#90 Self-serve analytics: yay or nay
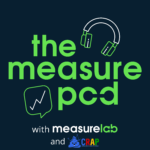
In this week’s episode of The Measure Pod, Bhav reflects on his biggest career failure: self-serve analytics. They discuss the challenges and complexities of implementing self-serve analytics in organisations, highlighting the factors that contribute to its success or failure. Bhav emphasises the importance of trust, data literacy, and problem-solving skills in enabling individuals to effectively use self-serve analytics tools. They also share personal experiences and strategies for promoting data democratisation and creating a culture of self-serve analytics.
Show note links:
- Please fill out our Feedback Form to let us know what you’d like us to talk about next season, or email podcast@measurelab.co.uk to drop Dan, Dara and Bhav a message directly.
Follow Measurelab on LinkedIn and on Twitter/X, and join the CRAP Talks Slack community.
Find out when the next CRAP Talks event is happening on LinkedIn.
Music composed by Confidential – check out their lo-fi beats on Spotify.
Master Google Analytics 4 with Daniel Perry-Reed on the next GA4 Immersion 6-week cohort training course. Early bird, charity and group discounts availible!
Quotes of the Episode:
- “…this topic and the whole concept of self serve has been one of the biggest failures of my entire career spanning like 10, 11, 12 years.” – Bhav
- “…self service doesn’t equal self sufficiency.” – Dara
- “…when marketers are always talking about like impressions and clicks as KPIs, I’m like, it means absolutely nothing. And like, you know, downloads of a podcast means nothing.” – Dan
Let us know what you think and fill out the Feedback Form, or email podcast@measurelab.co.uk to drop Dan, Dara and Bhav a message directly.
Transcript
The full transcript is below, or you can view it in a Google Doc.
Intro | Topic | Rapid fire
Intro
[00:00:15] Daniel: Welcome back to The Measure Pod. This is our final episode in the last run of 10 episodes we’ve got with Bhav Patel. Bhav, this is something you brought up, I think, even before we started recording the first episode and you said something that really stuck with us. And that’s why I thought it would be interesting to talk to you about, but you said that self-serve analytics is the biggest failure of your career. Now we’ve just come off the back, we’re recording this at the end and I can tell you you’ve got to listen through to the end to find out the answer to that. But a quick taster of that is that it’s probably not, but you had some interesting stuff to say about it.
[00:00:46] Daniel: I thought actually some of the examples you gave were really useful and actually it depends is kind of the answer, which is less satisfying than maybe we want it to be. But yeah, it was really interesting to hear you talk about it.
[00:00:56] Bhav: It depends is always going to be the answer to a question this big, right? Like this is not a small question. There are so many factors to take into consideration. Size of the organisation, the you know, like the maturity of the organisation, how much data they have, how good the data is. I think there’s so much to unpick there, but it’s something that’s been, you know, like something I’ve thought about for years. It’s a topic I’m super passionate about. I’m sure you guys are as well, because you obviously run the training sessions and Dara, you previously used to run the training session before you handed over to Dan. And so I think this is a topic that a lot of analytics professionals will probably think about.
[00:01:29] Bhav: It’s something that I, you know, hold myself accountable for. I think this isn’t something that, I would never pass as a responsibility to my stakeholders to go ahead and do. I take responsibility for it, it’s an OKR, an objective that I hold myself accountable for. And, you know, it becomes a very existential question and, you know, for me, it’s a lot about purpose and, you know, actually whether or not this is something we should be pursuing. And obviously you have to listen to the episode to go into a bit more depth about this. But it’s just something that I’ve just been super passionate about. And I don’t want to say my career has flourished and I’ve been successful in every turn I’ve failed in many ways. And for me, this has been what I consider the biggest failure which is why I just want to talk about it and I thought it might be a fun topic for our listeners who are mostly, I assume, analytics professionals.
[00:02:14] Dara: As much as I enjoyed this episode, I’m really looking forward to the one in the future where we bring you back on, Bhav, and you tell us about how you finally cracked it, where you’ve got the secret sauce.
[00:02:24] Bhav: I think we did like cover some of the ingredients for the secret sauce today, and that I think came out as we were discussing it, and actually it’s got me thinking about how I measure this. Again, I don’t want to give away too much. But obviously it’s, it’s, it’s a very interesting topic. It’s a lovely episode for me to end on being my last episode in all. So I’m glad we had the opportunity to discuss this in some great depth.
[00:02:46] Dara: I agree. I hope all of you listening enjoy the episode.
[00:02:48] Daniel: And let us know what you think. Just a bit of admin, there’s a link in the show notes to a form where you can tell us what you like, what you don’t like, and maybe some other people we should be speaking to. So please reach out and do that as you’ll hear through the rest of the episode, that’s how we mark the success of this kind of podcast. Otherwise, Bhav, it’s been a pleasure, we’ll save all the gushy stuff to the end of the episode if they listen through, but yeah, thanks again and enjoy the episode.
Topic
[00:03:09] Dara: Okay, I’m looking forward to this one guys, because you’ve promised me and I’ve promised the listeners that there’s going to be a bit of healthy debate at some point so this is my chance, I think, to stir this up between the two of you, because I have a feeling you might have at least slightly different views. And then I’m going to try and get my crowbar in and make those slightly different views, you know, a little bit more polarising. So the topic is self-serve analytics. Does it exist? Can it happen? Can you make it work? Who wants to kick things off?
[00:03:38] Bhav: I’m happy to kick this one off as I feel very strongly about this topic. I’m going to kick off by leading with the fact that actually this topic and the whole concept of self serve has been one of the biggest failures of my entire career spanning like 10, 11, 12 years. It’s a bold statement to make, and I probably shouldn’t say something like this so openly, especially if any future employers are listening and one of my objectives is going to be to help democratise and create an environment of like self serve analytics. But I’m going to be very transparent and it’s not for lack of trying, you know, we’ve tried. I mean, me and my team in the past, we’ve tried to at almost every organisation where I’ve played the role of head of analytics, the director of analytics, but I cannot make self-serve stick and that’s what I’m going to debate. So I’m going to be in the red corner, I’m going to be fighting the fact that maybe self-serve just cannot exist. And I’m looking forward to this discussion.
[00:04:32] Dara: You’re obviously just not doing it right.
[00:04:35] Bhav: Dara, I thought you were going to play like a neutral role in this.
[00:04:38] Daniel: No, he’s the pot stirrer. He’s just the perpetual pot stirrer.
[00:04:41] Dara: Yeah, exactly. Just getting my wooden spoon out and just getting, just starting to stir that pot.
[00:04:46] Daniel: I think this is going to be hard because I think I’m likely to agree with you, Bhav, but I think in just to kind of keep the conflict and for Dara to keep stirring, I think it’s slightly different from my perspective in terms of agreeing with you, but I’m going to disagree in the process. I think it’s doable and I think it’s possible, I just don’t think it’s achievable to the majority of businesses and teams and organisations. Like it’s a fundamental ground up thing that you need to be aware of and try for like, before you have the team, before you have the systems and before you have everything, because this is something that affects IT, it affects legal, it effects, you know, marketing teams, data teams, product teams.
[00:05:18] Daniel: So yeah, the reality is it’s unlikely and it’s not going to work. And the majority of times it’s a kind of fool’s errand. But I think it is possible.
[00:05:27] Dara: Well, can I just zoom us out for a second? Can we just define it first? Because, you know, I think different people might have slightly different interpretations of what exactly self-serve analytics means. Because you could obviously take it to different degrees or different levels. So why don’t we maybe start there and just kind of scope out a bit of a definition of what we, what we mean.
[00:05:45] Bhav: So I think for me when I talk about self-serve analytics, what I really mean is beyond just being able to access reports right. So you will have in most organisations, some form of a BI team or some, you know, someone who’s able to create and set up dashboards that people can use for the purpose of reporting, putting numbers in and out of decks, and just understanding the health of the business and keeping a finger on the pulse. For me, I don’t classify that as self serve. For me, that’s just reporting and creating a you know, an understanding of how the business is performing. Self serve for me is the ability to start peeling back the onion, and the layers of the onion and start asking the questions, why? And exploring those questions in the statement of a problem, it could be just some, something you want to look at or some understand a bit deeper, but there isn’t a clear cut way to do it, there’s not a dashboard that exists and requires some level of exploration. So that’s sort of kind of like my definition.
[00:06:38] Daniel: Yeah, for sure. I mean, I often refer to this as like data democratisation. It’s about providing like an equal access or an equal level of access to everyone that wants access and not ring fencing or gating that in any way. So it’s going to be things like, you know, I do a lot of training within the kind of Google Analytics space. And so when we’re training Google Analytics, it’s even that from that context, from that very narrow context, it’s like, should everyone in the organisation be given access to Google Analytics 4 to be able to go in and do the explorations, to do the reports. And then if they want to, they can build dashboards off the back of that data. Now, the reality is slightly different because I think that a lot of the time it’s data democratisation or self service is kind of like hidden behind. Oh, but I’ve given them a dashboard, you know, and I think that’s where I think that the debate comes in of like, at what point of access, whether it’s like raw unmodeled data versus an interface versus a reporting table you may have built off the back of your data warehouse that has had some cleaning, some modelling done to it and given them access there.
[00:07:36] Daniel: At what point do you say that you have democratised data, you have created a self-serve system or ecosystem, because I think that’s going to depend. And I think this is where for me, I think it’s, okay, there’s always going to be a level of, there’s always going to be a barrier between the end user and the raw data, because very few people in any organisation even wants access to raw data, even if they might say that what they’re actually meaning is I want access to a reporting table to build my own reports. And I think that for me, that’s where the data democratisation comes in. It’s like, where do we as an organisation draw the line? And I think that’s going to vary from company to company or even person to person really.
[00:08:11] Bhav: I’ve always tried to think about it as, because you’re absolutely right there. It basically comes down to ring fencing and accessing, and I think there’s also an element of like, width and depth that we have to think about in this, right? Like, for example, when I think about self-serve, I don’t mean giving people access to the raw you know, AWS tables or SQL tables, because I think that’s starting to now go into the roles of sort of like an analyst, right? For me, it’s maybe giving them access to Tableau or to Google Analytics or to Amplitude. So there is some easy to use interface, which allows people to access that data. And then there’s the depth of how far you’d, you know, like I consider someone to go. So when I consider self serve, at the very least you know, it’s just being able to play around in the interface, get in and out of the different types of analyses that you can do, whether it’s building a time series, whether it’s building a funnel, whether it’s just pulling together some dimensions and creating a report in, in Tableau or something like that.
[00:09:07] Bhav: So for me, it’s pretty much at its early stages, you know, I’m not expecting people to do like really deep cohort analysis or start building, you know, like regression models. It’s just, can they explore data? Can they find trends? Can they look back 30 days, 90 days, 180 days? Can they build some type of segment? Can they build some type of funnel? So it’s really sort of like some of those very simple concepts. Of course there’ll be people who then go further, but at a very entry level, that’s kind of what I mean is, as the barrier for self serve.
[00:09:36] Dara: Just to throw out, I’m sure we’re going to cover lots of reasons why it often doesn’t work or lots of problems that people encounter when they try and build a kind of self-serve analytics system. But the first one that comes to mind is people misusing it. So that’s often what happens, isn’t it? There’s an attempt to put it in place, someone goes in, draws the wrong conclusion or doesn’t understand what they’re supposed to be doing and mixes two things together that they shouldn’t or whatever. So how do we avoid that? How do we make sure that everybody who’s using the tools that are provided, they have enough of an understanding of what that data is, where it comes from, the context and they can actually make, you know, interpretations of it that are meaningful and make sense for the business.
[00:10:20] Daniel: This is the seesaw of going backwards and forwards between providing everyone access to raw data versus ring fencing it and providing a sheltered view or a manipulated view, because with great power comes great responsibility with great data access creates opportunities to fail basically. And I think the more access you have and the more availability of this data, the more likely you are to not understand every nuance or get something wrong or take a wrong number and run with that through, you know, optimizations or within your marketing campaign. So I think this is the argument of why we should not give everyone access to all the raw data. On the other side is you’ve got maybe very competent people that know all that kind of stuff and are getting frustrated about not having access to the data. But the thing is you have to create one rule almost for everyone, or at least at a team level.
[00:11:02] Daniel: So for me then if you are going to go down that path of, you know, giving access to that, to try and prevent that at some level, just has to come back to the less sexy things out there, which is documentation and training. It’s about providing resources for people to make as few mistakes as possible and to be able to have resources and documentation that’s evolved and adapted and added to when questions come up.
[00:11:24] Bhav: I think with all of this, there is an element of trust that’s required. So Dan’s absolutely right you know, there is some responsibility that analysts and data engineers need to take on to ensure the data is accurate. It’s, you know, whatever you’re giving stakeholders is reliable. But I think there’s also some level of trust that we as the, you know, the guardians, custodians of this data place on people who are requesting this data or we want them to use it. And it’s not really just about, of course, there’s accessing it, there’s manipulating it, there’s getting in and out of it and building those charts and pulling those reports together. But there’s also a sense of trust and, you know, we expect these people to be able to draw correct conclusions, right?
[00:12:05] Bhav: So let’s use experimentation as an example. If you’re going to give access to experiment data to your stakeholders, you know, you rely on them not to take that data and draw conclusions way too early, like within a couple of days when we haven’t gathered the sample size, or if someone’s doing a year of year analysis, we expect them to be comparing apples and apples and we expect them to understand the difference between percentage change and absolute percentage change, right?
[00:12:33] Bhav: So relative change and absolute change? So I think there is some sense of, you know, for me, self serve is not just about giving access. It’s also ensuring people know and have the tools and are armed with the right skill sets to be able to use that data and draw correct assumptions. So that’s for me, the two elements of self serve that I think about.
[00:12:51] Daniel: How do you police that then? So let’s say you’re working in an organisation and there’s a, there’s a team X and it’s five people and three of them have the desire and the want to go in, the other two don’t for example, but what happens then when you’re saying, okay, is it a person by person level, do you provide some kind of like, do you have to pass a test to kind of get access to that system so that they prove they’re not going to make those wrong assumptions? How do you do that? Especially at scale, how does that work?
[00:13:16] Bhav: So I’ve never got to the point where that’s the problem. The problem for me has always been once you’ve trained people to use the platform, to then get them to go back in and start using it on a regular basis. In Looker, you can do an analysis of your users. So you can go in and see who’s using Looker on a regular basis, how frequently they’re using it, who are the power users, who are the people who haven’t logged in for like 90 days. For me, the biggest challenge on self serve is getting people to go back in on a regular basis, look at the data. Then Dan, you know, you’re absolutely right, then how do you make sure? I think for me, it would have been, had I ever got to the point where people were using it on a regular basis, and maybe I never achieved this at scale, but on those, you know, you always get those one or two people who are like, yes you know, we love this we’re going to use every week and they’ll come back and just, you know, they’ll check with you, right?
[00:14:01] Bhav: Like, hey look, I found this. What do you think? Does this make sense? And I think if you can create an environment where there is some type of like feedback loop and it’s almost instantaneous or, you know, very low time in terms of assessing those self serve pieces of analysis that have been done, I think you can create an environment where, you know, the work that’s going out is somewhat quality assured and people aren’t making incorrect assumptions. But this is based on me having never successfully achieved this at scale.
[00:14:28] Daniel: So is this what you refer to as the biggest failure of your career? So tell us a bit about that. Like, why do you say those words? Why is this the biggest failure of your career?
[00:14:34] Bhav: Whenever I join a company or, you know, whenever I’ve inherited a team or whenever I built a team, I always set myself some OKRs, right? It’s a classic thing to do. You give yourself some objectives and I’m a firm believer of OKRs and I believe them in the sense that they shouldn’t be fluffy and they should be measurable. So I highly recommend a book called Radical Focus. If you want to learn about how to do OKRs properly, read Radical Focus. Anyway, I didn’t write it, so it’s a plug for someone else. But as part of that OKR process, I’ve always had, you know, like X, Y and Z OKRs and one of them has always been around democratising access to analytics and getting people to self-serve. And the way I’d always measure it is by number of weekly or monthly active users based on the platform we’re using. So again, it’s really easy to do this when you’ve got platforms like Looker or Amplitude, you can go in and make that assessment.
[00:15:22] Bhav: So for me, when I say this has been the biggest, you know, like failure of my career, it’s not that we fail to deliver the training or fail to provide those lunchtime learning hours, like a lunch and learn. Or those drop in sessions that, you know, these are classic ways to establish a self-serve culture, you know, we’ve done all those things. The failure is those monthly active users or weekly active users, however you want to track it, and those numbers are the ones that you want to see growing, you know, if there’s 200 people in your organisation and your monthly active user stays flat, like 25 and your team has 10 analysts, you know, you’ve only got like 15, 20, maybe 30 users on a weekly basis who are going in and actually using the platform so for me, that’s what I classify as failure.
[00:16:06] Dara: So I’m really interested in digging into that because I’ve experienced that before, where you give the people exactly what they ask for, and then for some reason they don’t use it. And there’s probably a whole bunch of reasons for that. Maybe it could be things like not having trust in the data or their own skills, maybe even, or if the tool is too slow, I’ve had that where, you know, someone says, look, it’s really painful I’d rather just wait for you to do it. Or if it keeps changing and they can’t keep track of what’s happening. Anyway, there’s probably lots and lots of reasons, but that in itself is really interesting because if somebody needs this data or they need to run this analysis, or they need to ask this question on a regular basis, why would they not then do it if you give them the means to?
[00:16:49] Bhav: I think the main reason for this is that when you run like training sessions, you have some very clear cut problems you’re using as examples, you do walk through of the platform and then you might solve like two or three problems and in those moments It’s kind of like aha yeah, I get this, this makes sense. But then you know, you send your students for lack of a better word out back out into the wild and they face their own problems and it’s whether or not those teams and the people who you’ve trained have the tenacity and willpower to go through the platform and try to reconnect what you’ve done, but apply it to their own problem. And I think potentially this is where the breakdown comes, because you’ve got a problem. As analysts this is, you know, this comes like second nature to us, this is breathing right for us.
[00:17:32] Bhav: We have a problem and we immediately translate it into an analytical solution of how we’re going to go about solving this problem. I’ve got a numerical background, I did maths at university, for other people it might be they’ve got a very scientific background or they’ve done it enough that it just starts to become second nature, and I think until people do it enough and struggle enough and overcome those internal problems, you know, turning those problems that they have into solutions is hard, it’s a big barrier to overcome for them. This is my belief, this is why self serve fails is that people lack the ability to take their conceptual, you know, their problems and conceptualise them into an analytics solution.
[00:18:10] Dara: It just made me think of another favourite topic of ours, which is data literacy. And is that, are we back to that again, where people don’t have the, it’s maybe not even that they don’t have the mindset, maybe they do, but they don’t feel equipped in some way, or they don’t have the confidence to do it. Because you’re right I think, you know, if you are delivering a training session, people have those aha moments. But then it’s like anything, you know, if you’re learning a language it makes sense at the time and then you stop speaking it and it all goes out of your head again, or, you know, regular expression or something like that, where unless you’re doing it every day, it doesn’t stick.
[00:18:41] Dara: It does make me think of data literacy again and whether people are just a mixture of scared and maybe I don’t like saying the word lazy, but whatever the polite version of that word is, maybe it’s just a case of thinking, oh, I’d have to go through and as you said Bhav, connect all those dots again, remember what it is, which report I need to go to. Or there was something I’m supposed to not do in that report, but I can’t remember what that was, or maybe I should just ask the analysts?
[00:19:06] Daniel: So my two cents on that is exactly that Dara, which is that it’s the problem solving and logical thinking, which is what you get through having a mathematics or a science background. I think anyone that’s come from a numerical kind of degree or education or, or kind of have a natural inclination to that kind of subject is because it’s like, I can think about something, I can break it into ifs and ands and ors, and I can think about a solution there. And the reality is that it’s not impossible for everyone, it’s like some people think there’s like, oh, I don’t like maths so I can’t do this or I can’t do that. And it’s actually because they just haven’t had a good teacher right. And often that’s the case we find with a lot of children is that, you know, they don’t like subjects because of the teacher. They find a teacher they like and then all of a sudden they have a natural gift for it. Or actually it’s just that most people are capable of it it’s just the way that it’s told to you.
[00:19:50] Daniel: So ignoring the teacher side, let’s assume, you know, we’re all getting the best education for Amplitude or Google Analytics or BigQuery, whatever it happens to be. I think actually it’s the initial breaking of the ice. So a lot of these people they’re sat in a room or they’ve watched a video and they’re like, okay, I now know that I can do this and how to do it, but it’s actually doing it, it’s getting that muscle memory, even as like the brain muscle memory of doing that. And for some people that may not have that logical thinking, problem solving attitude already built in from previous sort of experiences in life, you’re basically creating those pathways in the brain. And it’s actually just getting started is the hard part because some people are like, they know they could, and then they find excuses and actually doesn’t matter what they say it could be lazy, but it’s not, it’s probably, they don’t have time or they don’t see it as their job, or they feel like it would be better if someone else does it, or they need someone to check.
[00:20:37] Daniel: But actually, as soon as they do their first one, as soon as they log in and start playing around a lot. Ah, okay I get it. Or I know how to do it. And actually the second and third will just kind of, it will snowball down and I think it’s just that hard part. when I do my training sessions, and obviously this is focusing mostly around Google Analytics 4, but the same principles apply I explain it to a point whereby I say, look, your job while I’m doing this training with you is to not actually learn, but just to listen, absorb, and to think about ways of solutionizing stuff and thinking about processes. I said that your learning is going to happen after when you start putting it into practice and I say to them look I can’t force this obviously I’m not actually in their organisation I’m a third party.
[00:21:14] Daniel: But I said, my advice is to force yourself right now, make a promise to yourself to log into the platform and spend 10, 15 minutes with it every single morning with a coffee or a cup of tea. And just get that muscle memory, break that seal, you know, break that kind of barrier to get in and use it. It doesn’t have to be pretty, it doesn’t have to be something you show to other people, it’s just log in and do something. It doesn’t even matter if it’s relevant to your job. And I think if you do that for a week, and I tell them to potentially get back as a group together the other week after or a couple of weeks after, just to kind of reassess. The thing is you’re throwing spaghetti at the wall and it’s going to stick differently for everybody right. And I think collectively, if they know that they’re part of a whole, they’re going to feel a bit more confident to be like, I don’t have to learn everything, I can just do these bits. I’m really trying to encourage that throughout the whole process of just breaking that ice.
[00:21:55] Daniel: Because again, my perspective of this is not like, you know, here’s the content, here’s how you answer problems. For me, a success is someone asking me a question via email after the training course for me, that’s the success because, you know, some people aren’t going to ask just because they’ve naturally got it. That’s fine, but everyone’s going to have at least one question and the fact that they’ve reached out to me showing to me that they’re trying it, they’re putting into practice. And for me, that’s how I gauge success. It’s breaking that ice, is my KPI.
[00:22:21] Bhav: I have a question for you, Dan, you do this a fair bit, right? You have a lot of training sessions. And you’re constantly training clients and other agencies and, you know, what have you, right? Like, do you have like a number you aim for? Like a percentage that you aim for? And what is that around success of, you know, either people sending you that email or if you have access to some type of like who’s using the platform? Like, how do you ensure that the training you’ve delivered is landing and people are, you know, taking it on board and doing it frequently and regularly. And maybe they’re sending you an email, not right away, but within a few days or something like that.
[00:22:51] Daniel: So obviously once they’ve paid me, I don’t care anymore, that’s the main KPI. No, I’m just kidding. No, I don’t actually measure the response rate per course for example, I don’t actually measure that. That’s a really good thought though, so I should be actually measuring that. That’s a separate conversation, I’ll think about that separately, but in terms of the success, there’s two parts here. So the one which is harder when I’m brought in as a third party, as a trainer to do it, to have a training and then leave, because in a sense, I don’t then have access to that team and that group of people then afterwards, and I encourage the communication, but it’s very much towards me rather than backwards from me.
[00:23:23] Daniel: However, when I’m consulting. So working with companies and I’m running training, even if it’s, you know, again, Google Analytics or maybe we’ve built a suite of dashboards, an analytic suite, and we’re training them up on how to use the wider business to use that. Then actually it’s all about analytics again, it comes back to measurement. So we’re tracking the platform if we’re hosting it in like a Google site, so that’s able to be tracked. If we’re using Looker Studio, then we can implement analytics tracking on that as well. And it comes back to that and it purely comes back to, and a tracking of the platform, like you were saying before Bhav in terms of Amplitude and looking at the success rates of those weekly, daily, monthly active users.
[00:23:55] Daniel: And it comes back to the same core metrics of saying, are you using the thing that in a way you asked for? Right. And I know that we mentioned that earlier in the episode, but actually often, and this is the hardest thing, and this is the thing that you can’t, at least I’ve not found an easy way to teach. But when the hardest part of my consulting career is understanding that when people ask you for something, that’s not what they want and actually to figure out what it is they want and building a dashboard, I find that dashboard and building and reporting is often perceived to be an easy thing to do, and it’s actually one of the hardest things to do because you’re surfacing data to people that don’t know what to ask for, and it’s really impossible. But anyway, that’s how I measure the success, the same way as you.
[00:24:34] Bhav: I mean, I think if people make that first dashboard, even if they get it wrong, there is a very tangible output there for someone to say, hey look, you know, I’ve done this and then you build on it, right? Like I think when people start asking questions, like, for example, how is this metric doing by device or by marketing channel? Then you kind of think, oh okay, maybe I’ll just add that in as the next layer for someone to go a little bit deeper. So for me, that’s a great measure of success. If you’ve taught someone how to use a platform, and then they’ve gone and built a dashboard, that is the one of the best steps that I can think of, of taking that conceptual training that they’ve had and building something tangible about what they will probably use, because they’re building it with the metrics that they have in mind.
[00:25:09] Bhav: I want to touch on two things you’ve mentioned before, Dan, which was lack of time or they don’t feel like it’s their responsibility. Have I forced people into that training session? Or they’ve been nudged into those training sessions by their managers? And do they actually want to be there? It’s hard to say. I think for the people who want to be there and no one’s forced them to be there so this is something they consider as not, maybe not part of their job, but it’s certainly a way to enhance what they do. And that’s a great, you know, that’s a great starting point, but then you get hit with the time concept or the time element or the time factor, and that is that people who have a full time job doing something else, whether it’s marketing, product, you know, whatever it is, they’re going to naturally go back into doing that day to day job and actually, the next time they need to ask that question, it might be two weeks, it might be three weeks, it might be a month before they go back into it.
[00:25:55] Bhav: So this is something I haven’t tried. And actually, I’m just realising now it’s like such a stupid thing, I don’t know why I’ve never tried this before. At least not in a pre-training session. I do this with leadership teams when I join a company, so I create a centralised document and I asked them to ask, what are the big questions you want to know about the company right now? It becomes this living, breathing document that C suite contribute to, directors contribute to, heads of contribute to, but actually, if I did this beforehand, I said, hey look, before you come to this training session, make a list of 10 pieces of analysis you want to do or something you want to understand. Obviously we’re not going to be able to do it in this session, but hopefully by the time you’ve finished the training session, you can go away and try to attempt to answer those questions yourself because you’ve already got that okay, what is the first thing? And you can immediately try and get to it without waiting, you know, two weeks, three weeks, four weeks, a month, you know, whatever. So it takes that very fresh knowledge that you’ve just acquired and allows them to apply it in a very real situation. So maybe that’s how I solved my, you know, this biggest failure of my career.
[00:26:54] Daniel: Well, then you’ve got the next problem of getting people to contribute before the training. So that’s the next part, right? That’s always the thing that I found when I run the training sessions, it is always the case of like, provide me the questions you need answered, the kind of questions you might have around the platform and everything. And the reality is that most people don’t read the invite, let alone kind of contribute beforehand. And I think getting, maybe it’s a remote working thing nowadays, it’s slightly different when I do the onsite in person training, but when I’m doing these kinds of things, you know, there’s everything like the agenda, kind of what to bring, the kit to have, access to have, all of that stuff’s kind of like a prerequisite. I completely agree, maybe if I’m working in house somewhere or more into the team, rather than this outsider, because it’s a huge part of it, actually, that prevents me from doing all the stuff that I wish I could do, like that is just being this outsider.
[00:27:38] Bhav: I think it might be the outsider problem because I know that whenever I have run these training sessions in the past, I usually get people to like, I never force people to join the invite, I’ll send a blanket sort of like Slack message or invite or email something saying, hey all, I’m running this session, either put your name down for, you know, like this date, this date, this day, whatever. So you come along and so I think that is not the thing I’m worried about. It’s always going to be post-training.
[00:28:04] Daniel: What about you, Dara? Dara did all the training before I did. He kind of passed the baton over to me, I think as quickly as humanly possible actually, I remember joining and then day two was like, here you go, you’re doing training now. But you’ve run training, you’ve been doing this for a long time, consulting as well. Like, what do you think about this? Like, how do you get people to participate and to make sure that it’s sunk in and it’s been useful for them?
[00:28:23] Dara: I think you’ve got to accept that some people just won’t. And that’s frustrating because I think you automatically think it’s the most important thing because it’s what we do day in, day out. And you do always get those, you’ll get a handful of people in a training session. Well, actually, if you’re lucky, you might get a handful who ask you loads of really good questions and they’ve already spent some time looking at whatever platform it is that you’re training them on and, you know, they know what they don’t know, or they have an idea of it and you can kind of help them.
[00:28:49] Dara: Like you said, Bhav, if they’ve got that list of 10 questions or 10 pieces of analysis they want to be able to do, they might finish the session and know how to do five or six of them. And then they might even follow up with you afterwards and say, hey , I’m now looking into like number seven on my list can you help me? And then other people just won’t. And it’s, you know, whether, again, it is that they are scared to, or they’re just too busy and they don’t see it as high enough priority for them and they’d rather ask you. I think you do have to accept that you’re just not going to get everybody to buy into it.
[00:29:18] Dara: I wonder if like the expectations are sometimes really out of line where, so let’s say it’s us and we’re saying here, like here’s your shiny new self serve analytics solution that maybe we don’t always have a full understanding of what the users are actually going to want to use it for even if we’ve asked them. And equally, they don’t necessarily understand what they want because they don’t know until they don’t have it. And something I read, even just preparing for this today, something I read, which I thought was quite good, which is that self service doesn’t equal self sufficiency. And I wonder if what often goes wrong is you say, here you go, now you can go and help yourself and actually it’s not that simple is it you don’t just build it and then walk away. So maybe there needs to be a bit more focus spent on actually following up and checking okay, well if it’s not working, what’s going wrong? Right let’s refine it, what can’t you do? Okay well, that’s not going to be possible for this reason, but we can help you do that instead. And I wonder if a lot of the time it is just a case of, here’s your set of dashboards. Good luck, go on, fill your boots.
[00:30:17] Daniel: It’s such a big subject and such a hard subject to say in broad strokes for every organisation, because you know, if we’re talking about a startup let’s say less than 10 people within the organisation, getting access to Google Analytics and just logging in and having a look at a thing is absolutely fine. There’s almost no need for them to build dashboards, there’s no need for them at the moment, probably to have a data warehouse. It’s just log in, have a look at a predefined dashboard, walk away and that’s absolutely fine. So logging in and accessing the platform then is self-serve. I think then if you go up to the other extreme, you’ve got a company of hundreds of thousands of people, and then all of a sudden you’ve got multiple systems, huge volumes of data that won’t fit on a spreadsheet. You’ve got nuance in the data sets where you can’t just log in and look at total users or sessions anymore. And then all of a sudden you’ve got a data warehouse in the middle and you’ve got a data team and an IT department. I think then self-serve looks very different.
[00:31:05] Daniel: And I think self-serve then is probably, you know, there’s tools now that are coming out to address this, but dbt and Looker are these, these are kind of metrics layers, which are like a layer between engineering and analysis, right? So you’ve got the data engineering is kind of transformation, pulling data in, doing the kind of like the, the architecture, but then the analysis team don’t need access to the raw data they just need access to a enough of the data to do their modelling and their reporting of the back of the bit. So then, for example, rather than accessing raw data or even the UI, they need access to this other tool, which is like a layer, which has been set up between these two systems and I think this is where we might be struggling and I think everyone’s going to have a different opinion. And I think this is the hardest thing to judge, and it’s also something that changes over time. So data democracy, self serve, the definition of that will change as the company ebbs, flows, changes, and you get the different people in. I just wanted to throw that out there because it just came to my mind and actually is probably why we’re struggling to define it and quantify it I suppose is because of stuff like that too.
[00:31:59] Bhav: I mean, I’ve never looked at this as a, like when I think about my ability to, you know, build a self serve or democratise, data democratise culture, I try to confine it to my stakeholders. Now it might be that, you know, in some companies, my stakeholders were everyone in the company. In other companies, it was a subset of the, of people within the company. So I’ve always tried to be somewhat realistic because I have, you know, I did a short stint at you know, a big news publishing company and there was no way I was ever going to get self serve to work for, you know, there were like literally 10,000 employees, maybe, or, you know, or something like that, I was never going to get that to work.
[00:32:36] Bhav: But with my direct stakeholders and their teams, it was always sort of like the pursuit of that, you know, like getting these teams to self-serve. And that was always what I tried to do, because, you know, like in a, you know, if you join a big company, like let’s say you work for Coca Cola, you’re never going to get everyone in Coca Cola to be pulling reports and things like that.
[00:32:55] Dara: Trying to phrase this question, you’ve kind of teed this up as the biggest failure of your career. And I’m going to challenge that now, because I wonder if you actually have succeeded with it. So you gave a really good example there of the news publishing company where you said, look, there’s no way I’m going to be able to do it for 10,000 people. But that was never the expectation, but did you succeed in doing it for the core group of stakeholders?
[00:33:14] Bhav: I’m not convinced I ever did. And I think this, you know, it, it comes down to maybe there’s a, some existential question I need to ask here. It’s like, why am I pursuing this so badly? In your guy’s case, this is part of the service you offer as Measurelab, right? This is something you offer to your clients. It may be something you also offer as a one off package, but chances are it’s probably rolled into some other services that you offer, which is, you know, it could be implementation, whatever it might be, but it’s chances are it’s like a bulk package.
[00:33:41] Bhav: Now for me, having only ever worked client side for so long, you know, this is something that I’ve put on myself because it’s something I wholeheartedly believe in. I think if people can access and answer some of their own basic questions, that frees up my team to answer the bigger, the bigger questions. So maybe getting everyone to do it was never going to be successful. But you know, if I was able to make 10 product managers within a company become a bit more self-sufficient, that freed up you know, the analysts in my team to work on more better problems. And, you know, to some extent we, I have seen this in organisations where it’s like, okay, you know, my team were working on much bigger problems because there was just this absence of these really basic, hey, can you do this data pool for me? Or can you build this chart for me? Or can you build this dashboard for me? So actually, maybe I need to reassess my success criteria, not you know, this like bigger problem. And it’s really around the, is my team able to spend time doing meaningful work. And if they are, then I guess I don’t need to worry so much about the monthly active users or weekly active users on Looker or Amplitude or whatever. And maybe that’s how I need to reframe it.
[00:34:53] Bhav: So I need to go back and assess, because I do, you know, like, for example, my most recent stint at Hopin, I can say, you know, hand on my heart that my team spent a large portion of their time doing meaningful work. Same with Gousto, you know, my team weren’t spending their time doing like fluffy, like data pools and data like monkey type of work, they were working on more like very meaningful work that was contributing directly to the success of each of the pods and tribes that they were supporting.
[00:35:19] Dara: Turns out Bhav has actually successfully implemented self serve analytics so.
[00:35:29] Bhav: I genuinely have always considered this as a failure of my career. You know, regardless of my most recent, you know, like now redefinition of what success looks like, I’m still not convinced I’ve cracked the nut. And that’s maybe because of, you know, people don’t feel responsible that it’s their job, maybe they shouldn’t have to do this. Maybe it’s why am I pursuing this so badly? Maybe it’s just people don’t have time and, you know, like we’re always pulled in a million different directions so I don’t know.
[00:35:56] Dara: I’m going to be the annoying one and bring AI into this, somebody had to, it’s going to be me. So how is that going to change, change this? Because if we get to a point where we’ve got good LMMs built into analytics tools and users who aren’t maybe as data literate as maybe we might want them to be, can ask a series of questions that gradually get them to where they want to be. Is that going to be the answer to this? Like it’s the answer to everything and also the biggest problem, you know, it’s the problem and the solution to everything.
[00:36:27] Daniel: Well, it’s an answer. And I suppose that it’s going to be a pretty big part of the future of self serve. We all got so used to self serving via things like search engines and I think a lot of us are now getting quite used to the kind of ChatGPT kind of self-serve nature and understand the limitations. I think, you know, it’s an inevitability that there’s going to be some kind of text based chat interface to data. I think the hard part there is to, in exactly the same way that you can pull wrong data from an interface like Amplitude or Google Analytics, you can ask for the wrong data and I think trusting and relying and tying up these loose ends within the LMM is going to be the key part here, because if you just say, how well have I done yesterday? Like, what the hell does that mean? No AI is going to learn what that means because that’s going to be different to everyone.
[00:37:12] Daniel: Whereas I think if you say, okay, give me sessions for the last three months for organic search. And then all of a sudden, then you need to be like, you need to have a prompt be like, okay, well what, you know attribution model are we using? And what do you define as organic search? Do you just want to look at Google? And I think all of this stuff’s going to have to come out, but I think it’s going to take kind of what that was saying earlier is going to take that kind of like, that really fluffy layer away from the analysts and the analytics guys. And I think this is the key thing. It’s going to be able to self-serve the kind of volume of what can be self-served just out of the box is going to be increased, it’s going to start eating into the overall workload, which then frees up the other time. And I think if you’re unaware of the complexity behind this. So a lot of people that aren’t in the data analytics space might feel like, oh, it’s going to take jobs, it’s going to take the analyst jobs. Do we need to hire an analyst if we’ve got a chat box?
[00:37:56] Daniel: But actually it’s quite the opposite, it’s going to free up the time to actually do more meaningful work. And actually, if anything, it’s going to make them more productive and more meaningful to the business because it takes away some of the, you know, the reality of it, some of the bullshit requests that sometimes we get asked to do, which aren’t in any way meaningful right?
[00:38:11] Bhav: I mean, just to add to that, Dan’s absolutely right. Like I think if we do get to a state where people are able to ask these, you know, these chatbots and LLM models good questions, well, good prompts, give them good prompts to what they’re asking them. I think that frees up a lot of time. The question is going to be, and I think we touched on this on a recent podcast with Barry, is how do you get people to ask better questions? And I think that’s the tough, that’s going to be a challenge. So actually whilst, you know, AI or machine learning, or whatever, it will solve one problem, it will probably just shine a light on a brand new set of problems, which is how do you get people to ask the questions that really matter, you know, to really get under the hood of what’s going on, like, you know, like Dan mentioned that, you know, how many sessions did we see from organic search, that’s a good question, but then you go underneath that and then you want to ask is it converting? Does organic search do better? Has it done better than paid search?
[00:39:02] Bhav: If there’s been a rise in organic search, has it been through terms that aren’t converting as well? So, you know, even though we’ve seen a growth in that. Why is conversion going down? So, you know, these types of like more meaningful questions or suddenly, you know, especially in the product space, product doesn’t have a black and white, or, you know, like a predefined set of questions that you can lift and shift into any organisation that you, you know, you can do to some extent with marketing. Product questions, you know, when you start to understand what drives user behaviour and how a company makes revenue through product and product development, you know, the questions that have to be really bespoke, they have to be really targeted and you really do have to understand your product, then ask the right questions. And I think that then becomes a new challenge is how do you get people to ask the right questions? Because, you know, we’ve touched on this so many times, people just ask the wrong questions.
[00:39:48] Daniel: Well, this is a, there’s a tiny little feature in Google Analytics 4 for it sorry to bring it back to that, but that’s where my happy place and my expertise is. But like, there’s a big search bar at the top of GA4 and by the way, this is pre kind of like GPT blow up stuff. And it’s a search bar where you can ask it simple questions and you can get it some, you get simple answers. And I think this is something that I find a lot of people go, ah, that’s really cool because a lot of people don’t really want to log into GA4, they just want a number. And they haven’t got to the point of building dashboards or having a data person to be able to ask.
[00:40:16] Daniel: And it’s just like, I just need a number and that’s really useful, but that’s not the interesting part. I can imagine that expands over time with things like ChatGPT and Bard they’re developing, of course it will. But what’s really interesting is actually it provides a list of example questions and it says like, okay, do you want to look at acquisition? Do you want to look at kind of geography? And then you open that up and it’s got a predefined list of questions. And I think maybe that’s the way to go with this kind of world and maybe that can be generated to like, it can learn from what everyone else is asking in the organisation, but start with a list of curated questions that can be defined by the analyst, for example.
[00:40:47] Daniel: But it’s kind of like, do you want to look at performance? Okay. Here’s a set of questions you might want to start with and then elaborate from, but like it’s not in terms of like governance, you’re governing in a way you’re directing formed questions for them to ask, but they can still have access beyond that and continue that kind of conversation with the LLM. I don’t know I just think it’s something that’s quite interesting. I just, it made me think of that actually when we were talking about it. And I find that actually really useful because again, it goes back to that point is that people aren’t stupid, but people don’t know what they don’t know and they don’t know what to ask for. They don’t know how to ask for something because often they say, oh, give me sessions by day. Or like that example we just had Bhav about organic search sessions, like that’s not what they want to know. What they want to know is, is it working, but that’s so ambiguous they need to start somewhere and actually sessions from organic searches, where they start that’s not what they need, right?
[00:41:36] Dara: But it’s getting there already. So some of the, with the ChatGPT4 stuff, you can if you throw in a bunch of data, you can say, have a look as if it’s a person, I’m anthropomorphizing now, but like, have a look at this data Mr. AI and tell me what kind of questions I could ask to help me understand performance or help me understand if this is good or bad, and it will come back with a bunch of questions that you could ask. So it’s already getting there where, you know, if it’s got access to the right data, you could say, I’m trying to understand, you could start with literally the most basic question of I’m trying to understand if this is doing well or not and then it you know, what should I ask you? And it will come back and give you those suggestions. So I think you’re right, because often the problem is people don’t know the right question, but they do know what they want. And if they can kind of continue to refine those questions until they get to the real question.
[00:42:25] Bhav: For anyone that’s listening, I truly, truly believe this is a skill that can be learned. Like this isn’t a, you either have it or you don’t. Let’s use this podcast for example, I actually run this exercise when I’m giving a very specific talk at a conference. I have this one talk about systems thinking and building a systems model and looking at your product as a system. The exercise and thought exercise I like to do in the spaces of conference is to understand what makes the conference successful and we can do it with this podcast, right?
[00:42:50] Bhav: Imagine if you’re a podcast, you have your own podcast, chances are most people probably just saying how many people have logged in and listened to the podcast, right? That’s a good question, but it’s not a meaningful question. I think the way I would think about it is, okay, where are people dropping off? Are the podcasts too long? Are they too short? For example, if you’ve got a hundred percent listener rate right to the end, then, you know, maybe you could expand it a little bit and give people something, maybe they want some more, you know, for you to go in a bit more depth.
[00:43:15] Bhav: It could be, are there topics you want to analyse? Like actually, are some topics getting more listens than others? Are there particular guests that are coming on which are attracting more you know, more people to listen to it? It’s a really dumb game, but I like to do a whole bunch of thought exercises around products and like companies, like what are the types of questions that I think are meaningful? And I think if people can do that about their own company, it could be, you know, it could be anything, just make up a scenario and what are the kind of like the core success metrics? Now, in the case of a podcast, we want it to be retention. So of course we want thousands and thousands of users, but we also want those users to come back on a weekly basis.
[00:43:49] Bhav: And then it’s like, okay, well what drives that? Okay, is it the topic? Is it the length? Is it the guests? Is it the, you know, the nature of the conversations? Is it too technical, it’s too philosophical, whatever that might be. That’s how I approach these type of problems. Because I know we’ve been talking about this quite conceptually, and I just wanted to bring a really applicable flavour or layer to it so that people can think about, how do I get to ask better questions and that’s kind of like my mental exercise.
[00:44:14] Daniel: I have been trying to click about and trying to get some stats for this podcast, trying to see some of those things that while we’re on the live on the podcast, but actually I can’t, and I’m struggling to find it. So I’m going to give up on that, but I know for example, exactly right Bhav in terms of those metrics for stuff like this, it’s not just listens, this is one of my favourite conversations to have but it’s like you know, when marketers are always talking about like impressions and clicks as KPIs, I’m like, it means absolutely nothing. And like, you know, downloads of a podcast means nothing, but you know, do they contact us by the way, listeners, we have a form to get in touch with us and to ask us questions or suggest guests and suggest topics and you know who you are if you’re listening, but we’ve had a bunch of people get in touch that way. And I think that’s the kind of real meaningful stuff, right. It’s about it being meaningful, and I was trying to log into the Apple podcast to see like listen through rates and the percentages as well, just to see that kind of drop off. But you know, again, sorry, going back to the point I agree.
[00:45:03] Dara: Okay so through the course of this conversation, have either of you changed your point of view? So Bhav, do you now think, now that we’ve encouraged you to see your own success more clearly, have you changed your mind? Do you think it’s possible to do self serve analytics?
[00:45:18] Bhav: I don’t know. I don’t think I’ve changed my mind. I still think like, I’m definitely going to reassess it. And I’m going to definitely ask the question, why am I pursuing this? Because I think it’s a case of it might be the right, you know, the company I’m working for might be the right company to pursue it. In other companies, it may not be the right, the right thing to pursue. So I think it becomes a very situational circumstantial question that I need to ask myself. And then once I’ve done that, I need to potentially rephrase and reframe my success metrics and my success criteria of what self serve success looks like. So I don’t think I’ve changed my mind, I’m 38 years old, ask me again on my deathbed.
[00:45:58] Dara: That sounded a lot like a maybe to me. You’re on the way in the other direction, you’re on the path, I think. But I will, I’ll ask you in the future and see if you’ve changed your mind.
[00:46:10] Bhav: I will end on one final thing, whether it’s something I think is successful or not, or can be successful or not, is irrelevant. What I still firmly believe in is the pursuit of it. And I think the pursuit of self-serve data democratisation and empowering people to ask and answer their own questions and ask better questions is a worthy cause. So whether I’m successful on it or not is irrelevant, I will probably continue to pursue this for the rest of my career.
[00:46:38] Dara: Dan, have you changed your view?
[00:46:40] Daniel: Well, I’m just going to agree with Bhav, but it’s the journey, not the destination, right? And as Bhav was saying it, I was kind of agreeing and thinking it myself, but like, I think actually changing my definition of success of a kind of journey like that is the key thing. So like removing everyone being able to self serve, but it’s actually out of everyone that would have done it, have they done it? And I think that’s the key thing. And like I was saying earlier in the episode, it’s like actually what self serve means is going to be different for everyone. So there’s not some holy grail of like everyone knows SQL and goes into the database and pulls this data. Actually self serve is relative to the tools and the organisation and the kind of things that you’re doing right now. So I think for me, I’ve changed my mind to a point of being kind of yay or nay with the self-serve analytics. And I think actually, for sure, it’s something we should all be trying for. I think it’s only ever a good thing, but actually that destination is different and success is variable. And so again, it’s the destination, not the journey, but yeah, for sure. I think, yay, self-serve analytics.
[00:47:36] Dara: Well I’ve failed in my one mission, we’re 10 podcasts down now, and I haven’t managed, if anything, I’ve got you two to agree even more than you already did, rather than to get you to come up with some healthy debate, but it’s been great. And it’s been great for the last 9 podcasts with you, Bhav, and this one’s been a really nice one to wrap it up. So thank you for agreeing to come on and join us and be guest host for these 10 episodes. Hope you’ve enjoyed it as much as we have.
[00:48:01] Bhav: No, I have. I’m getting all emotional now. It’s actually been, it’s been fantastic. I’ve loved meeting all the different guests that we’ve had. I’ve loved the conversations that the three of us have had. I hope, you know, we continue them it doesn’t have to be in podcast format, but certainly in different platforms and arenas and, you know, events like that. Obviously, Dan you’ve been volunteering and hope you continue to volunteer at CRAP Talks for the, you know, for the foreseeable future. So no, I’ve loved it thank you so much for inviting me it’s been an absolute pleasure. I hope people who have listened to these podcasts have found it valuable, I hope I have brought some value to the podcast and to all the listeners. So thank you I’m very, very grateful and very humbled to be here.
[00:48:35] Daniel: Thank you so much, Bhav it’s been a pleasure. And to everyone listening, we’ll be back in a couple of weeks, hopefully fingers crossed with more, more analytics chit chat.
Outro
[00:48:44] Dara: That’s it for this week to hear more from me and Dan on GA4 and other analytics related topics all our previous episodes are available in our archive at measurelab.co.uk/podcast. Or you can simply use whatever app you’re using right now to listen to this, to go back and listen to previous episodes.
[00:49:03] Daniel: And if you want to suggest a topic for something me and Dara should be talking about, or if you want to suggest a guest who we should be talking to, there’s a Google form in the show notes that you can fill out and leave us a note, or alternatively, you can just email us at podcast@measurelab.co.uk to get in touch with us both directly.
[00:49:19] Dara: Our theme is from Confidential, you can find a link to their music in the show notes. So on behalf of Dan and I, thanks for listening, see you next time.
Daniel Perry-Reed
Subscribe to our newsletter:
Further reading
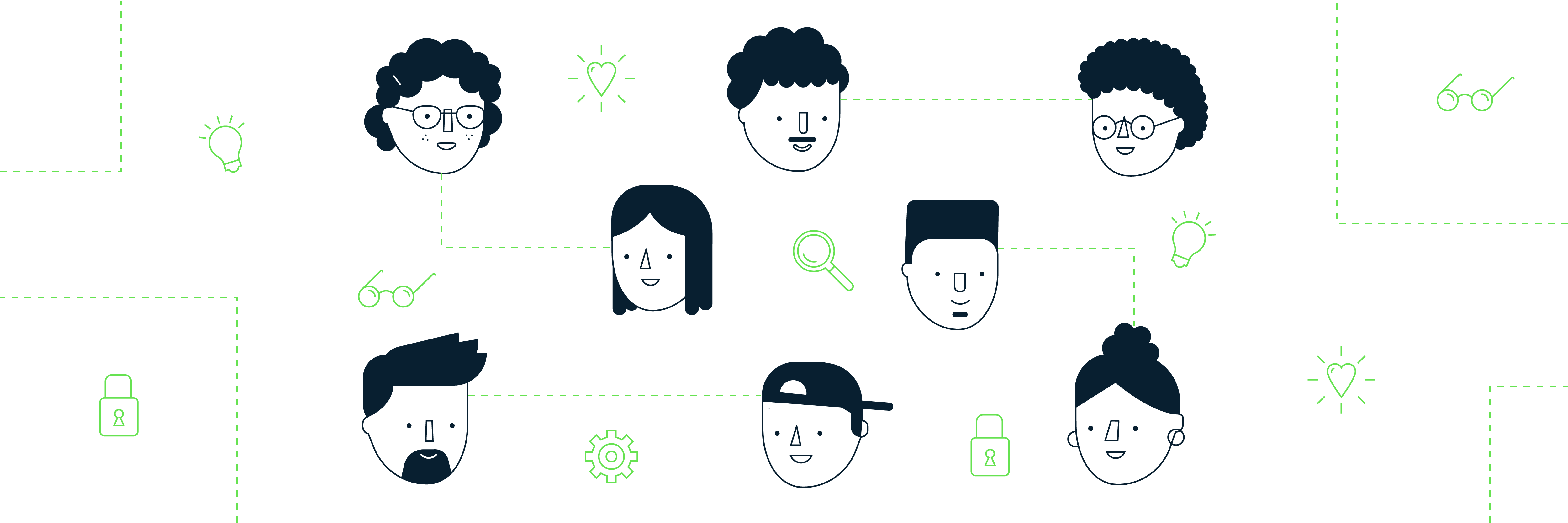
Firing up some remote feedback with My Three Things
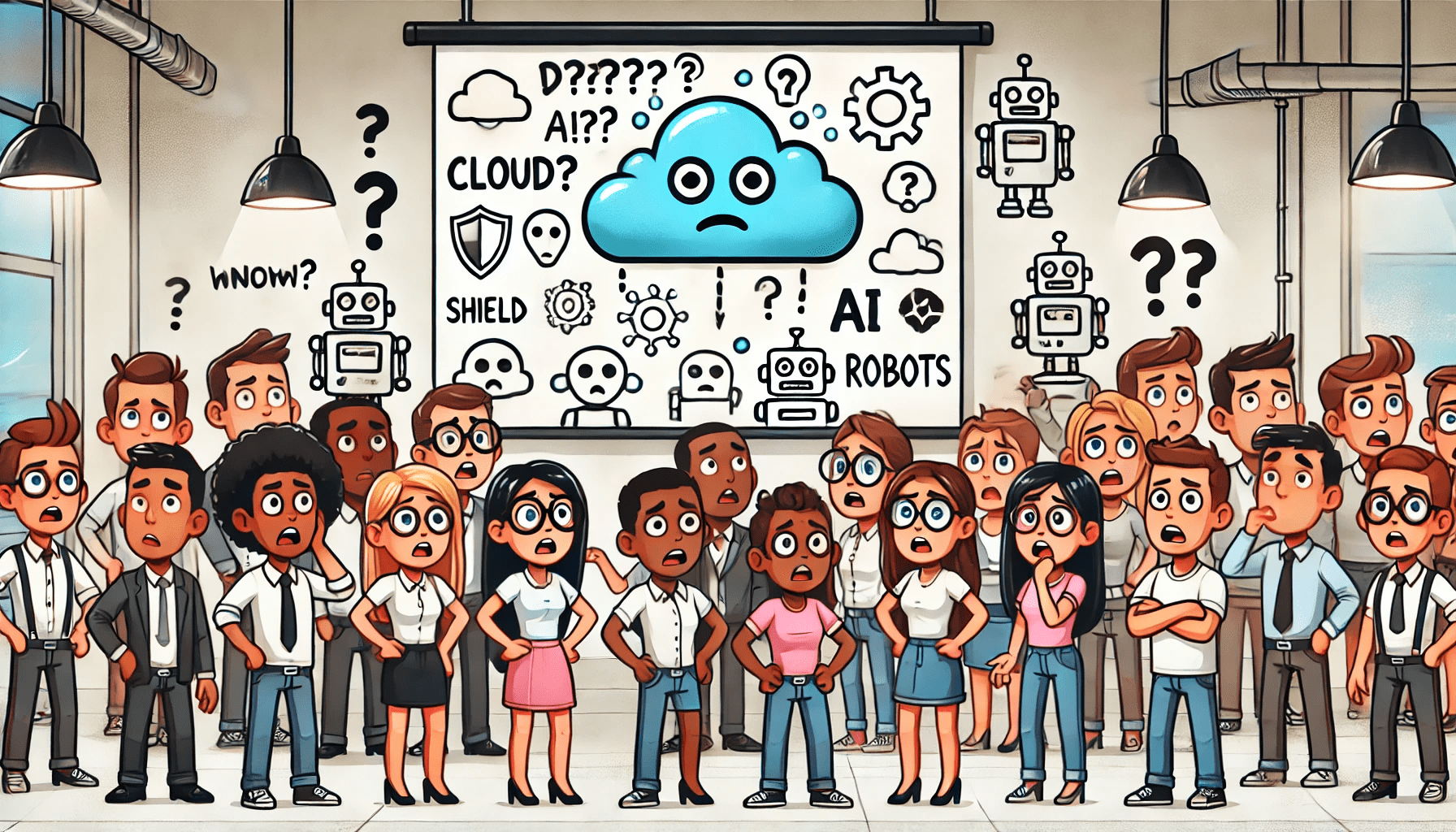
The modernisation of marketing and the role of marketing analytics
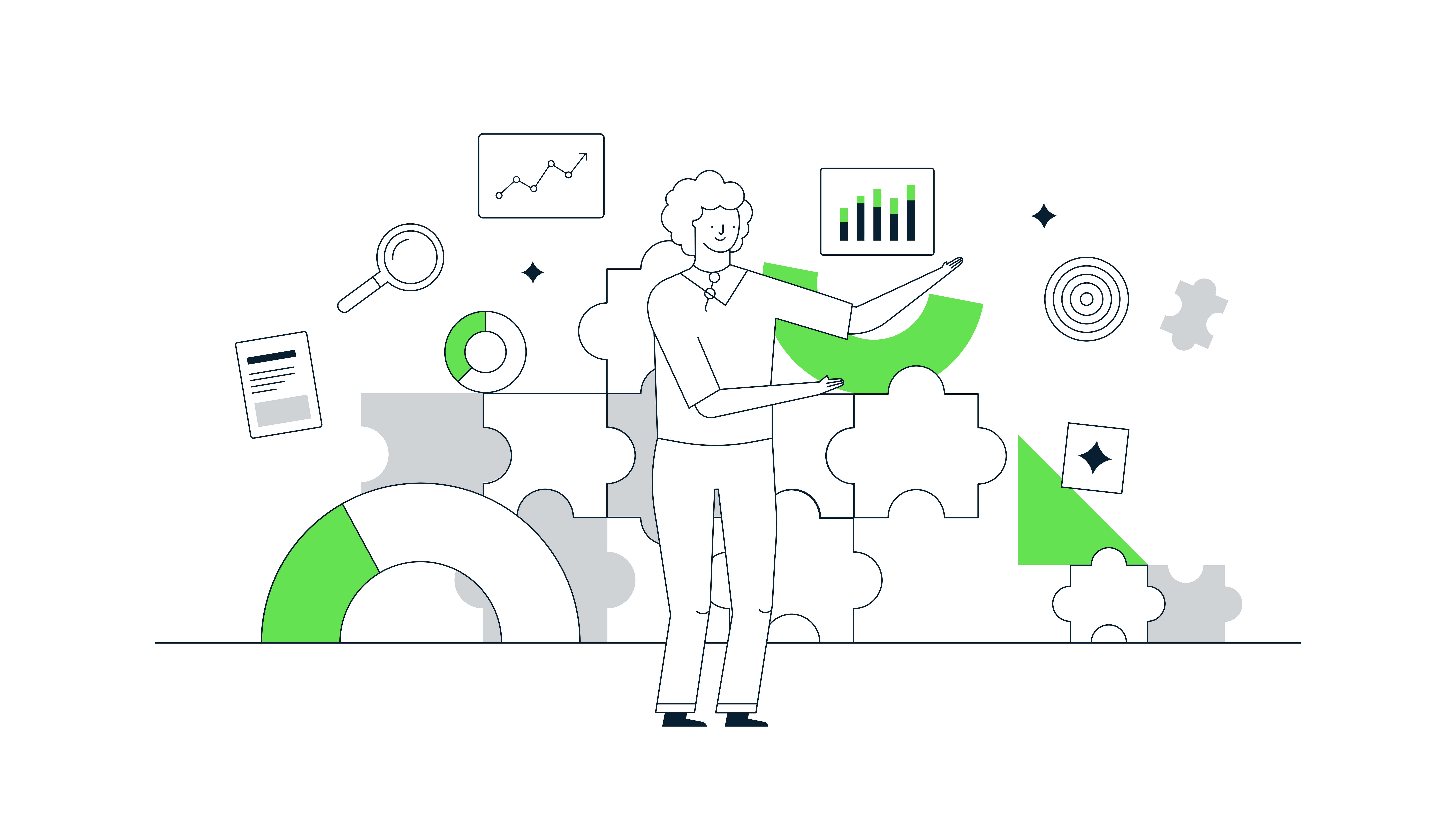