges that we see when we talk to brands is that they are so used to this, let’s, let’s call it the Google analytics world of, you know, marketing performance evaluation that they don’t even kind of see that there might be other ways and, you know, opportunities to look into other marketing activities.
[00:11:46] János: And I mean, through, through this, methodology that we just, you know, kind of started describing econometric modeling or MMM. What you are able to is basically include every sort of potential effect. And I’m not just talking about marketing activities, but also other sort of, you know, factors that increase your bottom or top line as a business, such as for example, Weather or, you know, macroeconomic situation, specific events happening in the world.
[00:12:15] János: You know, the changes in Syria or, you know, wars around the world happening. So these can all be to some degree integrated into such a modeling approach, because what you essentially only need is. Some sort of indication on which day to what degree this, you know, this event happened or, to, to make it a slightly more, practical example for, for example, for TV or offline marketing channels, billboard advertising, what you essentially just need is the daily spend or the weekly spend on the particular activity, to understand whether, you know, to start analyzing if that somehow correlates to your, to your sales or to your you know conversion event of interest if that makes sense
[00:13:02] Bhav: I think for things like that. You’re absolutely right. Like it is, it’s fairly easy to Implement a quantifiable metric into some type of model. You know, use the example, whether, whether it’s something that we can track on a daily basis, spend on offline channels, again, something we can track and contribute. If you’re starting to think about sort of like more world events, how do you build your model?
[00:13:25] Bhav: The that into a model, because it’s not a, it’s not a nice, easy, like number that you can just put in that goes into a regression model that you can then use to calculate this. So, yeah, I mean, you don’t have to give away the traits of this, like the secrets of like, I’m sure people love to hear it.
[00:13:42] János: I think that very much depends on, on the particular use cases. But, let’s just assume we work with a shipping company and we would want to do, MMM for them. so they might be impacted by the war in the, you know, in the, in the sort of near east, region and the, and the difficulties that they cannot potentially ship their, containers to the zoo is kind of channel, adding to that the pirates, you know, pirates activity and all the difficulties that come through that.
[00:14:14] János: So they need to ship it around the, Horn of Africa. So Cape town basically, and that comes with, so, and the way they are able to. quantify that is that the, just the cost, are getting much higher and that’s not due to, you know, some supply. Well, you could see it as a supply chain issue, but, that’s something where can they can give us the sort of price index, which is of course caused by, by the wall happening there.
[00:14:40] János: but it’s, it’s, it’s, it’s not, something that, you know, it’s just a zero or one in the field called, you know, is there a wall in Gaza or, you know, Ukraine. I mean, you could even do with potential, you know, some events, you could go about it like that. For example, if you, want to have a thing such as the sport event, Olympic games in the summer included, how are you going to quantify that?
[00:15:04] János: So you have to think about that. and that you could be in just in a way that you use, you know, some sort of binary indicator before you have nothing in there. but it’s always better to, you know, find for something that’s more closely related, such as a proxy. That’s more closely related to the actual business case.
[00:15:21] János: If that makes sense.
[00:15:22] Bhav: Yeah, no, that’s a great answer. I think that makes a lot of sense. Thank you.
[00:15:25] Dan: I’m, I’m, I’m keen to not have to do lots of definitions in the show notes for this episode. So how about we just pull back very quickly and talk about like, what is when we talk about attribution modeling and when we talk about MMM, what are those things and why.
[00:15:38] Dan: Why as marketers or marketing analysts or general data people, should we care about these things and what is the differences?
[00:15:46] Bhav: That is a great question, Dan. I think that’s probably one of my favorite questions you’ve ever asked. Why should we care about this?
[00:15:52] János: Why should we even care? Like I had a, I don’t know if you know him, Ben Dutta from Power Analytics.
[00:15:57] János: I think he’s pretty awesome. we had him on our podcast once and he said, actually, I don’t give a F about attribution. I think it’s just a tool that gets, needs to get the job done. And that’s how we probably should think about it much more. instead of, you know, Seeing it as a, means in itself, so to say, rather understand that it needs to fulfill, you know, business value and the way it can do it.
[00:16:21] János: It’s, you know, very plainly and simply speaking, it can tell you which channels you should not invest in because you’re losing money on them, or they just not performing to, you know, whatever Ross or CPA levels you, set. And which other channels you should maybe invest more money into to grow your business.
[00:16:41] János: So that’s essentially what like it’s, it’s a fairly simple, you can think of it as an, you know, the marketing manager with the budget, being an investment manager and thinking of, you know, having to manage a portfolio of potential stocks, the stocks being the channels that he can invest in and then having to, and that’s a very simplistic.
[00:17:00] János: You know, sort of translation into this world, but then having to decide which stocks to buy or which channels to invest in, in which channels to DMS from. That’s essentially what, you know, measurement should solve for. And then like going into the details, what’s the difference between attribution, modeling, attribution, marketing, measurement, marketing, mixed modeling.
[00:17:21] János: And, maybe even things such as incrementality testing. So the attribution modeling came up through this, you know, Google analytics where we had, where we had last click, but suddenly we also had something such as first click. And for those of you who remember, there was also in Google analytics, linear attribution, time decay, like all these other static attribution models that you could set, I think that’s where the term attribution model came from.
[00:17:47] János: So basically saying, okay, if I have a conversion, how do I attribute this conversion or this revenue to the different marketing touchpoints that I could track in that journey? so that’s, I, I, I, I wasn’t part when, you know, that term was defined. I just used it then, when, when I learned about it or from when I learned about it, so, and, and you could argue that marketing mixed modeling is also solving for the attribution problem, right?
[00:18:12] János: Because we are not, distributing one particular conversion of revenue, that has happened to the participating marketing touchpoints, but we attributing overall for one month, one day. We attributing the sales, the conversions happening to the different, marketing channels that are, that, that a marketing manager drives.
[00:18:32] János: And the big difference between attribution modeling and multi touch attribution, which is, more or less the same, but as the term says, if you think of attribution modeling, last click can be an attribute or is an attribution model as well, similar to first click. So it’s just, you know, based on one touch point, either the first or last.
[00:18:52] János: So multi touch attribution. Means that you’re looking at all the touch points that have happened, the trackable touch points, so mostly clicks. so that’s multi touch attribution and then data driven multi touch attribution to make things even more complicated is when you try to attribute the value based on data driven algorithms or data driven, in this case, machine learning algorithms, so that you, for example, in our case, we have converting.
[00:19:19] János: user journeys and non converting journeys and then we try to learn with the help of machine learning algorithms which marketing touchpoint delivered how much value. so you can think of it as understanding the conversion rate of marketing interactions or marketing touchpoints and for that for calculating conversion rates you obviously don’t only need the Converting journeys, but you also need the non converting touchpoints.
[00:19:43] János: so similar, if you want to evaluate, and I’ve talked about this example, I think literally a thousand times past couple, on the last decade, so to say, I mean, if you think of it as a football player, European football. The real football.
[00:19:57] Bhav: Thank you for clarifying . Sorry for being that, just in case any of our American friends are listening.
[00:20:03] János: Yeah of course we can also talk about quarter quarterbacks, but I mean, of course quarterbacks, like, if they have missed pass, you wouldn’t evaluate a quarterback solely on the successful passes that he played, but also, accounting for all the missed passes that he played. So this ratio, this conversion rate is important.
[00:20:18] János: Right? And same for a midfield player in real football. you know, you wouldn’t only look at them, from, you know. The perspective of how many successful awesome passes he played, but maybe also how many mispasses that might’ve led to a counter strike, for example, and a goal, received, so to say.
[00:20:35] János: So that’s the idea between, behind data driven multicharge attribution. And there are many different algorithms that can be used, Markov chain, Monte Carlo, Markov chains, and analysis shapely are some of the more common ones. you can apply logistic regression. what we do is we use a particular type of deep learning algorithm called long short term memory network.
[00:20:55] János: which automatically accounts for the, for the sort of sequence information. so these long term short term memory networks come from Google, Google has used them, for language, translation at some point, because language is also just a sequence of words and then hence also letters. So, you want to understand the sequence because it made, it makes a difference if you have your Google touchpoint first or your Facebook touchpoint first in a journey where you have both touchpoints included.
[00:21:22] János: So that, that’s, that’s the explanation about, attribution modeling for detached attribution. And then in essence, the big difference or the, the big challenge, so to say with that is that it’s based on. Tracking data. And as we’ve just discussed GDPR, and then all the tracking restrictions that came along, primarily driven, by Apple, through, you know, ITP and the, iOS updates that they have driven is that, yeah, these, these things were heavily impacted.
[00:21:51] János: And then, also, you know, some businesses, even decided to Completely not to multi touch distribution anymore and go all in on marketing mixed modeling. And the big advantage of marketing mixed modeling is that you don’t need user level data. you can do marketing mixed modeling, marketing evaluation, with, you know, fully GDPR compliant without having to use any PII.
[00:22:13] János: or, or, you know, GDPR, relevant data.
[00:22:17] Dan: So, I mean, I suppose that brings up the obvious next question is why do people still use multi touch attribution? And why is not everyone just doing MMM? And have we not moved on with the technology? If that has flaws and this kind of addresses those, like what there must be like the other side of the coin.
[00:22:32] Dan: What’s the cons with the MMM that multi touch attribution is essentially solving for, for those.
[00:22:39] János: Yeah, yeah, exactly. Great question, because that’s, you know, what everybody thinks once we, tell the story on how MMM is also all these privacy issues and additionally, huge advantage, you can also include offline marketing activities, things that you cannot, you know, track through tracking system, and you can include non marketing effects for really understanding commutality, because that’s what we in the game for, right, we want to drive incremental impact and not just, you or, or retargeting or discount affiliate campaigns that, might not drive actual incremental impact.
[00:23:14] János: So why wouldn’t we go all, and literally we as the, as the achiever, company, so that the company that got acquired by funnel, we had. Situation where we thought, okay, should we just pivot into MMM like fully and, which would have made sense, right? Also to our investors, I think they would have completely bought it and could have, I don’t know, probably raised another internal round of financing round.
[00:23:40] János: But we thought, well, we understood a bit about, you know, the, the amount of data that you would have an MMM because we come from a machine learning background. And we’ve like, as I said, our machine learning engineers were not. very positive in terms of the amount of data that we have with MMM. And they were, they were very skeptical if this could even work out.
[00:24:03] János: So our first experiments were rather surprisingly positive so that the models were sort of robust, but the more we kind of dove into MMM and the more we found out about MMM, we also saw, saw. that it can, that, you know, MMMs can fly around all over the place. Meaning, you can have, three very good statistical, or three good MMMs in terms of statistical KPIs.
[00:24:27] János: So great, you know, low, MAPE and high R square or whatever kind of KPIs you’re using on the holdout sample, for example, for, with regards to MAPE, but essentially giving you. different and contradicting, recommendations in terms of, you know, Oh, this model tells me to invest more into TikTok and this other model tells me to invest less into TikTok.
[00:24:50] János: And that’s, if you, if you talk to other MMM practitioners, they might not tell you this on record, so to say, but that’s something that a lot of MMM practitioners will, will also tell you. And, And that, that, that’s why, you know, again, short, summary here, that’s why we need other ways to sort of, make sure that MMMs are being calibrated, validate, however you want to call it, but in a sense, make, make more robust.
[00:25:18] János: You shouldn’t only count on MMM, at least not if you also want it as we as a software as a service business, do it in some sort of, you know, self service capacity.
[00:25:28] Bhav: I mean, it still kind of ties back to what we were talking about. So not, not so much, why should we care, but now kind of like, we’ve got all of these models to choose from all these different platforms that do different things.
[00:25:39] Bhav: And if you’re in the marketing team, which model do you listen to? Which model do you use? Because. You have your last click, which everyone is comfortable with. They understand how it works. And it says that for this channel, this is the CPA, or this is the RO or something like that. Then you get a little bit quote unquote sophisticated and you start doing first click or some type of decay model, or even, you know, some evenly distributed version of your attribution model, and that tells you something else.
[00:26:09] Bhav: And. Then you kind of introduce these more advanced methods where you’re looking at the MAPE or the R squared value, and it’s moving away from session or click based model to a more holistic model. And that tells you something maybe slightly different to what your last, because Most people will still be able to do some type of like comparison.
[00:26:30] Bhav: What do you, what do you listen to? Like, what are people going to listen to? What’s going to work best? I know you’re probably going to say the one that funnel uses, but let’s say you’re going to be objective in this instance.
[00:26:41] János: No. Yeah. I mean, obviously the one that funnel uses. No, no, no. so I mean, and it’s, yeah, it’s interesting that you mentioned that because we see still.
[00:26:53] János: So when we started 10 years ago, our, like. Our main, how should I put it, like challenge was to convince people not to do last click anymore. So, you know, because we, we try to come up with football analogies all the time, specifically, you know, being a German company. So we started in 2015, 2014, we won the world cup.
[00:27:12] János: So we said, And I don’t know who of you remembers it, but there was one player who scored the final goal against Argentina, Mario Goetze. And we always said, in the pitch, Mario Goetze won the World Cup for Germany, right? He was the only, like, he could have done it alone, right? And then I was like, what the heck are you talking about?
[00:27:30] János: And then we said, look, this is what you’re doing with Last Click. it’s complete rubbish. It’s like, yeah, it’s and, and Tim, our, chief data scientist back then, he’s now with funnel as well. But he always said last, last click is like McDonald’s, you know, it’s crap. You know, you shouldn’t go there, but you know, it’s there like, and everybody goes there now and then.
[00:27:51] János: And so, you know, it’s, it’s somehow a standard that everybody’s kind of grown up with. And the thing is that it’s. It’s still hold some sort of information, right? You kind of know, but is it the only information you should look at when driving your whole marketing, which budget decision making probably not.
[00:28:12] János: Right. And it’s still also just a model on how your marketing, like everything we talk about today, all the different, like MMM testing, whatever, are all models to arrive at some sort of understanding of reality, right? And then the question is, how wrong are these models? Even the most sophisticated MMM with whatever algorithms, Bayesian frequentist combination, I don’t care.
[00:28:35] János: They are all just models. And, and similarly to last click, first click time decay, They might be more useful than not. And, but, but I think what’s important is to understand the limitations and that we, as the sort of marketing community need to talk about these limitations very, very openly and not pretend as if any of these approaches is the holy grail.
[00:28:57] János: And of course, I truly believe that it’s better to. Do MMM and do data driven multi touch attribution and look at things as, you know, statistical KPIs, then solely doing last click. That’s, that’s true. But truth to be told in our funnel and measurement dashboard, we also show a KPI. Called last click just for reference.
[00:29:17] János: So we’re not saying like, we’re not kind of patronizing marketing managers to say you are forbidden to look at last click. It’s, you know, it’s the, you shouldn’t do this. that’s not what we’re doing, of course, but, yeah, we just want to make sure that people understand the limitations of that.
[00:29:33] Bhav: I have to, only because you used the McDonald’s analogy, which I really like, by the way.
[00:29:38] Bhav: and I actually, I really liked the Mario Garza analogy as well, because I think I completely get it. There is a, there’s an analogy that was told, said by Avinash Kaushik, many years ago. you’ll probably be familiar with it. He said, attribution modeling, attribution modeling is a bit like thanking your first girlfriend for your current wife.
[00:29:55] János: Yeah, right. Well, it’s the same.
[00:29:59] Bhav: maybe, you know, you could do so. But yes, but I guess it comes down to and I get what he’s trying to say here, right? It’s I don’t think it’s an absolute, just like the Mario Garza analogy is not an absolute. In some instances, there is a Argument that actually, you know what the sales funnel or the sales process or the sales cycle is so short that you don’t need to look back like, like first click, you know, like offline, like they just, you know, the purchase, the path to purchase is so short in other instances where let’s say you’re buying a car, you need to look at econometric modeling and multi touch attribution and all those type of things where the purchase consideration cycle is significantly higher.
[00:30:37] Bhav: So do you think. That’s a bit of a loaded question. Do you think this is this approach is for everyone?
[00:30:44] Dan: If you set up a Google BigQuery export from Google Analytics 4, and you’re not really sure what to do with it, it’s your first time using the GCP, then give us a shout. We’re here to help. We’re GCP certified, GMP certified, and of course, I can’t stop talking about Google Analytics 4.
[00:30:58] Dan: So we’re here to help you make the most out of that data and show you exactly how powerful it can be. The world of cloud databases in the Google cloud platform can be complex and overwhelming at times, but ultimately it’s there to serve a purpose and to make the most out of the data you have, you can reach out to us by scanning this QR code, or you can click the link in the show notes to find out more, or you can just head over to measurelab.co.uk and find out a bit more of what we’re about.
[00:31:24] János: Good question. I mean, I just have to mention, I think Avinash, and I really admire him. I’ve read a lot of his blog posts, but I think that was when he was still employed by Google, right? So, and you have to think of who, who profited most from last click, back then. So, No, but in all honesty, I think there’s some truth to that.
[00:31:42] János: so of course, like businesses that are just starting up and, you know, maybe below 10 million in, in, revenue, yearly revenue, maybe it’s not the most important thing to look at it, but they still should understand the limitations of that. So, I mean, we’ve grown up with businesses that came from the performance marketing world.
[00:31:59] János: So Flixbus is a really good example of that. I don’t know if you know them, but they own off Greyhound bus in the U S. Like they want to be the worldwide leading coach, bus coaching service, so to say, and they, they started off as being the best performance marketing managers and, and, you know, or best, one of the best performance marketing managed, managers and, and, really excelling and, performance marketing, Google ads, et cetera.
[00:32:24] János: But then at some stage they realized, and I think for that, you could have even argued that last click is not the worst. You know, type of decision making rather look at last click and Google analytics and see also other clicks from other platforms than just looking into Google ads where you wouldn’t even see Facebook clicks, for example.
[00:32:40] János: Right. So, I mean, last click is still better than looking into each app platform in isolation, if you do, for example, elastic analysis and Google analytics, you could argue. So I think. Yeah, for, for that case might make sense. But if you, as soon as you start driving up a funnel type of campaign, and I’m not even talking about, you know, offline, maybe just like meta prospecting campaigns, both, well, first they’re usually not the last click in your journey.
[00:33:06] János: And maybe they are not even a click. So, you know, prospecting, branding campaigns and social media, usually the main effect is not coming through, through clicks and visits, on your website directly from that ad, but through sort of a branding type of, impact. And, and that’s where you need, other type of, approaches.
[00:33:27] János: So I wouldn’t say this is for everyone. And of course we need to look into it business, in a more, differentiated way. and also I wouldn’t say last week is, you know, rubbish for everyone.
[00:33:42] Dan: so the, the, the, the, the, the key question here is going to be, and, and, and actually for those, few people that were at the event the other week, in London that me and Janos were at, I asked you the same question.
[00:33:53] Dan: There is, I suppose like when we talk about, having different methodologies of measuring our marketing, it feels like we’re, we’re solving a problem, one problem at a time, and there’s a different perspective because as you go to Google analytics last click. That’s going to be different to the Google ads last click, which is different to the Tik TOK last click.
[00:34:11] Dan: And then your Facebook and your email. And then you’ve got, what we’re saying here is we’ve got MMM that will solve it slightly differently. Essentially we’re, we’re adding more in without removing stuff. So we’ve got this kind of like debt, this kind of like overhead that we’re kind of adding in, which makes.
[00:34:25] Dan: Deciding what to do if you’re that marketing manager, you know, using your stock analogies of like, where do I move my money to maximize revenue? It makes it even harder because you’re having contradictory opinions and you’re adding more opinions, more chefs into the kitchen, which is my favorite analogy here.
[00:34:38] Dan: So how do you solve for that? Because you could argue that I could find a model that, that, that. That proves my point, right? Let’s say I have a, I’m the paid search manager and I’m like, well, I’m going to pick a model that makes mine look great. And I’m going to tell my CMO that I need more budget because look at my numbers.
[00:34:53] Dan: And then they’re, everyone’s doing the same. So how is that kind of addressed or solved? Or at least from your experience, like what’s the, how do you mitigate everyone just finding the answer they want?
[00:35:03] János: Really great question. I forgot to address this one point that you also mentioned, like what we see a lot of businesses working with MTA, maybe Google analytics.
[00:35:12] János: On the one side, and then even, you know, having some MMM practice either, you know, they’ve Work with Robin, they have, capable data scientists doing that, or they’re working with the, MMM only solution. So that also happens. And then you have contradictory contradicting results all the time. And that’s similar challenge, right?
[00:35:29] János: Because you have two systems telling you different things. Most of the times in these cases, it’s like completely different departments working on different KPIs. Which also is not great, right? Because you know, it’s one might be the branding department, the media department. The other one is the digital performance marketing channel department, so to say.
[00:35:45] János: So how do you, how do you consolidate? And I mean, we have a very specific view on that, but I don’t know if you read this, Google’s playbook on, what’s it called playbook on, measurement. Something like that. I forgot the exact title, but it’s a well, well, well worth read. and basically they make the point and, that you need to combine these methodologies and that you shouldn’t look at to each one of these in isolation.
[00:36:10] János: and nowadays it goes, by the name of triangulation. And we as funnel, obviously, very much guilty of, putting that term out there as well and, promoting it. But the idea is basically that you not look at any of these methodologies, MTA incrementally testing in platform attribution results in isolation and combine them in a way That you have one number per channel and not like, as you said, you know, your last pick numbers, your numbers, your in platform numbers, but one number that, you know, as a solution, for example, we recommend you should look into, and, and that’s similar to, it’s kind of developing into this.
[00:36:51] János: Not best practice because it’s, again, might not be the best solution for everyone. And depending on the, you know, measurements, so sophistication, maturity, but it’s, it’s kind of, coming up there as, as the, as a way to go so that you combine different approaches to arrive at, you know, at one, one KPI per channel.
[00:37:10] Bhav: I did a piece of analysis, not too long ago, where I was comparing the different attribution models against each other. So I had the last click, the first click DDA, Facebooks and the, the, the platform that they were using was called Phosphor and their attribution model. And so I was actually comparing it and I arrived at a similar conclusion and I, you say it’s not for everyone.
[00:37:35] Bhav: I think it is for everyone in many cases, actually, because You’re always going to get bias from the marketing person who’s on the PPC channel, the platform that you’re using, the only way to overcome that bias. And I normally hate saying more data is better, but in this case, more data is better by having access to all of the attribution models per channel and the breakdown of them.
[00:37:54] Bhav: And having a look at sort of like a aggregated view of all of these channels will give you kind of a wisdom of the crowd approach to marketing spend optimization that will ensure that you are not waste, maybe not, we’re not wasting. There’s always going to be some level of like wastage in your, in your bucket, but you’re minimizing the way the waste I get because the challenge with.
[00:38:18] Bhav: And I think with the everyday marketing person. They’re never going to understand the concepts of like mean absolute percentage errors, right? Like that’s mate, by the way, for those who are wondering what mate is or R squared, which hopefully more people are more familiar with. They’re not going to understand these concepts.
[00:38:32] Bhav: So to be able to build this themselves is going to be a little bit harder. It’s going to be a little bit trickier. So the best way is to like take whatever data points you have and and create some type of aggregated view, which will allow you to remove bias and give, I believe, a more fairer view of the world.
[00:38:48] Bhav: Because that’s what we’re really trying to do here. We’re trying to understand the world as accurately as possible. We’re never going to get 100 percent accurate. So what do we try to do? And it’s, as you said, we try to minimize those errors. So I think it’s a great point.
[00:39:00] János: No, but, but exactly. You, I think you, you’re completely right on that. and, it’s, it’s, I mean, truth, like the truth that we, or what we see a lot is that, you know, there are some businesses out there that maybe are not best suited to do MMM because for example, they only do Google ads, right? You don’t have the biggest MMM challenge. If you just grow, starting to grow with, with Google ads, but you still need to be.
[00:39:26] János: Kind of understanding as soon as you want to invest into upper funnel type of activities, you should look into something else. The last click that’s what I kind of meant, but you’re right. That, I mean, the brands that we talk to, they have these challenges like, you know, different perspectives, different teams, looking at different numbers all the time.
[00:39:43] János: And we tell them, look, there’s a, there’s a consolidated way on a better way to do this. I mean, again, you know, trying to mitigate the disadvantage that you have with each methodology. and, you know, leverage the advantages that you have. So for example, for MTA, as we discussed, like it’s very much reduced and focus on the, on the lower part of the marketing funnel, right?
[00:40:05] János: You cannot, you cannot sensibly measure, like a, TV campaign or an out of home campaign with, MTA that like usually like, there’s not a good way to do that. and on the other hand with MMM, Again, it’s like based on a very limited amount of data. So it can be very volatile if you’re not doing it right.
[00:40:24] János: And, and then with incrementality testing, which is the way to try to, you know, prove causality, meaning nowadays how incrementality testing is usually done. You have one region, in, let’s say for example, Germany, you choose Bavaria. And say these, all the people in Bavaria are being exposed to your marketing activity out of home campaigns, for example, and, the ones in Baden Württemberg, another region or another state in Germany is not exposed.
[00:40:50] János: And then you compare the difference, sort of say as a test control group, just as a quick explanation there. So that’s great because it gives you very. sort of more causal understanding of the impact of your marketing activities, but it takes a lot of time. Like, you know, it’s nothing that you, you do the test now.
[00:41:06] János: And then in an hour you have the results that usually runs for, for days and weeks. Right. So that’s another disadvantage of incrementary testing. But on the other hand, it gives you probably the most, causal, understanding of the impact and the incremental impact of, marketing, specific marketing activities.
[00:41:23] Dan: Do you think that then is, is there a use for. These multiple different perspectives. I always, I always consider these different, measurement techniques as like lenses you apply on the data, right? They just tell you different stories and collectively you can make up the narrative and join the dots between them.
[00:41:39] Dan: Right. but I’m just thinking about this, like, they’re not real time. As you said, like sometimes they take weeks to run with incrementality tests. Sometimes they need weeks, years, days to process for the MMMs attribution is, you know, got his flaws and limitations, but can be a bit more quick and reactive.
[00:41:56] Dan: Right. The actual marketing tools themselves. Let’s take things like Google ads. They’re not going to plug into our MMM capabilities to then optimize the ads on a real time basis. And so they’re going to continue to run with their own modeling capabilities, their own voice perspectives out. The same can be said for TikTok and the same can be said for all of our other different marketing channels.
[00:42:15] Dan: So like where, where does this come in? I mean, we talked about adjusting budgets and, from a, the, the, the kind of CMOs perspective of kind of shifting the spend on a retroactive historical analysis project. But is there, is there anything beyond this? Like, should people that are, let’s say I’m a PPC manager right now, and I’m looking at this and I know it exists and I know it’s important.
[00:42:37] Dan: And I know what to do, but does it? Does it actually affect me? Like, how does something like this affect me managing my campaigns and keywords and spend on a daily basis?
[00:42:45] János: Yeah, great question. So I don’t know. So if we start at MMM, probably the, the impact will be more on the manager deciding how much the PPC manager should be allowed to spend overall on PPC.
[00:42:57] János: Right. So that’s kind of, and that’s a very specific type of decision that can be driven by MMM. And it can be operationalized in a way that, you We use MTA results. so as I said, MMM with a small data problem that it has, you usually shouldn’t do MMM on a campaign level because there’s like way too many campaigns, thousands of campaigns, but only like per year, you have 365 data points if you do MMM on a daily level.
[00:43:22] János: So there’s this mismatch, mismatch between number of input variables and the number of data points that you have, but we can use MTA results. Or in platform results to split up the MMM attributed value to PPC, for example, down to campaign levels. So for example, if we know multi touch attribution tells us like generic search campaign A has 30 percent and a generic search campaign B has 70 percent of the empty, like MPA conversions.
[00:43:51] János: then we can split up the overall MMM Numbers that we allocated to PPC down to these campaigns in that way. So that’s a very specific way on how to combine MMM and MTA. And then that information can be used by the PPC manager to say, Oh, this campaign overall is not creating that much incremental impact as I thought of.
[00:44:10] János: So I’m, you know, maybe reducing budget or, you know, increase the targets on that specific campaign. whatever the, you know, specific steering, strategy is in that case. One, one other way, and I, I always need to be a bit careful to not, you know, oversell or not to be too salesy on what we actually do at Final Measurement.
[00:44:28] János: But one way, and also other vendors do that as well. But one thing we do is we track Google click IDs. So the, you know, clicks, the Google click IDs are the clicks, the IDs on clicks that, happen on Google ad campaigns. And then we can give value to these particular Google click IDs and export them back into Google ads so that the Google bidding algorithm is able to Can use this more unbiased evaluation of the specific clicks.
[00:44:52] János: that’s nothing that the PPC manager is involved in on a daily basis because it’s an automated process, but we have 50 percent of our clients, of the, of the measurement clients using that for, for direct optimization of Google ads campaigns, like such a much more unbiased way to evaluate the, Google ad campaigns.
[00:45:12] János: and yeah, make sure that it’s based on a more holistic view because you, you account for other. Marketing channels effects and make sure that Google isn’t being overvalued.
[00:45:21] Bhav: So, so far, what we’ve talked about is it’s actually, we’ve actually gone pleasantly into quite a lot of depth on this topic, which I love, but I’m going to take it sort of like upper level, because I really think that there are going to be people who listen to this podcast and think, yeah, like you guys are absolutely right.
[00:45:39] Bhav: Like everything you said so far resonates, but we are not the people who make these decisions. So I want to ask you, Janusz, like when you, when you were going out to clients and you’re, you know, reaching people, how do you convince the marketing person who holds the budget to think about this stuff differently?
[00:45:59] Bhav: Because assuming most marketing managers or sorry, most marketing directors or CMOs, let’s assume they’ve come through the ranks of, I was going to say traditional marketing, but actually it’s been sort of like, 15 years since google ads has been a thing right that most people have seen that transformation, but they only know Last click or first click if you know if we’re lucky or maybe some multi touch or something like that, whatever How do you convince them to take on sort like this more sophisticated methods because?
[00:46:26] Bhav: What we’re talking about is going quite in depth into like statistics and that kind of like prerequisite knowledge that’s required to understand the mechanics of what you do. How do you translate that to a marketing director who only knows? And first click.
[00:46:41] János: Yeah, good. So, we just had a, kind of, call that was like, yeah, say it’s called, that was very much related to that situation where the marketing manager was, so they do significant TV ad, campaigns and, he was basically.
[00:47:01] János: It’s true to be told most of the times is I’d say probably 80, 90 percent of the time the marketing managers are already aware of this challenge that they are, they know that they shouldn’t like, not that they shouldn’t, but that they’re probably not fully understanding the impact of the marketing, upper funnel marketing activities, and that could be social prospecting or TV or any other sort of upper funnel activities or branding campaigns, but they might not be Know about the ways on how to do it.
[00:47:30] János: That’s more, more often the situation that we encounter to be very honest. And that’s different to 10 years ago where we needed the Mario Götze example to get people away from last click. And now marketing managers also through all the changes, GDPR, tracking restrictions, they kind of understood that that’s, that’s what I would.
[00:47:48] János: Yeah, probably want to put out there. So it’s not that we need to convince people that they need to understand the impact of the upper funnel activities because they already doing it. Right. So they, they somehow doing things right, even though they cannot fully quantify it, that that’s, I think that’s a good message to be put out there.
[00:48:06] János: Nobody is just doing Google ads because they, that’s the only thing that they can measure properly. so, but, but then, and then what we start to explain is actually really going into the. Nitty gritty of it trying to explain that what MMM is, you know, how it looks like we try to explain them how the whole modeling process, because the next thing they ask is, yeah, but how do I know this works?
[00:48:27] János: And you just not made this thing up. And, maybe, I don’t know, Dan, you remember this, the, the other keynote that we had, in London, the Andrew, he did an awesome presentation. He said, what was that about MMM? this is fucking made up. That’s literally what he said. MMM is. Who thinks that’s motherfucking made up?
[00:48:46] János: So, I hope we allowed to cuss on this, otherwise just beat me out there on this F word, but that’s what he literally said. So I’m just quoting him. So, and, and, you know, that’s the thing with, MMM and these more seemingly advanced methodologies. You need to get people involved and help them understand it.
[00:49:01] János: So the, I think the short answer is educational efforts. That we as vendors and players in the industry, need to invest in. And, so we really need to, you know, we planning to have some training sessions. I think Google’s playbook was awesome to put it out there. The, the work that you guys are doing with a podcast, these are all things paying into that because nobody’s going to buy a solution in the long run or, you know, Invest into an approach that they don’t fully understand.
[00:49:29] Bhav: Well, maybe let me phrase it another way. Should we make most CMOs and marketing directors redundant and replace them with, with scientists is because marketing is different now, right? Like there’s the creative aspect of marketing, of course, right? But then there is the measurement aspect of marketing, which in itself is a full time job, understanding.
[00:49:53] Bhav: How to spend where, you know, et cetera, et cetera. And ensuring that you are senior enough to make those decisions, right? Like, let’s say you’ve got a really creative CMO who doesn’t understand like diddly shit about, MMMs and things like that. And they continue to pump up money into some creative campaigns, which are probably doing some type of a job, but actually it needs that kind of.
[00:50:15] Bhav: Numerical mind for lack of better word, to be able to translate what you’re seeing in raw data. And understand the difference between causality and, you know, all of these correlations and make these informed decisions where should we scratch, should the CMO be replaced with like a chief data officer?
[00:50:35] János: I think it wouldn’t sell. I’m being contentious here. Yeah, no, I, I think you touch a point of, touch upon that. That’s very, interesting. What we generally see is that marketing managers. overall get much more technical and much more, you know, quant like people. So they understand concepts such as, well, ROI, of course, but then also the more, you know, machine learning and statistical KPIs.
[00:51:00] János: that that’s, that’s something that I see at least in the last decade shifting quite dramatically. Replacing the CMO, again, that, you know, that’s the one that’s been Supposed to buy our solution. So I’m not going to, confirm that that wouldn’t sound well. No, but, I, I think like also, also in that regard, we see CMOS wanting to learn about that and getting more technical and, yeah.
[00:51:26] János: And of course there’s this natural fluctuation with CMOS, you know, some going into the well deserved, sort of, How’s it called a pension time and, and, and maybe more technical people come up. And maybe if the organization decides who should be the next CMO and organization, should it be the, I don’t know, brand director or should we, you know, maybe use the performance marketing manager who’s used to these sort of discussions around modeling.
[00:51:51] János: maybe more so. I think that that’s kind of the way we, we, yeah. So, but, but it’s an interesting point. And of course, yeah, this all needs to be supported by us as the industry in terms of educational efforts.
[00:52:06] Bhav: we cannot just hope for, I was gonna say, I’d love to understand what the decision was happening. I don’t know if you’ve been following the Jaguar, ad campaign recently where they completely, you know, that was a through and through creative brief, but I wonder if there’s a, there’s a, there’s someone who, you know, the man behind the curtain. Or the woman behind the curtain, who was like, let’s do something outrageous because like numerically, this is going to add up. And then they, so I would have loved to have seen
[00:52:32] Dan: how do we go viral? Here you go. You figured it out.
[00:52:36] János: How will we get as many, comments hating on us?
[00:52:40] Dan: Hey, all publicity is good publicity, right? yeah, I’m sorry to be this person, but I seem to be every episode, but we are running quickly out of time and I want to respect your time and our time.
[00:52:51] Dan: And I want to kind of get us towards wrapping up towards an end. I wanted to, before we wrap up and start doing this, a couple of questions, I want to throw at you a bit of a quick fire question, unfairly, cause I haven’t told you them ahead of time. is there, this is the moment to plug, funnel, funnel measurement and the tool itself.
[00:53:08] Dan: is there anything that you want to plug anything you want to say? Any links you want to throw out there that I can put in the show notes?
[00:53:15] János: no, I mean, overall, generally speaking, what, what, what’s so interesting about this acquisition of, Atriba as a measurement company by, funnel data, is that we have been partnering for quite a while because we knew it when we dove into, you know, MMM, when we, when we really explored MMM starting in 2018, also due to GDPR, we thought really quickly about, okay, where do we get all this aggregate data from?
[00:53:40] János: the most efficient way. So we looked at different, you know, data hubs, so to say. and, we started partnering with funnel, end of 2019. So before the merger, 80 percent of our clients were already using funnel. So it was a very natural combination. And, until this day, you You’d probably know the other players out there, such as, what’s the data Rama at Verity Supermetrics.
[00:54:04] János: None of these have an MMM solution, or, you know, triangulation solution for that matter. So that’s, I think the shameful plug here, that, you know, this, the solution, I think quite interesting because it’s not just about the algorithms and machine learning and the whole data science, the, the even more, you know, Almost more important part is the whole data generation integration part.
[00:54:25] János: And that’s what funnel data hub is so great at. So I think that’s, yeah. again, I think quite an interesting development then. And we, you know, we like competition. so we, we, very excited to see what the other players are doing in this space, but the combination is I think quite interesting to go, check out our website, there’s a lot of, amazing content out there.
[00:54:47] János: We have, measurement academy out there. If you want to test that, we’re gonna, put a lot of measurement content out there. there is, there’s also some, beginner tutorials for all of these, topics on YouTube that we just recorded, which are, very on a high level. So not too, too much talking about maybe and all of these things.
[00:55:09] János: Yeah, that’s, that’s it. And then we’ll also gonna have a podcast and I’ll hopefully be able to invite you at some stage, which we’re going to restart in January. marketing measurement matters. there’s some episodes on Spotify. yeah.
[00:55:22] Dan: And, that 95 percent discount code is. , 42, of course, love it. well look, thank you Yash, for that. So now time, for a quick rapid fire, wind down, section. so, three questions and then you’re off the hook and, you can get back to, whatever you would, we interrupted you doing today. So, if, you was to give, some advice to someone starting out on their marketing measurement journey today, let’s say it’s a, a team, an organization, a startup, whatever it be, what would that one piece of advice be?
[00:55:55] János: Go read Google’s measurement playbook. I think that’s a good start. It’s a, we need more like the, the standards and then, also feel free to check out our block on funnel measurement, but, for a more unbiased, recommendation here, I think that’s a good start.
[00:56:10] Dan: Looking ahead, what do you think will be the biggest shift or trend in the world of MMM and MTAs over the next five years?
[00:56:18] János: Over the next five years, I think, MTA and tracking will go through sort of Renaissance. People will realize that yeah, cookie, some cookies will die. Not all of them. Of course, that was a big flaw in the thinking there and that we’ll see, yeah, Renaissance of MTA and, and tracking, becoming more valuable again.
[00:56:38] Dan: Amazing. Yeah. I haven’t heard that. That’s really interesting. I’d love to dig into another time. final question, outside of the world of MMM, MTAs and measurement, what do you like to do outside of work to wind down, to escape everything?
[00:56:48] János: Ah, that’s easy. Kite surfing, kiteboarding, wing folding, everything that’s on the water. That’s amazing. Like that’s total freedom outside of any thought of algorithms and restrictions, privacy, or, you know, tracking related, yeah, water sports. And of course, spending as much time as possible with my three kids.
[00:57:03] Dan: Amazing. Well, thank you so much for joining us on the podcast. everything you mentioned, I was furiously typing, but I’ll add some more in, all the links will be in the show notes, including ways to connect with you is LinkedIn the best way to connect to you, Janosch, if anyone’s listening, wants to connect, or is there any other ways they can get?
[00:57:18] János: Yes, definitely. Feel free to connect directly on LinkedIn, either through private message or, I mean, also of course, through, funnel’s website, but LinkedIn is fine. Cool. Perfect. Perfect. All right. Well, thank you so much.
[00:57:30] Dan: That’s it for this week. Thank you for listening. We’ll be back soon with another episode of The Measure Pod. You can subscribe on whatever platform you’re listening to this on to make sure you never miss an episode. You can also leave us a review if you can on any of these platforms. We’re also over on YouTube. If you want to see our lovely faces and our lovely guest faces while we do this as well, make sure to subscribe to the Measurelab channel to make sure you never miss an episode as they come out.
[00:57:52] Dan: If you’ll leave us a review, that’ll be hugely appreciated. You can do that on most of the podcast applications, or there is a form in the show notes. You can leave feedback directly to me and Bhav. Thank you for listening and we’ll see you on the next one.
Subscribe to our newsletter:
Further reading
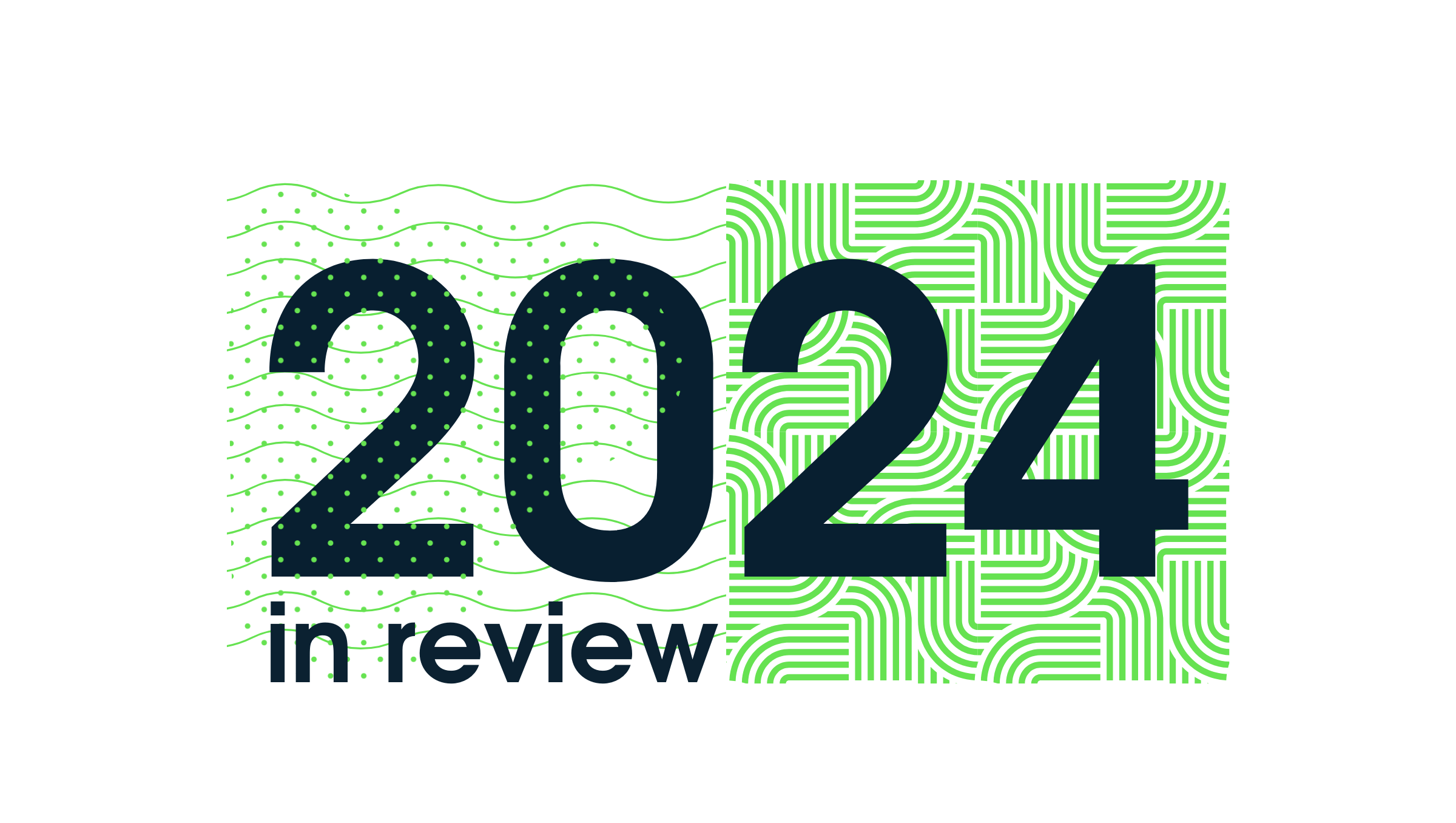
2024: Measurelab’s year in review
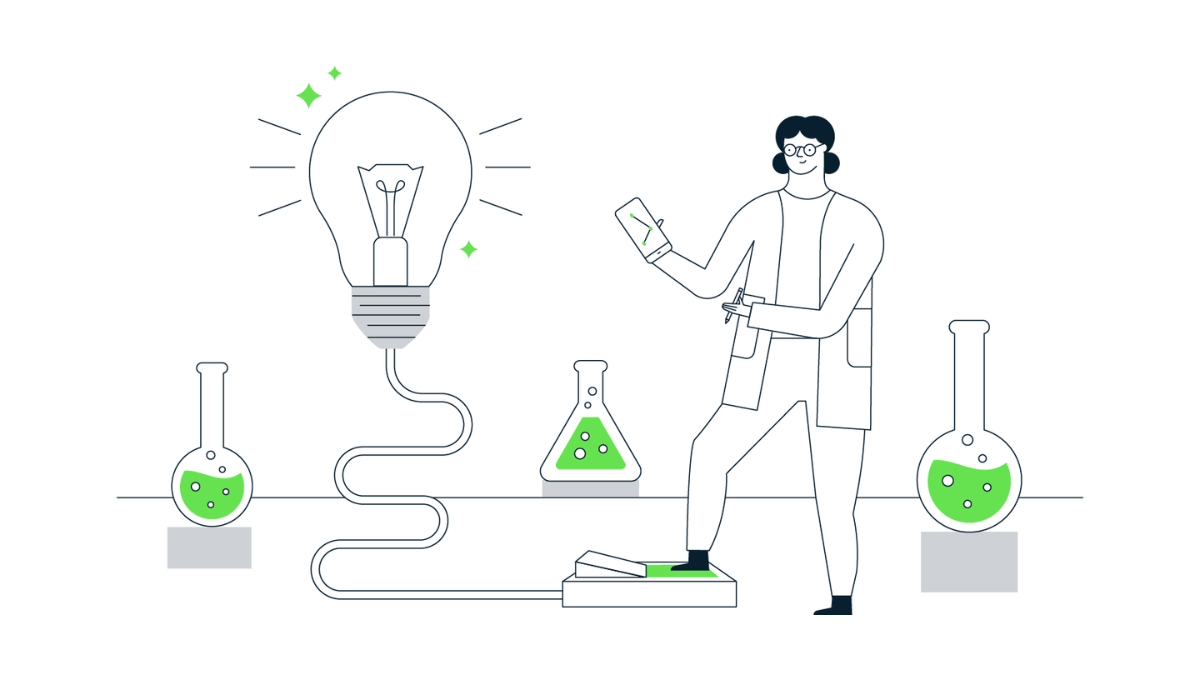
A step-by-step guide to migrating scheduled queries to Dataform
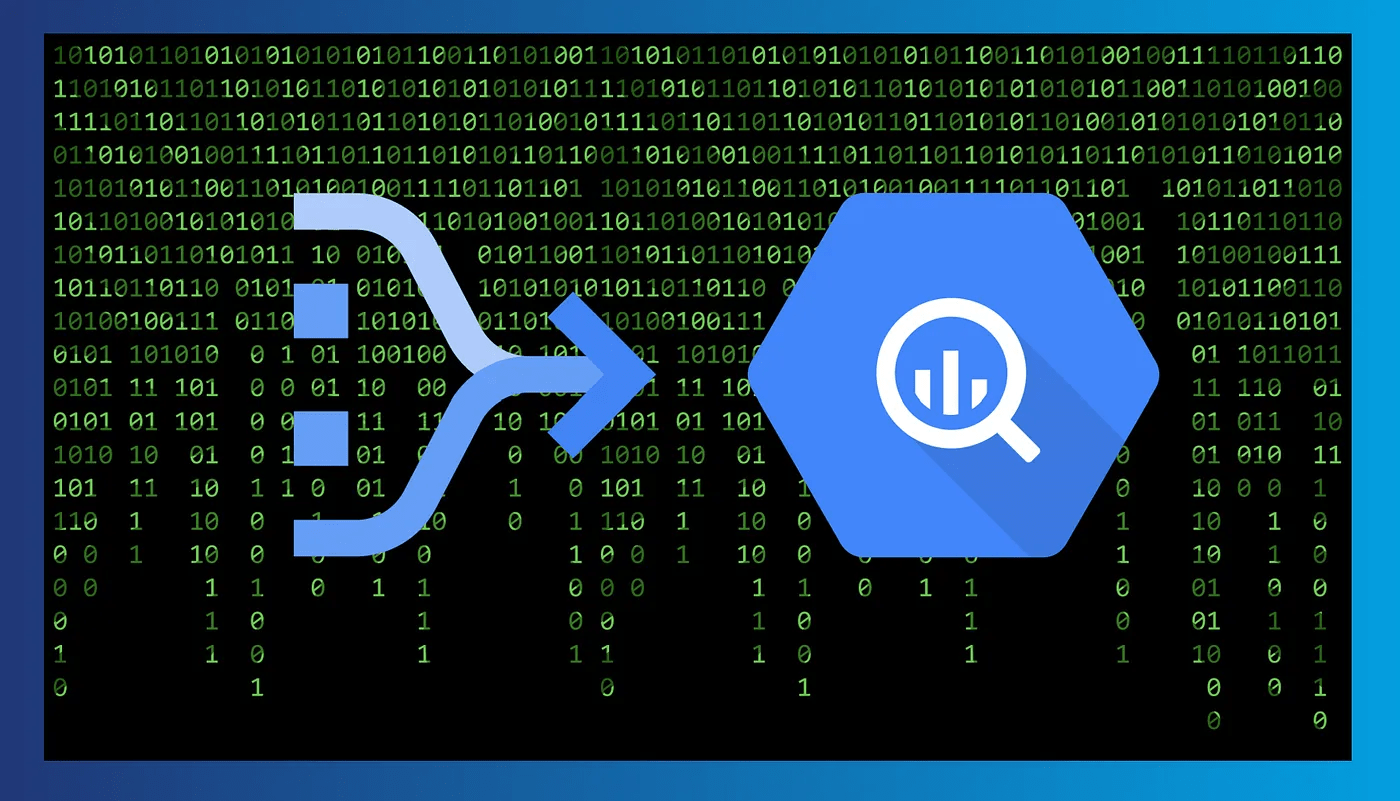