#40 What is the modern data stack? (with Mark Rittman)
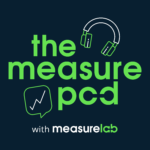
This week Dan and Dara are joined by Mark Rittman to talk about the ‘modern data stack’ and how the danger with all analytics implementations is the ‘so what’ factor. They also touch on how the changes in Google Analytics 4 are changing things for the modern data stack, and what type of company would truly benefit from a fully-fledged setup.
Check out Rittman Analytics – agile analytics consulting for the modern data stack – https://bit.ly/3LTE9oV.
Mark’s Medium article on his Rittman Analytics’ modern data stack setup called “How Rittman Analytics does Analytics Part 2 : Building our Modern Data Stack using dbt, Google BigQuery, Looker, Segment and Rudderstack.” – https://bit.ly/3wRFOXO.
Check out Mark’s (pretty epic!) DJing music on SoundCloud – https://bit.ly/3PIYMap.
In other news, Dan MeasureCamps, Dara swims and Mark cycles and makes music!
This is the last episode in this run, 40 episodes can you believe it?! See you all in a few weeks after a short break!
Check out on LinkedIn:
- Mark – https://bit.ly/3PLkdI0
- Dan – https://bit.ly/3JQKHEb
- Dara – https://bit.ly/3vzV0bO
- Measurelab – https://bit.ly/3Ka513y
Music from Confidential, check out more of their lofi beats on Spotify at https://spoti.fi/3JnEdg6 and on Instagram at https://bit.ly/3u3skWp.
Please leave a rating and review in the places one leaves ratings and reviews. If you want to join Dan and Dara on the podcast and talk about something in the analytics industry you have an opinion about (or just want to suggest a topic for them to chit-chat about), email podcast@measurelab.co.uk or find them on LinkedIn and drop them a message.
Transcript
[00:00:00] Dara: Hello, and thanks for joining us in The Measure Pod, a podcast for people in the analytics world. Welcome to episode number 40, believe it or not. I’m Dara, I’m MD at Measurelab. I’m joined as always by Dan who’s an analytics consultant also at Measurelab and we’re very pleased to also be joined today by Mark Rittman from Rittman Analytics. So hi Dan, hi Mark. How are you both doing?
[00:00:37] Daniel: Yeah, good thanks.
[00:00:38] Mark: Very good, very good to see you here and to speak to you so I’m glad to be on the show.
[00:00:41] Dara: Thanks for agreeing to join us Mark, we’re keen to have a good chat with you, but before we get into the detail, what we usually do is ask people how they got into analytics and data in the first instance. So this is your chance to wonder as much or as little down memory lane as you like really just to give a bit of an intro into who you are and how you got to where you are today.
[00:01:01] Mark: So I suppose unlike a lot of guests that you have on who all seemed to kind of ended up by accident working in analytics. It’s been something I’ve actually been interesting now for about 25 years now. So I know I don’t look that old, but yeah, but 25 years. I had a more convoluted route into technology in that I did computers at A-Level, O-level, did a year of a degree at Brighton University. And funnily enough I did it for a year and then I dropped out I didn’t want to do computers anymore at that point, I’d had enough of it.
[00:01:29] Mark: So I dropped out and I went to do a business studies degree, worked for about 10 years at like an actual bank years ago and worked as a kind of a branch manager and all this kind of stuff on a graduate scheme. Then I heard about this project that was going on at head office that was to do with marketing and online marketing, but particularly it was a project to do with kind of Oracle, and I heard enough about Oracle to know that was an interesting area to be in. I went to work in head office, got involved with this Oracle project, which was around data warehousing.
[00:01:56] Mark: And so after this kind of 10 year hiatus really away from technology, I ended up on this project and then from that point onwards went to work in consulting and then eventually set the business up now that we have. So it was a kind of like a planned route, but I suppose there’s a little diversion at some point really into the world of kind of business. But actually when you work in analytics, having some understanding of the business and the context of it is very useful, it’s not just about technology.
[00:02:18] Dara: Absolutely right, and you’re also a fellow podcaster and Dan was actually on your podcast Drill to Detail recently, do you want to give us an overview of your podcast as well, while you have the chance to plug it here?
[00:02:29] Mark: Yeah, sure. So it’s a podcast I have been running for about six years now. So the podcast is called Drill to Detail and it was originally something we set up as a vehicle to better talk to people that I found interesting. If you just said to somebody, can I speak to you on the phone for an hour about what you do, they would think you’re maybe a stalker or kind of, you certainly would be an interesting conversation, but you can approach people to be on a podcast and it’s kind of normal. So it’s really about, I suppose, the people and the technologies, and I suppose the ideas that are driving analytics at the moment. So it’s a bit more focused on the technology side of what we do and a bit more focused recently on what we call the modern data stack. But yes, we had Dan on there recently and, you know, it’s very good to have you on there.
[00:03:06] Dara: And you mentioned the modern data stack, which leads us nicely into our topic for this week. So we’re really keen to dig into this with you to understand your thoughts, your approach, some of the tools and tech that you use as part of what you would call the modern data stack. So with our background in GA (Google Analytics) and the GMP (Google Marketing Platform), and we’re thinking about how that fits into a data stack, you’re coming at this from a broader perspective, I guess. So our worlds do crossover, but it’d be interesting to maybe explore a little bit about the differences between them and also potentially how tools like Google Analytics fit into the overall picture of what you’re working with as well.
[00:03:40] Mark: So the modern data stack, maybe it’s worth us defining that first of all, what you mean by this really. Like a lot of things, it’s partly kind of relevant, partly, you know, a set of buzzwords that people can make into whatever they want it to be. But taking a sort of step back in history really. So when I first started working with analytics tools and analytics platforms about say 25 years ago, you would go out and you would buy your analytics suite products. Typically from one vendor and it would be a big implementation of say, I don’t know, Cognos or Oracle or whatever, you’d buy all your products from this vendor. It would all be potentially kind of pre-integrated, but it would be a big-ticket investment. It will be hosted and run on premises and it would be something that would be maybe owned by the IT department and more technical, I suppose, than user-friendly and approachable.
[00:04:25] Mark: So like all technologies and like all parts of industry, things go in cycles and what’s happened over the last five years or so is that rather than buying all the stack from one vendor and everything being a big monolithic suite of applications, like a lot of things in technology the stack in this case has been, I suppose broken up into various parts really, and made more user friendly, hosted in the cloud and generally moving towards the sort of thing that a non-technical person could implement and work with as opposed to being an IT thing. So you know, being very specific about what a model data stack is. There’s a few components in there that are fairly sort of common really, and one way of looking at it saying, well, you’ve got, for example, in there a fully managed ELT data pipeline that’s one part. So a tool like for example, Fivetran, or maybe even just the ability of say Google Analytics to export directly to kind of BigQuery. You’ve typically got a data transformation part to it and there’s a technology or tool that everyone uses now called DBT, that’s a key part of this really. You’ve also got in there, the cloud-based columnar data warehouse, so in your world that might be BigQuery.
[00:05:29] Mark: You’ve also got things like Snowflake or even Redshift, for example. And there’s a data visualisation part to it, and the tool that we use a lot and actually again in your world is quite common, is a tool called Looker. So it’s really breaking what would be in the past a big suite of products into a set of components, making them user-friendly hosted in the cloud and very easy to get working with.
[00:05:48] Daniel: Does that also afford you the ability to kind of swap them out if you need to, because it’s all a modular process now you’re not contracted into a single platform. So if you needed to throw in Power BI alongside, or instead of Looker, at some point in the future, you don’t have to rebuild your entire architecture to do so.
[00:06:03] Mark: Precisely, yeah so very well said. So another key part of it is the modularity of it, so if you find that, for example, the data pipeline that you’re using now is not maybe what you want to have in the future, you can swap it out. Everything there is meant to be replaceable, modular and so on. So yeah, absolutely the modularity, but the fact that you’re not tied into one particular vendor but what we’re finding is even within one category of a product within that stack. So if you think about say data visualisation, a trend that’s happening now is saying actually even the visualisation part, can that be broken up into its component parts?
[00:06:34] Mark: So, I suppose one of the analogies is with the Unix world, where you have individual tools that do one job and one job only, and you can swap those tools in and out. But the idea is that rather than you buying into everything, you can just buy into the modules you want and then actually, as you say, you swap them out in the future, but that does come with its own costs and its own overhead. You’ve then got the job of integrating these together, which is the other side to it really.
[00:06:56] Daniel: Can I actually pick up on something you just said there Mark and I’ve always been quite interested on this. And that’s the, maybe start with the definition of what a metrics layer is and why is it only recently it’s being talked about everywhere. I look on LinkedIn and all these blogs, but what’s the focus and where has it come from?
[00:07:11] Mark: So the idea of a metrics layer is to have a single place that is typically vendor independent. Where you define your metrics and the relationship between them, so the dimensionality of your metrics or the hierarchies and so on there, I guess it’s probably in contrast to where you are now really. So a metrics layer is in some respects, think of it like a semantic model or it’s the business meaning of metadata and definitions over the data that sits in your warehouse for example. In the past that’s been handled by business objects or tools like that. Recently Looker for example, have the sort of data layer, data model where you define the metrics layer but you do it in the context of a particular tool. And the advantage of that is that it’s all part of one combined experience but the disadvantage is obviously it only really works with that one tool.
[00:07:55] Mark: So the metrics layer is like to say, can we take those definitions of metrics and measures and dimensions and so on, and put them into some form of layer or whatever that is independent of tools, and then the BI tools then become consumption layers for the metrics layer really, you know, that’s the kind of thinking behind it. Where it’s quite interesting is that there’s now several competing visions of what a metrics layer could be, and there’s also, what is the current definition of a metrics layer is really, you know, knowing how this thing can really kind of where it can go to is a very kind of primitive version of where I’ve seen these things go in the past. So there’s almost an element of rediscovering things that actually have been there for a long time. And the other part to it really is with a metrics layer, it does depend on the BI tool that’s actually adopting and using this. Where’s the incentive for a vendor to adopt a metric layer that makes their actual BI tool effectively disposable or modular, but the idea is to have a central place to define these things that can be used by lots of tools.
[00:08:48] Daniel: Sorry, I just want to pick into this it’s really interesting. So is this where Google are positioning Looker to kind of feed into Data Studio? Maybe you’ve got a metrics layer and the kind of consumption there because I know they’ve developed a connector from Looker to Data Studio, and I was always thinking about what the value of connecting to basically visualisation layers or BI tools are. I’m just wondering are they kind of positioning Looker to be an independent metrics layer?
[00:09:10] Mark: So if you’ve got a BI tool or some kind of visualisation tool that uses a metrics layer that is rich enough to be usable beyond that one tool, then your dream as a vendor is to have that tool, have that metrics layer adopted by the tools because then license wise, typically, if you’ve got a bunch of people you want to use Data Studio or the strange announcement that came out a while ago, which was that Tableau would also link in with this. So this is, Lookers play in a way to own the metrics layer space by reusing lookers one, but now the advantage to Looker is that typically for every user of that metrics layer, they have to have license for Looker. So it’s not like they’re providing this out of the goodness of their heart, it allows Looker to monetise users of BI tools other than Looker, who are making use of your definitions of dimensions and metrics and so on.
[00:09:52] Mark: So you can see why the vendor would do that as a kind of customer of this there’s that kind of classic thing of saying, I want to have a single version of the truth. The best way to do that is to have all different tools using those same definitions. But again, I think you’ve got to think about the cost of it if you’re going to do this, you need licenses for Looker for all these parts, but also, you know, if you’re going to start to have an independent metrics layer is it better to do it through Looker or is it better to do it through a sort of maybe an open source sort of framework for doing this, but then of course it does rely on those tools actually adopting this really.
[00:10:21] Dara: So that is interesting what you said about Tableau using the Looker metrics layer. So surely for them wouldn’t they be better off developing their own version of that metrics layer because otherwise the customer has to have Looker licenses, but then also Tableau licenses as well.
[00:10:37] Mark: In isolation, it’s a good move for both customers and for all the people involved. So I suspect the way it will work is that Tableau will just be able to connect to what appears to be a database connection, but it would present Lookers explores and views and so on as, effectively as database tables and joins and so on there. So if as a business you’ve defined a metrics layer / business data model, and your users to want to carry on using Tableau because Tableau is a better visualisation experience. It’s better that they connect to the central definitions of what these metrics are as opposed to their own, because the big problem with Tableau in my view is each person has their own take on how these metrics are defined.
[00:11:15] Mark: So it makes sense from an organisational point of view, but I wonder how the licensing would work out because they would need to have, I would imagine at least have Looker licenses for those as well. And that’s where you start to say, is it better to use your warehouse as the central definition of these measures. One take of the metrics layer is the DBT Labs version of that, where it’s not only definitions of, these are the measures, these are the dimensions that it connects to, but start to define what I called like second order measures. So things like year over year definitions and so on. And, you know, is it better to connect to that than to connect to a vendor’s proprietary one.
[00:11:48] Mark: I suppose, this is the example of where it gets interesting in the modern data stack world, which is pretty soon, it can get fairly complicated and you can imagine in time, someone will come along and say, why don’t we pre-integrate all of these things into a suite? And there you go, back in that circle again really. But yeah, these are the kinds of debates that are going on at the moment really within the modern data stack world.
[00:12:07] Daniel: So that leads quite nicely on to my next question, Mark, which is considering all that and the level of complexity you can go in optionally, even if it’s wrapped up within a single suite of tools, it’s still going to be pretty complicated and pretty multifaceted, especially in the skillsets and the technology stacks that you’re using, what kind of companies would be looking to adopt or implement a modern data stack. So who are the people that would benefit most from that, and then, is there a kind of case where some companies you’d almost advise them not to because they might be biting off too much more than they can chew.
[00:12:36] Mark: It’s interesting, so actually on the podcast episode that you came on last week, you made a good point about building your own attribution models and although it is possible to do that in many cases, it makes sense to just use the models that come with GA (Google Analytics) for example, other cases, when you’ve got the resources, when you’ve got a data engineer on team, you’ve got the ability to invest, not only in the short term, but overtime, in building out your capabilities in that area, that’s where it makes sense. So as a business, we think about who our ideal customer is. And in the past, we’ve actually worked with a lot of startups, a lot of very early stage, seed level, seed funding level startups and work with them on building out modern data stack platforms.
[00:13:13] Mark: In practice I find that there’s a point at which you’re too, not too small, but when there are better ways to spend your time, really, and I would say that maybe a marketing team with no IT support. Arguably you know, is it really worthwhile to be ingesting data yourself, loading into BI tool, yourself, modelling it yourself and so on. If you’re a seed stage business, are you better off using the reporting that comes in Amplitude for example, or comes in GA (Google Analytics)? I tend to think businesses that are at say series A series B level where they’re starting to actually properly invest in analytics, that’s when it makes sense. When there’s a point to building a data warehouse, when there’s the ability for you to think of it as being a journey, not a one-off project. That really for me is where it makes sense, but certainly it does take some investment. And even though the parts are largely fire and forget, and they kind of work as they are, you still need to think about what is your data model, how you can integrate data and so on.
[00:14:06] Mark: So I’d say there is a point at which it’d be too small to do it, and you’re better off using things that you provide, for example. But certainly I think once you start to invest in people for your data team, that’s where it makes sense.
[00:14:17] Daniel: Yeah, it’s always going to be about proving that return right, of the investment in especially technology or data. It’s that kind of trying to justify or measure a return on your analytics investment or data investment, but whether that’s to employ people or employ technology to do that or consultants to use the technology. But I think if they’ve already made that jump into investing in people I think then the technology, it’s just enabling them to be better and faster and more powerful. So I think in a sense you’re kind of, you don’t have to convince them that this is worth investing in. They know that, and then it’s just expanding and accelerating that, trying to convince them that investing in technology in the first place is probably a waste of your time.
[00:14:53] Mark: I think there’s something that we haven’t really mentioned, which I think is a key part of this. We talked about like, ELT data warehouses etc., but the other part to all of this really is the idea of analytics being a branch of software development. So you said a minute ago about if you are hiring people in, then it makes sense to think about this kind of stack or whatever, I would argue that the most impactful thing that you can do, if you are starting to hire people in to do your analytics, to actually to export data from GA (Google Analytics), for example, into kind of BigQuery, think about how are we going to understand the data and so on is to think about, I suppose, the structured way to start analysing that data to be modelling it.
[00:15:29] Mark: And rather than it just being a bunch of say scripts in a directory or a bunch of Google Sheets and so on. There’s a framework called DBT (data build tool) that we will tend to use now, which treats analysing data as something that has a process, you version control with the work you’re doing, all of the transformations that you do and analysis is linked together in what’s called a sort of a graph of queries. The point is, if you can approach analysing your data in a structured way, that’s the most important thing really. It’s not so much about whether you’ve got Fivetran or you’ve got whatever, whatever. It’s about are you building up your analytics assets and are you working in a way that is testable, is version controlled and actually is repeatable as well? And that probably applies as much to the work you do really as well as anything else. And so I think it’s the combination of these modular tools, plus treating analytics as a branch of software development, that’s the kind of big innovation in this area really.
[00:16:18] Dara: Does DBT, does it play nice with all of the kind of tools that you’ve mentioned Mark?
[00:16:23] Mark: So if you think about, for anyone who hasn’t heard of this before, so DBT, it’s an open source, toolkit and framework that is a way of structuring queries that you write using SQL, for example, against maybe your kind of GA (Google Analytics) data or whatever, really. So where it really fits into the workflow is that you would use a tool or service, like say maybe GA’s (Google Analytics) export to BigQuery. That would land data into your data warehouse in a kind of raw unstructured format, and you then need to kind of reformat and restructure that data to actually then analyse it in a certain way, maybe breaking out sessions or users into a certain table and so on.
[00:16:56] Mark: So DBT would be the transformation stage of what’s called deep extract load and transform (ELT) workflow. And so it can be something that you trigger yourself, it could be run automatically by maybe a scheduler or something. So the point is it accesses the transformation stage in what you do. Now actually within the Google world, it’s a very similar approach of, very lightweight way of structuring, like the queries that you write. And I suspect that in the future, we’re going to find that this DBT like acquisition that Google made, plus Looker plus BigQuery, it’s going to be maybe their take on how we can analyse data in this lightweight way, but in a way that scales up and is modular and fits together and so on.
[00:17:33] Daniel: But I think then we’re back in the danger of going into that process again, of like putting them all under one roof and then breaking them apart, it’s going to be harder again. So is this another inevitability or do you see that being different this time around?
[00:17:44] Mark: So to answer your question I would say that I think it’s inevitable that there is going to be some bundling again of this. So, first of all, I think for a purely vendor strategy side, there’s lots and lots of vendors in this space now. If you’re an analytics vendor or BI tool, data pipeline vendor, you obviously say that your product is now part of the modern data stack. And you look at the ecosystem diagrams and it’s hilarious how many things are in there and there’s like sub categories of categories. So I think on a purely practical level, you’re going to get consolidation in that space because what with the way the economy is going now, maybe the tech bubble bursting and so on, it’s not going to be quite so easy to get funding and you’re going to get a lot of vendors by the vendors. So I think that’s going to happen as a consequence of just normal vendor kind of strategy, but then you got to say to yourself, really, what is the overhead in trying to stitch all these things together?
[00:18:32] Mark: So our consulting business, my own business, you know we make a good living building and integrating these modern data stack systems together for customers. But what people don’t seem to realise is I suppose the human capital cost of that. So if somebody comes along and says, actually we can pre-integrate a lot of these and there are vendors out there that say, let’s take maybe the data pipeline from Fivetran, put it all together within a, sort of like a package solution, that I think would have a lot of appeal, really, because not everybody can afford to have a data engineer and everyone wants to be a data engineer. So I do think that there is space there for packaging these things up, and it’s just a natural cycle of things you’re going to get this consolidation at some point.
[00:19:08] Daniel: All right, so I think I’m going to have to ask Dara, how does a Google Analytics tie into all this? So we mentioned Google Analytics and maybe some of the connectors it has with BigQuery, and I think quite obviously it’s like a data source within the kind of wider modern data stack. Obviously we are in mid transition to the new version of Google Analytics, Google Analytics 4 is round the corner. So I’m not expecting you to answer loads of technical questions about GA4 but has that already started to make ripples in the modern data stack side of things? Or is this almost like a tiny little conversation happening in the corner and people like me and Dara making a big deal out of it.
[00:19:40] Dara: Is it just big news for us?
[00:19:42] Mark: So if you’re asking my opinion on that, so what’s the relevance of what you do and what we do. So I think on a very basic level, the tools that I tend to use, day-to-day building modern data stacks are often the same tools that you are using. So we were using Looker, we use BigQuery, you use BigQuery. So I think there is, and obviously GA (Google Analytics) exports to BigQuery. And so one of the easiest projects for us to do is to turn on the export to BigQuery, take a, maybe a Looker block, that comes from Looker. So a pre-packaged sort of data model and series of dashboards from Looker. And we can get some quite rich analysis of GA4 data in kind of Looker pretty quickly.
[00:20:14] Mark: One of the most common questions people want to ask from our customers is what is our customer cost of acquisition and what is our return on investment on sort of our activity really. And so bringing in, I suppose, the marketing interaction data from GA (Google Analytics) bringing it in, I suppose, the ad spend data from Google Ads as well. All of that into say BigQuery and then bring it together in the form of a DBT model with Looker dashboards and so on, it’s quite often, GA (Google Analytics) data is the starting point for projects that we do. I think where we’re different to you is you would be using the modern data stack to further enrich and enhance and analyse data from GA (Google Analytics), maybe bringing in some additional data, some additional maybe context or whatever, but it would always be the context of trying to better understand the behaviour of your visitors and activity.
[00:21:00] Dara: Just to go back to something you’ve talked about a couple of times, which is Looker, what type of business? Because you mentioned that we, for example, we would sometimes work with Looker. Typically we tend to be working with maybe power BI or Data Studio, Tableau as well. What kind of business would you say needs Looker versus one of the other visualisation, BI tools?
[00:21:20] Mark: So, so putting this in context, then we’ve got maybe the first BI tools that maybe your customers might use, or with marketing context will be Google Data Studio or Power BI or tools like that, and they’re great tools for an individual person gathering some data together and then querying it themselves for their own purposes. But generally it’s a single person who doesn’t really mind that their take of data might be slightly different to someone else in the company, but it’s so they can get insights and answers to that data. So where Looker comes in, so Looker’s genesis really was kind of understanding that you’ve got a lot of online businesses now where the only interaction, the only kind of contact they have with their customers is through data really. And so that is a common thing across the whole company.
[00:21:59] Mark: It’s important that everybody understands everybody has the same view of that data is able to share definitions and so on. So Looker really is for businesses that are, want to go beyond individual people analysing data, to have a shared view of what the definition of your business is. So in practical terms, the cost of it is typically in the kind of five figures, right? So we normally say to customers need to have a budget of £50k or whatever to use Looker. So Looker is still priced on that basis, not on the usage. So there’s a kind of like a minimum affordability level, but it’s really for businesses that see the value in having a common understanding of the data across multiple departments, as opposed to individuals trying to answer their own individual question just for themself.
[00:22:43] Daniel: So Mark, actually, I saw you post on LinkedIn was it yesterday even, that you wrote up on Medium a really detailed exploration of your own company’s implementation of the modern data stack. So, yeah, I mean it was just because I saw it last night, I thought was really interesting so tell us a bit about that.
[00:22:57] Mark: So it’s been interesting, the feedback to that. So my intention with that was to show in practice how a non-trivial implementation of this could look really. So one of the things that’s interesting about reflecting on that is really what this is, is building a data warehouse. You know, as much as we say, the modern data stack is this new thing and it’s very modular and so on, at the end of the day, we’re still having to think about how do we ingest data? How do we conform the definitions of things, where customers from this feed customers from that one and so on, how do we do identity resolution and how do we structure this data in a way that is then suitable for analysis?
[00:23:32] Mark: So really one thing that came from that from writing it, is actually as much as this is all new and trendy and so on, there’s much that is still the same really. And I’d say that probably with work you do, again, years later, there’s still much the same really. The other thing on it is the profusion of components in there as well. So I suppose that the takeaway from that was how much things haven’t changed when they appear to be. It’s also thinking about another aspect to the modern data stack, which I haven’t really covered so far is I suppose there’s more emphasis now on the activation of data. So the danger with all analytics implementations is the ‘so what’ factor it’s that you analyse data and you go, well, what do I do with that really? And this is a bit of a, kind of a double-edged sword, really, because a lot of the activity in the ecosystem of the modern data stack recently, it’s been about how can we do this thing called reverse ETL?
[00:24:20] Mark: So taking a practical example, we do this ourselves. So we analyse and compute a whole bunch of segments, for example, for our customers and for our prospects where we looking at, how they use our website and looking at how they interact with content we publish, we can, from that deduce things like, what parts of what we do, could they be interested in, what is maybe their channel kind of preference, depending on the level of interaction they’ve had, for example, you know, how much of a promoter are they of what we do and all these kinds of things. Now that’s all well and good being in the warehouse, it’s sitting in BigQuery or whatever, but what if that was sitting in say, HubSpot or sitting in other systems we use or being used, so maybe find lookalike audiences in Facebook, for example. So in the past it would have been stuck in the warehouse or you’d have to use a tool, like say segment personas to try and build a CDP in there. But now can we take the data sitting in the warehouse and can we then through batch or whatever, feed that directly into HubSpot.
[00:25:12] Mark: Or can we use that to actually drive decision-making, automating decision-making in the business, which is great because it’s about getting value from the data and so on. The danger a little bit with that is it’s probably the scariest thing you possibly do because the data warehouse is not a system of record, and it’s certainly not governed and maintained and managed in the same way that say your financial system is. So it’s the idea of activating your data and using it to actually drive activity is interesting, but it’s really being done by people who don’t really understand, or don’t really have the responsibility to think about, well, what can happen if this goes wrong? We do that ourselves, but it’s one thing to pick the wrong Facebook audience but then you wouldn’t necessarily want to take more kind of concrete actions based on that. But that’s the other part of it, really, the idea of data activation.
[00:25:56] Daniel: Yeah, for sure the ‘so what’ factor is a really, really good way of putting it and I don’t know if we’ve got time today, but I’d love to chat to you a bit more Mark, especially around how, around that kind of, or non-governance of that, especially around things like consent and privacy and the difference between this kind of reverse ETL and a CDP and where those are going but maybe for another time we’ll bank that for episode two.
[00:26:16] Dara: Okay amazing. Thank you, Mark. This is the bit in the show, I think you’ve listened to our show. So you probably know what’s coming. You’ve maybe had a chance to think about this, but this is where we ask the really difficult question of what you’ve been doing outside of work lately to wind down. So what do you do when you’re not thinking about the modern data stack?
[00:26:31] Mark: So the true answer is because I spend most of my day managing the business and liaising with customers. What I do in my spare time is build out our warehouse and write articles on the modern data stack in some respects, when you’re running a business the one thing you don’t get to do is the thing that you find interesting. Other stuff I do is do a lot of cycling, so most weekends I’m out and the other thing I do, which is always fun, people were a bit surprised but I still do DJing because I used to be a DJ in Brighton years ago. So I still do that as well, I have my SoundCloud account and put mixes out there and that sort of thing. But yeah, other than that, generally, most of it is actually kind of still playing around with this technology because twenty-five years later, I still find this fascinating and I still, you know, more than anything I love tinkering with all this and building out new capabilities and writing about it.
[00:27:10] Dara: Brilliant what about you, Dan? What have you been doing lately to wind down?
[00:27:14] Daniel: I’m just definitely going to have to find the SoundCloud link and put that in the show notes as well, I’m really interested, maybe I’ll grab that from you later. So mine is a similar train Dara, so I want to talk about going to MeasureCamp on the weekend. So at the time of recording, it was a couple of days ago, but I know these come out slightly delayed, but on Saturday was MeasureCamp London, it was the 10th anniversary of MeasureCamp. And so it was an amazing experience to have like the whole decade of MeasureCamp being there, lots of people that we knew, lots of people I didn’t know, and I volunteered on the day as well to help out. So it was really nice to get involved in the behind the scenes things with the running of things. I didn’t do too much, I can’t take any credit other than giving people their badges at the front door and walking around. But yeah, it was such a lovely experience being back in real life with, you know, fellow analytics people. It was an amazing event in its own right, and it was the anniversary and it was the first one back, a lot of stars aligned and we had an amazing time and I know you were there Dara as well, but yeah, it was, it was an amazing time. We had a little crew of Measurelabbers there as well, so representing. So I just wanted to say it was great fun, I enjoyed it and I’m sure we’ll probably be talking about all the stuff we’ve been listening to and talking about there for some time to come. Anyway, what have you been up to Dara to get out of analytics?
[00:28:17] Dara: Mine feels a bit boring now by comparison, but I’ve been swimming. I’m not a great swimmer, so it’s not competitive swimming or even particularly high exercise swimming, but just started going to the local pool with my partner Hannah recently. Just do a few lengths as something totally different to unwind and relax, but yeah, I’m not going to be doing Olympic trials or anything like that, I think that ship has sailed.
[00:28:38] Daniel: So there’s me thinking that you’re running would make you a better swimmer, but it obviously doesn’t translate.
[00:28:42] Dara: Funnily enough no, I haven’t tried running underwater but maybe that’s what I should try next.
[00:28:46] Daniel: You’re missing a trick.
[00:28:47] Daniel: Yeah, exactly. Okay one more question for you, Mark, which is where can people find out a bit more about you?
[00:28:53] Mark: The website is rittmananalytics.com and we’ve got a blog on that, which has a lot of articles hopefully that would be relevant to this conversation, how we do these sort of things and techniques and so on. There’s also a medium site we have, which I think is markrittman.medium.com. The podcast that we host is called Drill to Detail so drilltodetail.com. Yeah and I’ll post my SoundCloud link on there as well afterwards. So hopefully that will get out and I’ll get loads of fans because of that.
[00:29:17] Dara: Brilliant, thank you. We’ll post links to all of those in our show notes. Dan, what about you? Where can people find out more about you or get in touch with you if they want to?
[00:29:26] Daniel: LinkedIn is the best way, LinkedIn, and my website danalytics.co.uk.
[00:29:30] Dara: And it’s LinkedIn for me. Okay that’s it from us for this week. As I mentioned at the top of the show, this is episode number 40. So we’re going to take a couple of weeks off after this one, but we’ll be back with you soon, and that gives you plenty of time to go back and re-listen to your favourite episodes or if you missed any, go back and listen to them for the first time, you can find all of our previous episodes in our archive at measurelab.co.uk/podcast. In the meantime, if you’d like to suggest a topic for Dan and I to discuss or better still, if you want to come on the show and discuss it with us, you can reach out to either or both of us on LinkedIn, or you can email us at podcast@measurelab.co.uk. Our theme music is from Confidential, we’ve got links to their Spotify and their Instagram in our show notes. I’ve been Dara, joined by Dan and Mark. So it’s a bye from me.
[00:30:18] Daniel: And bye from me.
[00:30:19] Mark: And bye from me, thank you.
[00:30:20] Dara: See you next time.
Daniel Perry-Reed
Subscribe to our newsletter:
Further reading
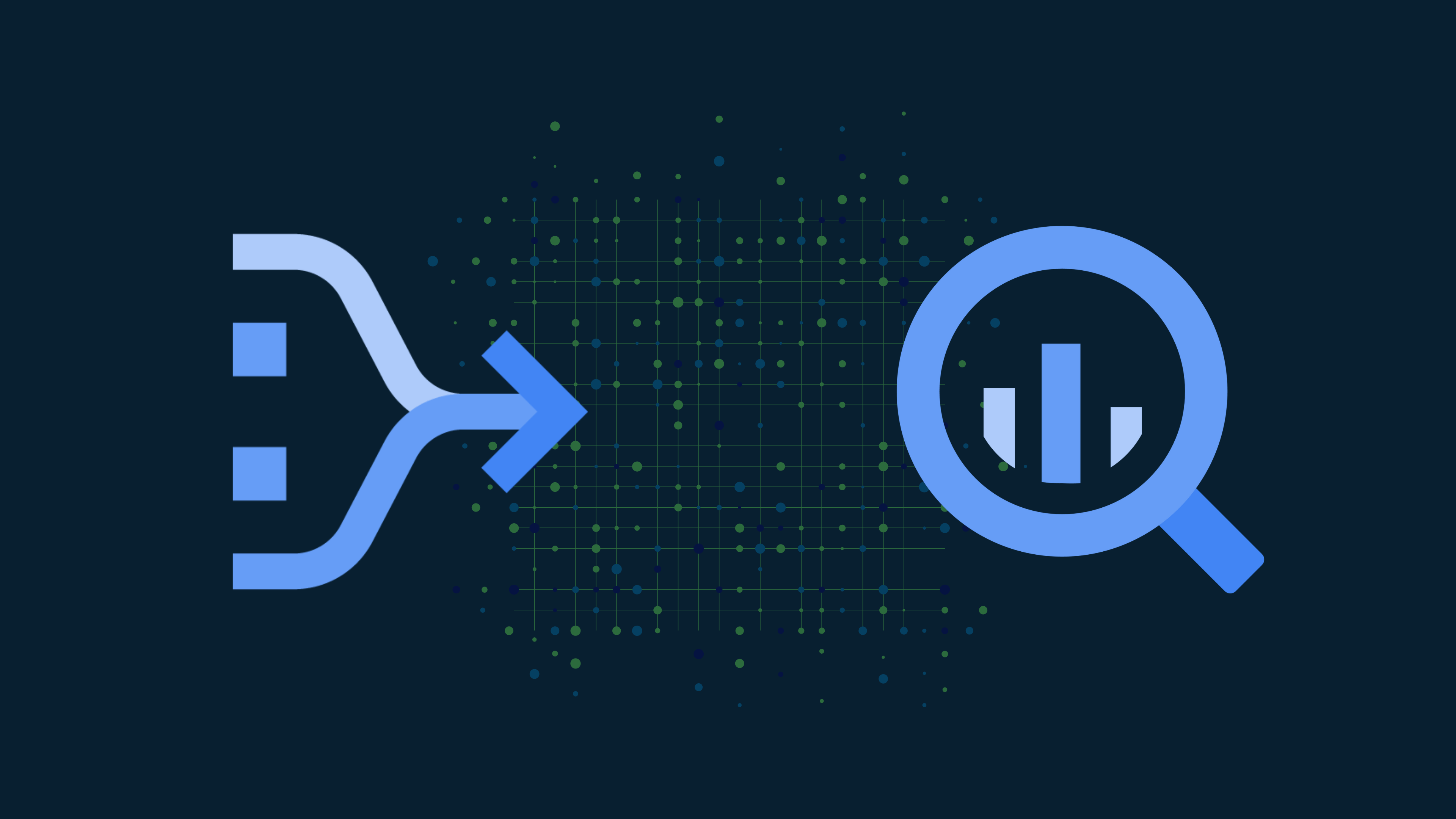
Dataform for BigQuery: A basic end-to-end guide
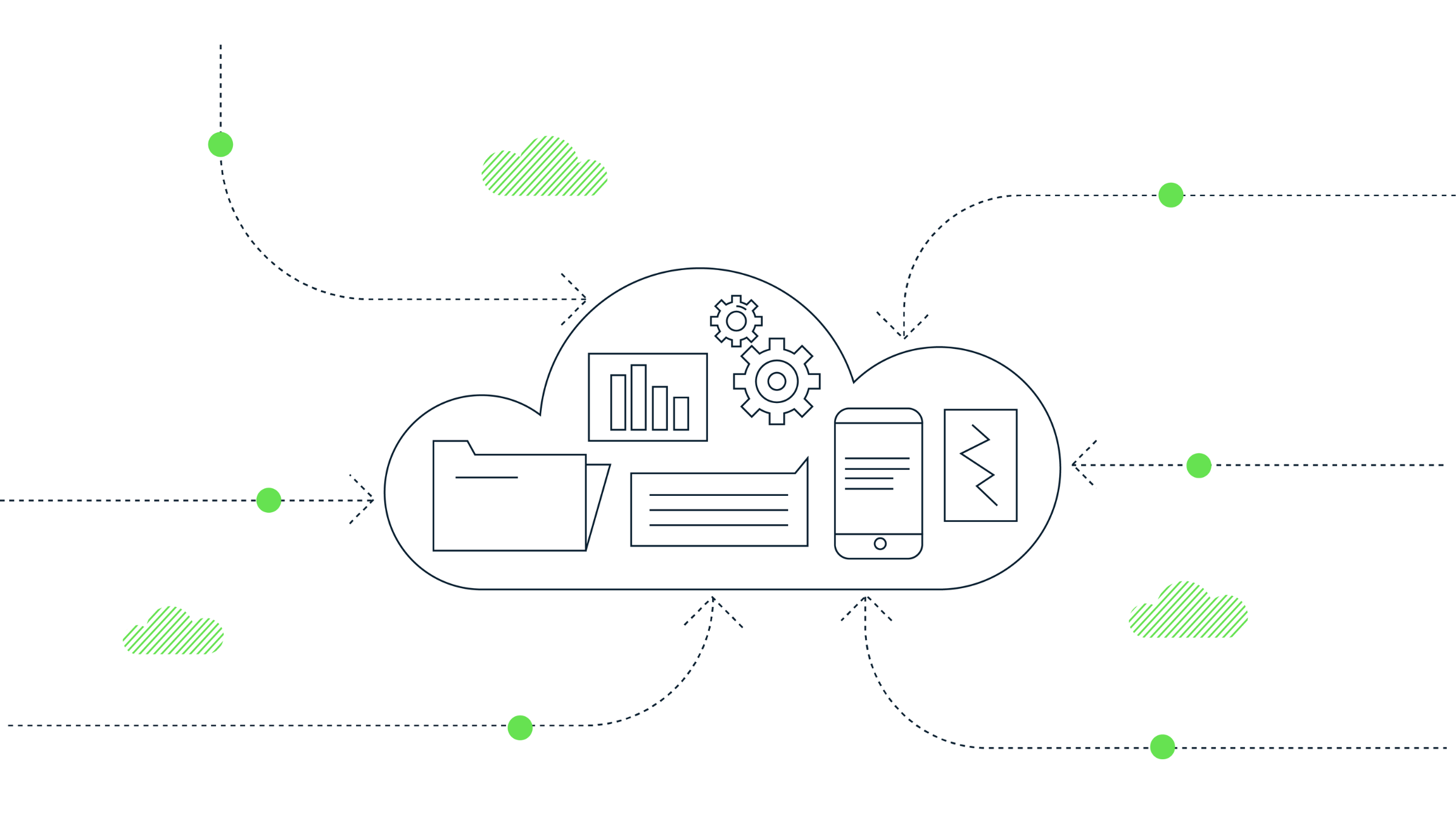
How to extract GA4’s event sequencing in BigQuery using the new batch fields
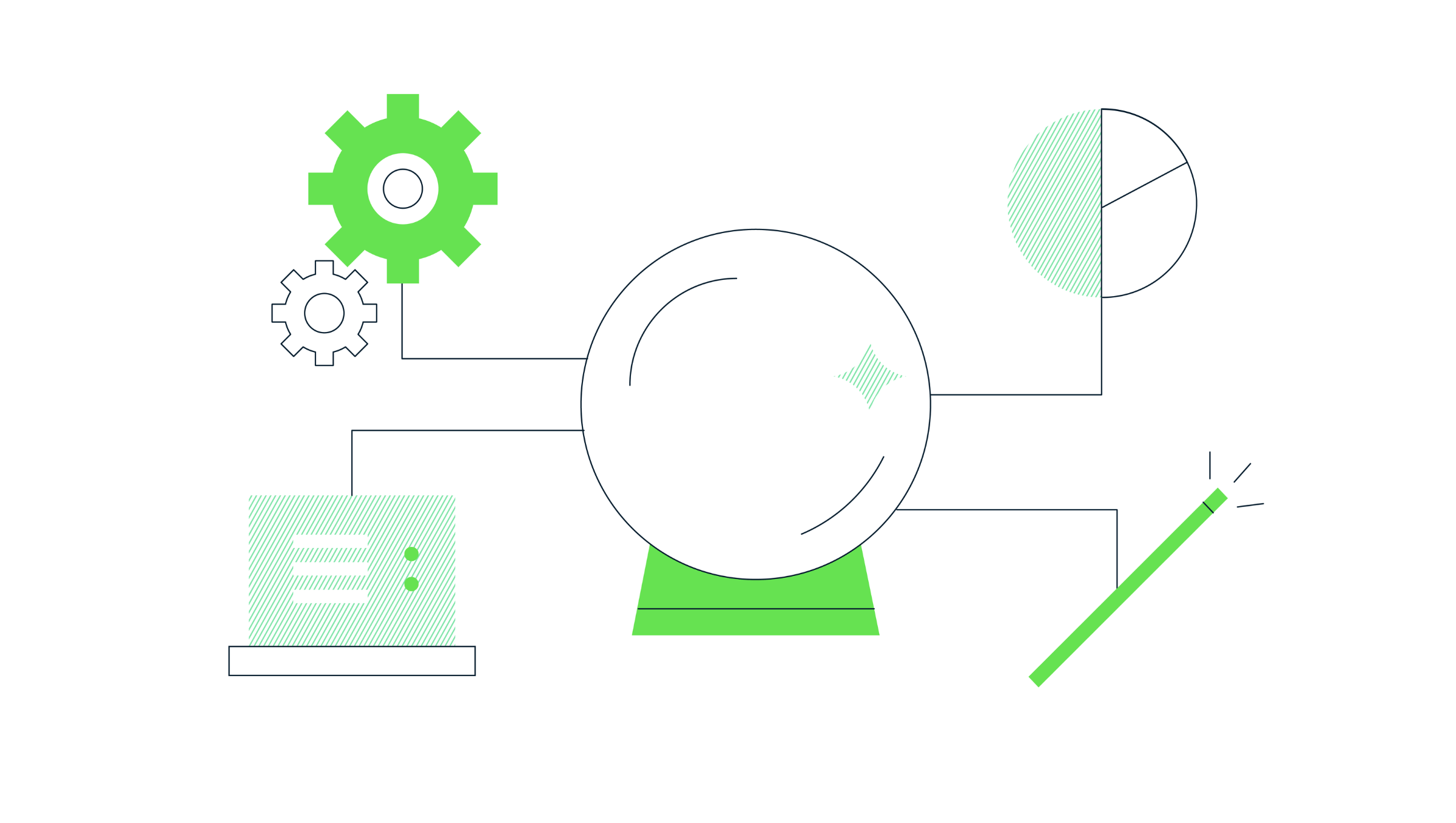